Deformity removal from handwritten text documents using variable cycle GAN
International Journal on Document Analysis and Recognition (IJDAR)(2024)
摘要
Text recognition systems typically work well for printed documents but struggle with handwritten documents due to different writing styles, background complexities, added noise of image acquisition methods, and deformed text images such as strike-offs and underlines. These deformities change the structural information, making it difficult to restore the deformed images while maintaining the structural information and preserving the semantic dependencies of the local pixels. Current adversarial networks are unable to preserve the structural and semantic dependencies as they focus on individual pixel-to-pixel variation and encourage non-meaningful aspects of the images. To address this, we propose a Variable Cycle Generative Adversarial Network (VCGAN) that considers the perceptual quality of the images. By using a variable Content Loss (Top-k Variable Loss ( TV_k ) ), VCGAN preserves the inter-dependence of spatially close pixels while removing the strike-off strokes. The similarity of the images is computed with TV_k considering the intensity variations that do not interfere with the semantic structures of the image. Our results show that VCGAN can remove most deformities with an elevated F1 score of 97.40 % and outperforms current state-of-the-art algorithms with a character error rate of 7.64 % and word accuracy of 81.53 % when tested on the handwritten text recognition system
更多查看译文
关键词
Handwritten text,Strike-off,Semantics,Generative adversarial network,Image-to-image translation
AI 理解论文
溯源树
样例
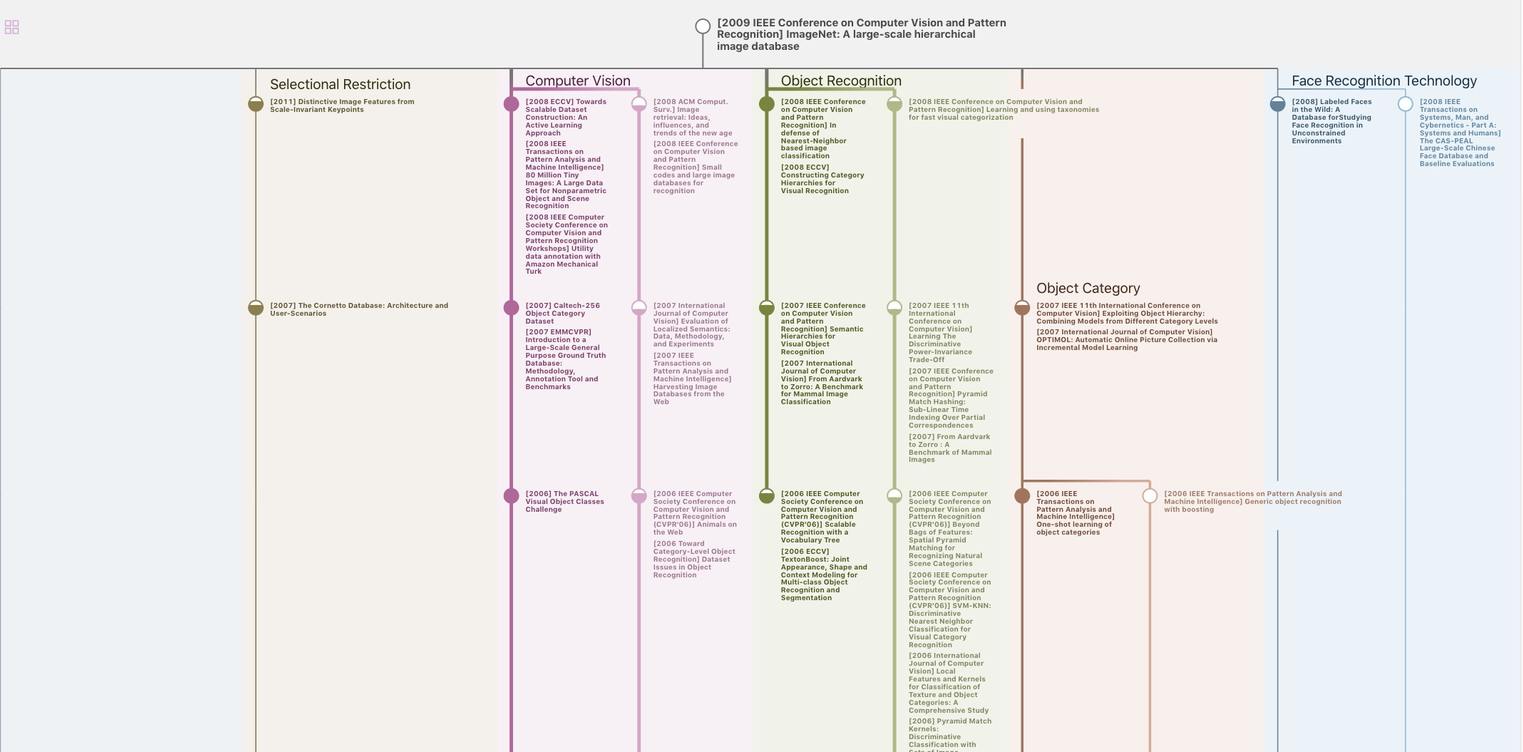
生成溯源树,研究论文发展脉络
Chat Paper
正在生成论文摘要