A predictive model of macrosomic birth based upon real-world clinical data from pregnant women
Research Square (Research Square)(2022)
摘要
Abstract Aim: To develop a nomogram model for the prediction of macrosomia using real-world clinical data.Methods: In the present study, we retrospectively analyzed the medical records of pregnant women who delivered singleton infants at a tertiary hospital in Shanghai from 1 January 2018 through 31 December 2019. We extracted the data from a total of 13,403 pregnant women for this study, with the original dataset split into a training set (n = 9,382) and a validation set (n = 4,021) at a 7:3 ratio to generate and validate our model. The independent risk factors for macrosomia in pregnant women were analyzed employing multivariate logistic regression, and the nomogram model used to predict the risk of macrosomia in pregnant women was established and verified with R software.Results: We compared the differences between the macrosomic and non-macrosomic groups within the training set and found 16 independent risk factors for macrosomia (P < 0.05), including biparietal diameter (BPD), head circumference (HC), femur length (FL), amniotic fluid index (AFI) at the last prenatal examination, pre-pregnancy body mass index (BMI), and triglycerides (TG). Values for the areas under the curve (AUC) for the nomogram model were 0.917 (95% CI, 0.908-0.927) and 0.910 (95% CI, 0.894-0.927) in the training set and validation set, respectively. The internal and external validation of the nomogram demonstrated favorable calibration as well as discriminatory capability of the model.Conclusions: Our model has precise discrimination and calibration capabilities, which can help clinical healthcare staff accurately predict macrosomia in pregnant women.
更多查看译文
关键词
macrosomic birth,pregnant women,predictive model,clinical data,real-world
AI 理解论文
溯源树
样例
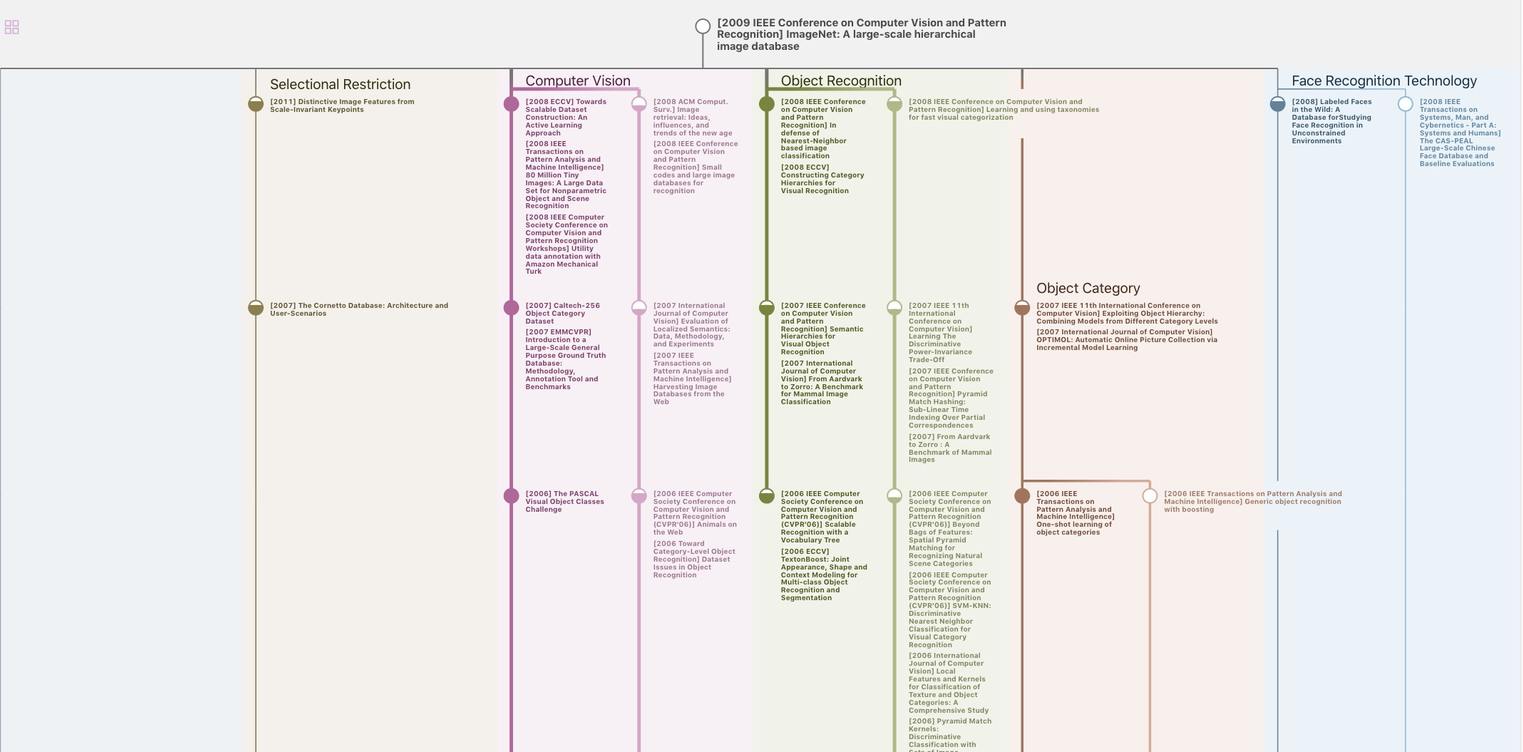
生成溯源树,研究论文发展脉络
Chat Paper
正在生成论文摘要