Hyperbolic Active Learning for Semantic Segmentation under Domain Shift
ICML 2024(2024)
Abstract
We introduce a hyperbolic neural network approach to pixel-level active learning for semantic segmentation. Analysis of the data statistics leads to a novel interpretation of the hyperbolic radius as an indicator of data scarcity. In HALO (Hyperbolic Active Learning Optimization), for the first time, we propose the use of epistemic uncertainty as a data acquisition strategy, following the intuition of selecting data points that are the least known. The hyperbolic radius, complemented by the widely-adopted prediction entropy, effectively approximates epistemic uncertainty. We perform extensive experimental analysis based on two established synthetic-to-real benchmarks, i.e. GTAV $\rightarrow$ Cityscapes and SYNTHIA $\rightarrow$ Cityscapes. Additionally, we test HALO on Cityscape $\rightarrow$ ACDC for domain adaptation under adverse weather conditions, and we benchmark both convolutional and attention-based backbones. HALO sets a new state-of-the-art in active learning for semantic segmentation under domain shift and it is the first active learning approach that surpasses the performance of supervised domain adaptation while using only a small portion of labels (i.e., 1%).
MoreTranslated text
Key words
Active Learning,Transfer Learning,Representation Learning,Meta-Learning,Semi-Supervised Learning
PDF
PPT
Code
Data
View via Publisher
AI Read Science
Must-Reading Tree
Example
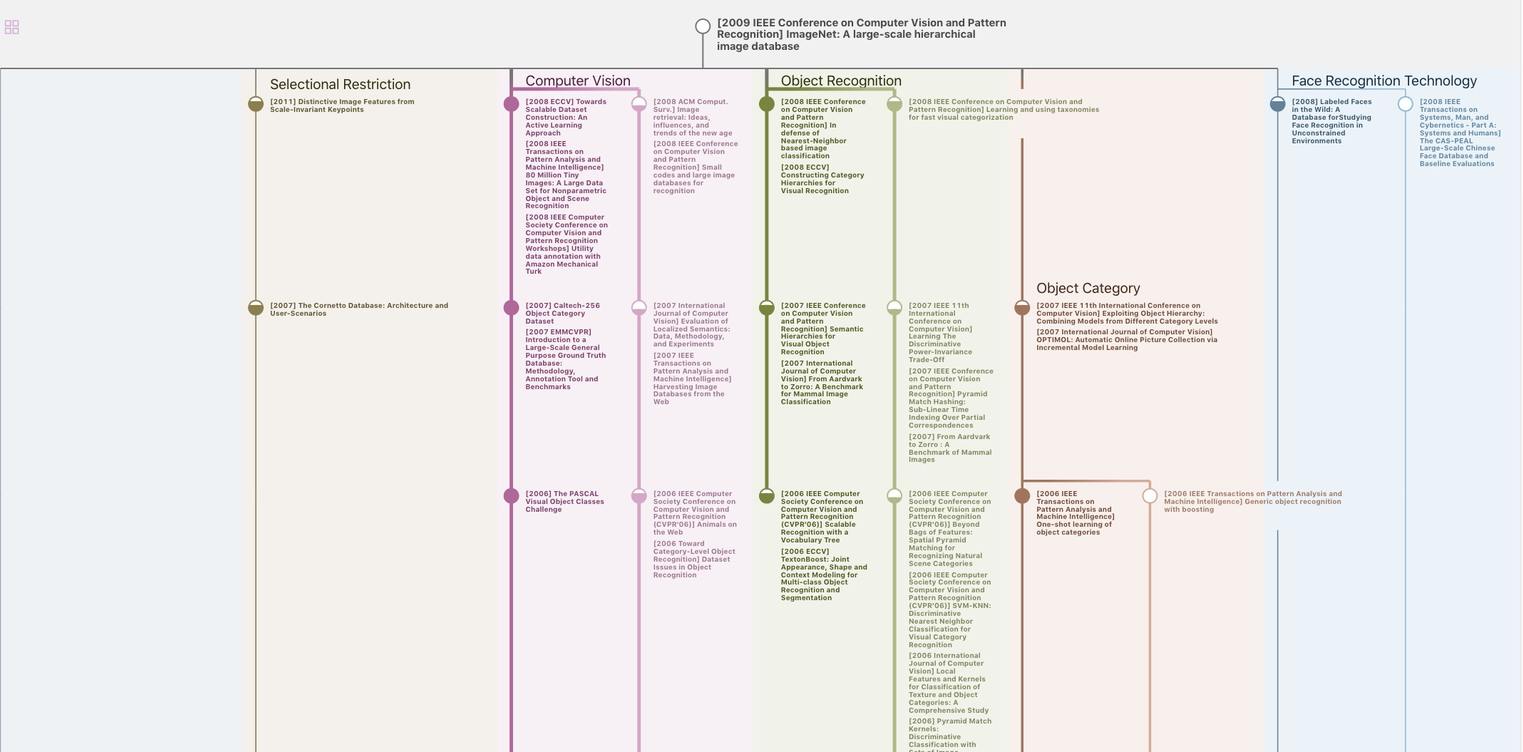
Generate MRT to find the research sequence of this paper
Data Disclaimer
The page data are from open Internet sources, cooperative publishers and automatic analysis results through AI technology. We do not make any commitments and guarantees for the validity, accuracy, correctness, reliability, completeness and timeliness of the page data. If you have any questions, please contact us by email: report@aminer.cn
Chat Paper
Summary is being generated by the instructions you defined