eNODAL: an experimentally guided nutriomics data clustering method to unravel complex drug-diet interactions
bioRxiv (Cold Spring Harbor Laboratory)(2023)
摘要
Unraveling the complex interplay between nutrients and drugs via their effects on ‘omics’ features could revolutionize our fundamental understanding of nutritional physiology, personalized nutrition and ultimately human health-span. Experimental studies in nutrition are starting to use large-scale ‘omics’ experiments to pick apart the effects of such interacting factors. However, the high dimensionality of the omics features, coupled with complex fully-factorial experimental designs together pose a challenge to the analysis. Current strategies for analyzing such types of data are based on between-feature correlations. However, these techniques risk overlooking important signals that arise from the experimental design and produce clusters that are hard to interpret. We present a novel approach for analyzing high-dimensional outcomes in nutriomics experiments, termed e xperiment-guided N utri O mics D at A c L ustering ( eNODAL ). This three-step hybrid framework takes advantage of both ANOVA-type analyses and unsupervised learning methods to extract maximum information from experimental nutriomics studies. First, eNODAL categorizes the omics features into interpretable groups based on the significance of response to the different experimental variables using an ANOVA-like test. Such groups may include the main effects of a nutritional intervention, and drug exposure, or their interaction. Second, consensus clustering is performed within each interpretable group to further identify subclusters of features with similar response profiles to these experimental factors. Third, eNODAL annotates these subclusters based on their experimental responses and biological pathways enriched within the subcluster. We validate eNODAL using data from a mouse experiment to test for the interaction effects of macronutrient intake and drugs that target aging mechanisms in mice.
### Competing Interest Statement
The authors have declared no competing interest.
更多查看译文
关键词
nutriomics data,interactions,drug-diet
AI 理解论文
溯源树
样例
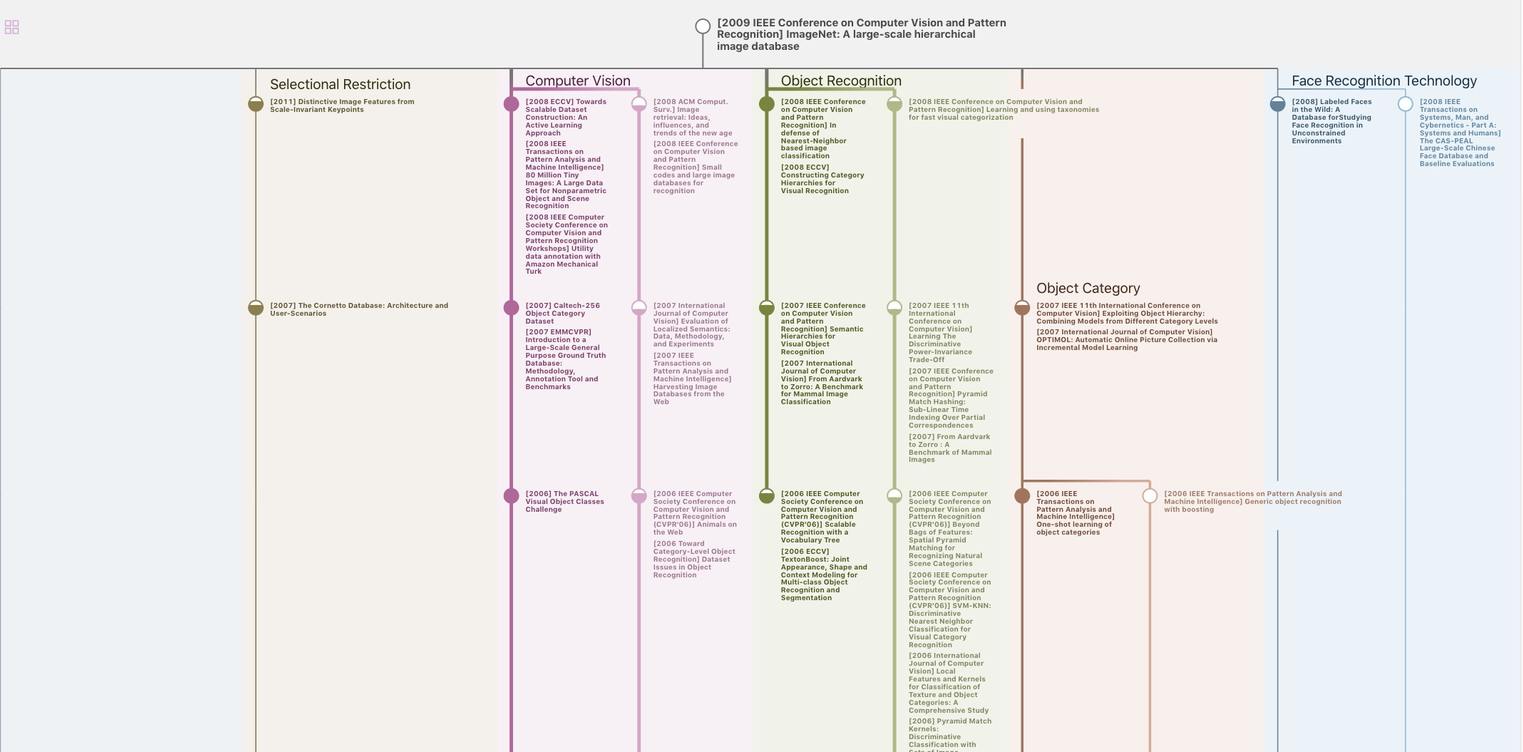
生成溯源树,研究论文发展脉络
Chat Paper
正在生成论文摘要