Surface and Subsurface Oceanographic Features Drive Forage Fish Distributions and Aggregations: Implications for Prey Availability to Top Predators in the US Northeast Shelf Ecosystem
ECOLOGY AND EVOLUTION(2023)
Biodivers Res Inst | Northeast Fisheries Sci Ctr | CSS Inc | Normandeau Associates | Bronx Zoo
Abstract
Forage fishes are a critical food web link in marine ecosystems, aggregating in a hierarchical patch structure over multiple spatial and temporal scales. Surface-level forage fish aggregations (FFAs) represent a concentrated source of prey available to surface- and shallow-foraging marine predators. Existing survey and analysis methods are often imperfect for studying forage fishes at scales appropriate to foraging predators, making it difficult to quantify predator-prey interactions. In many cases, general distributions of forage fish species are known; however, these may not represent surface-level prey availability to predators. Likewise, we lack an understanding of the oceanographic drivers of spatial patterns of prey aggregation and availability or forage fish community patterns. Specifically, we applied Bayesian joint species distribution models to bottom trawl survey data to assess species- and community-level forage fish distribution patterns across the US Northeast Continental Shelf (NES) ecosystem. Aerial digital surveys gathered data on surface FFAs at two project sites within the NES, which we used in a spatially explicit hierarchical Bayesian model to estimate the abundance and size of surface FFAs. We used these models to examine the oceanographic drivers of forage fish distributions and aggregations. Our results suggest that, in the NES, regions of high community species richness are spatially consistent with regions of high surface FFA abundance. Bathymetric depth drove both patterns, while subsurface features, such as mixed layer depth, primarily influenced aggregation behavior and surface features, such as sea surface temperature, sub-mesoscale eddies, and fronts influenced forage fish diversity. In combination, these models help quantify the availability of forage fishes to marine predators and represent a novel application of spatial models to aerial digital survey data.
MoreTranslated text
Key words
forage fish aggregation,hierarchical Bayesian model,joint species distribution model,predator-prey interactions,trophodynamics
求助PDF
上传PDF
View via Publisher
AI Read Science
AI Summary
AI Summary is the key point extracted automatically understanding the full text of the paper, including the background, methods, results, conclusions, icons and other key content, so that you can get the outline of the paper at a glance.
Example
Background
Key content
Introduction
Methods
Results
Related work
Fund
Key content
- Pretraining has recently greatly promoted the development of natural language processing (NLP)
- We show that M6 outperforms the baselines in multimodal downstream tasks, and the large M6 with 10 parameters can reach a better performance
- We propose a method called M6 that is able to process information of multiple modalities and perform both single-modal and cross-modal understanding and generation
- The model is scaled to large model with 10 billion parameters with sophisticated deployment, and the 10 -parameter M6-large is the largest pretrained model in Chinese
- Experimental results show that our proposed M6 outperforms the baseline in a number of downstream tasks concerning both single modality and multiple modalities We will continue the pretraining of extremely large models by increasing data to explore the limit of its performance
Upload PDF to Generate Summary
Must-Reading Tree
Example
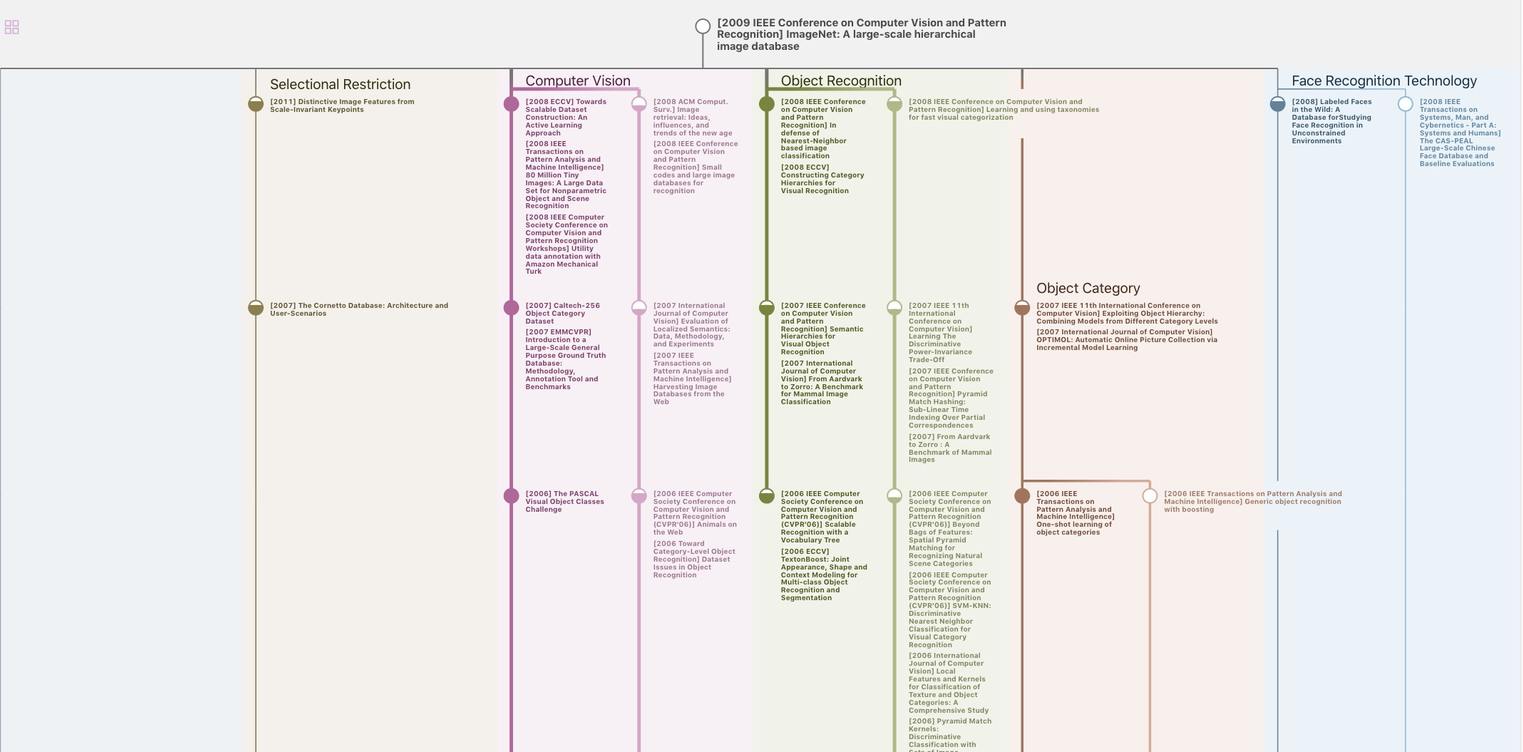
Generate MRT to find the research sequence of this paper
Related Papers
Assessing Small Pelagic Fish Trends in Space and Time Using Piscivore Stomach Contents
CANADIAN JOURNAL OF FISHERIES AND AQUATIC SCIENCES 2024
被引用3
Ecology and Evolution 2024
被引用0
Evaluating Drivers of Recent Large Whale Strandings on the East Coast of the United States.
CONSERVATION BIOLOGY 2024
被引用0
Data Disclaimer
The page data are from open Internet sources, cooperative publishers and automatic analysis results through AI technology. We do not make any commitments and guarantees for the validity, accuracy, correctness, reliability, completeness and timeliness of the page data. If you have any questions, please contact us by email: report@aminer.cn
Chat Paper
GPU is busy, summary generation fails
Rerequest