Monitoring Fracture Saturation using Transportable Acoustic Sources and a Neural Network Differential Autoencoder
IOP conference series(2023)
摘要
Abstract Acoustic signals from transportable acoustic sources were analysed using a Siamese twin neural network differential autoencoder (TDAE) to monitor changing fracture saturation within a laboratory testbed consisting of synthetic fractures. The small transportable acoustic sources, called “chattering dust” and introduced inside the fractures, generate hundreds to thousands of acoustic wavepackets that propagate across other fractures in the system. A 4-class study was performed using the TDAE by physically cycling among four different saturation conditions of the fractures. Accurate classification of all four fracture saturation conditions was achieved using mode-pooling. This work demonstrates that the twin neural network differential autoencoder, which is ideally suited to identify subtle differences among classes, can extract information about the conditions of fractures from complex and variable wavefields consisting of overlapping coda wave signals.
更多查看译文
关键词
fracture saturation,transportable acoustic sources,neural network
AI 理解论文
溯源树
样例
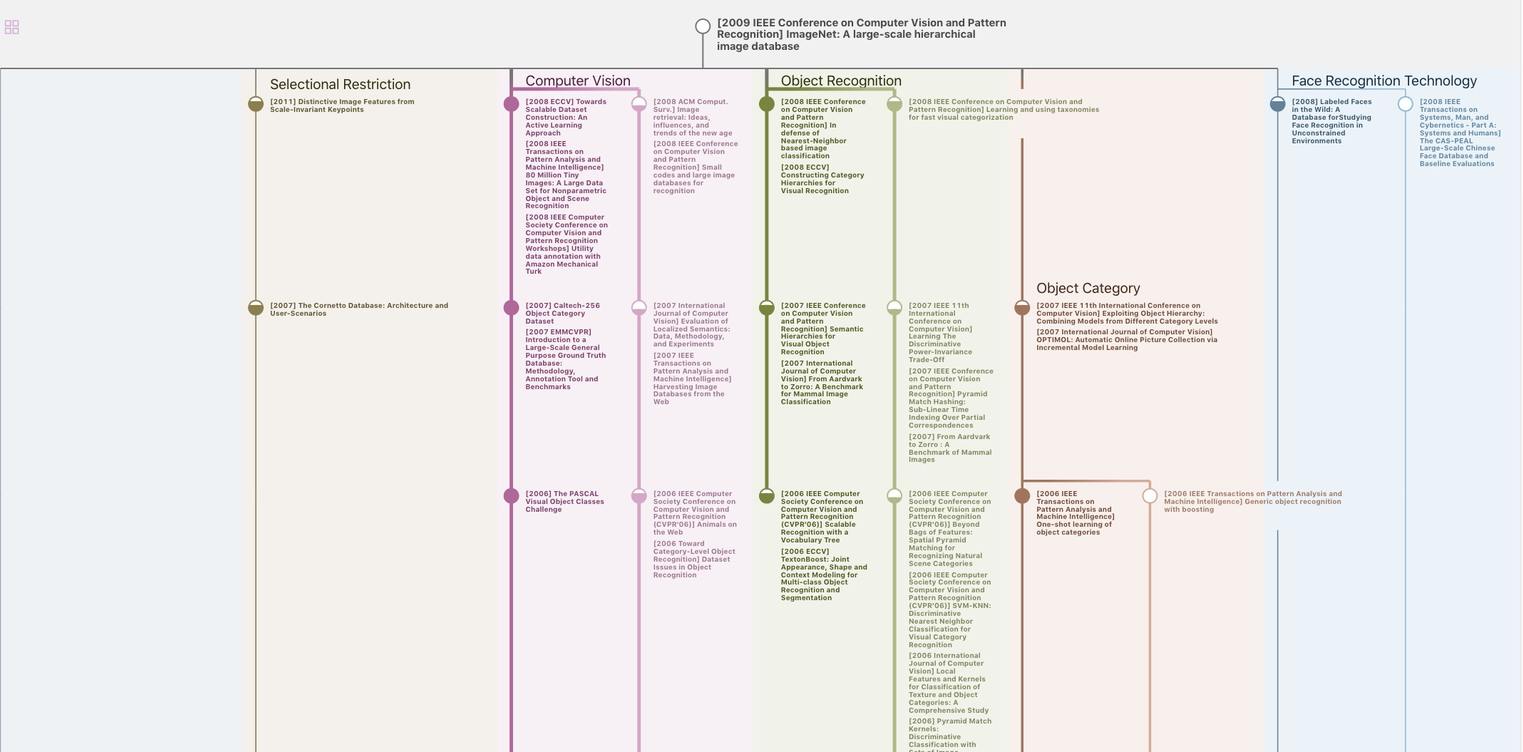
生成溯源树,研究论文发展脉络
Chat Paper
正在生成论文摘要