A Machine Learning-Based Strategy for Experimentally Estimating Force Chains of Granular Materials Using X-ray Micro-Tomography
Géotechnique/Geotechnique(2023)
摘要
A machine learning-based strategy is presented to estimate the contact force chains of uniformly sized spherical granular materials under triaxial compression, using particle kinematics and inter-particle contact evolution data measured by X-ray micro-tomography (μCT). To this end, a graph neural network (GNN) is introduced to predict the contact force chains of granular materials at the end of a shear increment based on the evolution of contact network and grain displacement during that shear increment. Meanwhile, discrete-element modelling (DEM) is performed for a glass bead specimen under triaxial compression with the use of in situ μCT scanning. The DEM model has the same initial conditions as the glass bead specimen and is validated by comparing the calculated stress–strain curves, particle kinematics and inter-particle contact fabric evolution of the numerical specimen with the experimental results. The DEM model is used to generate sufficient virtual data to train the GNN model, which is applied to the glass bead specimen to predict the evolution of contact force chains. The model-predicted results yield a power-law relationship between the above mean normalised particle maximum normal contact forces and the probability density function, which is consistent with the findings reported in previous numerical studies.
更多查看译文
AI 理解论文
溯源树
样例
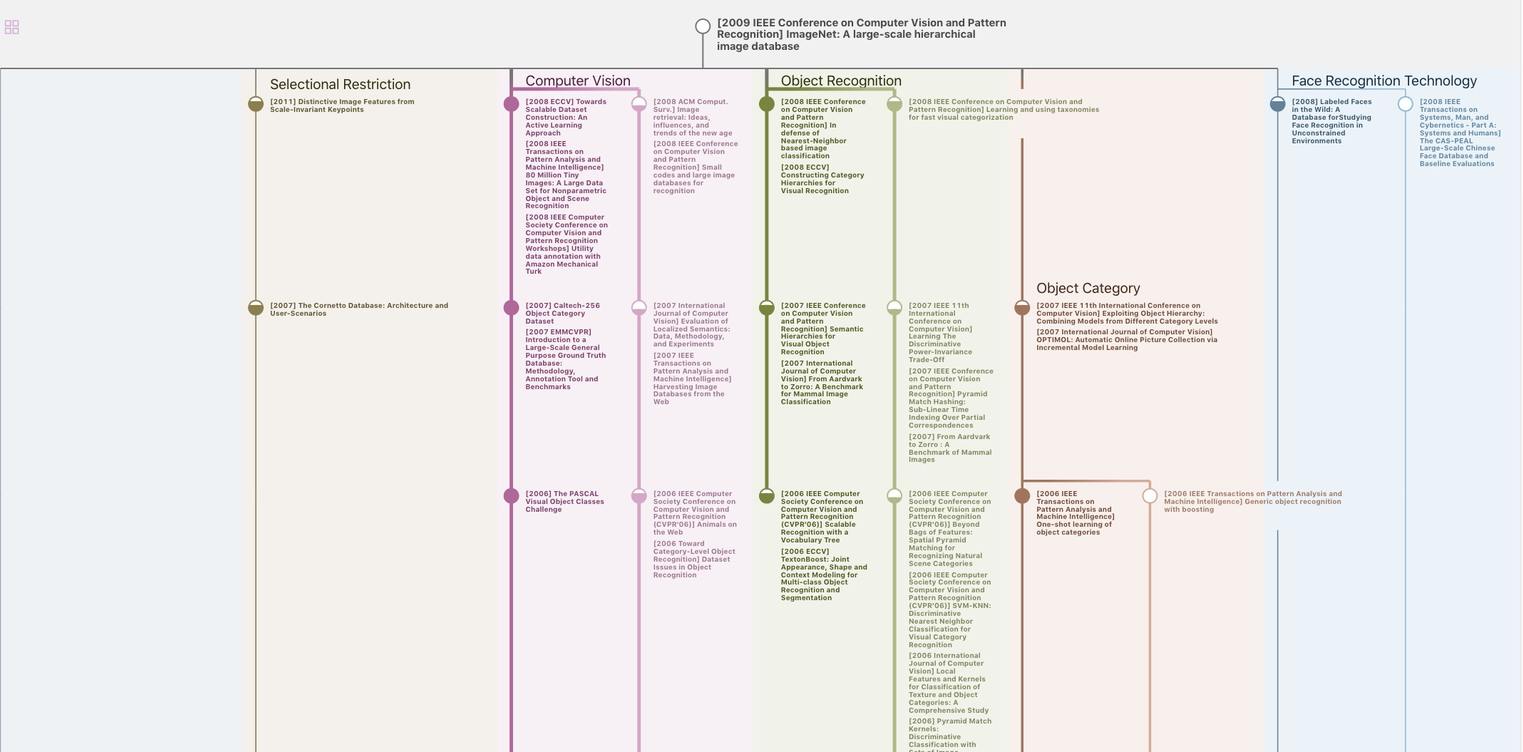
生成溯源树,研究论文发展脉络
Chat Paper
正在生成论文摘要