High-resolution all-sky land surface temperature and net radiation over Europe
crossref(2022)
摘要
Abstract. Land Surface Temperature (LST) and Surface Net Radiation (SNR) are vital inputs for many land surface and hydrological models. However, current remote sensing datasets of these variables come mostly at coarse resolutions. Although high-resolution LST and SNR retrievals are available, they have large gaps due to cloud-cover that hinder their use as input in models. Here, we present a downscaled and continuous daily LST and SNR product across Europe for 2018–2019. The LST product is based on all-sky LST retrievals from the Spinning Enhanced Visible and InfraRed Imager (SEVIRI) onboard the geostationary Meteosat Second Generation (MSG) satellite, and clear-sky LST retrievals from the Sea and Land Surface Temperature Radiometer (SLSTR) onboard the polar-orbiting Sentinel 3 satellites. The product combines the medium spatial (approx. 5–7 km) but high temporal (30 minute) resolution, gap-free data from MSG, with the low temporal (2–3 days) but high spatial (1 km) resolution of the Sentinel 3 LST retrievals. The resulting 1 km and daily LST dataset is based on an hourly merging of both datasets through bias-correction and Kalman Filter assimilation. Longwave outgoing radiation is computed from the merged LST product in combination with MSG-based emissivity data. Shortwave outgoing radiation is computed from the incoming shortwave radiation from MSG and downscaled albedos using 1 km PROBA-V data. MSG incoming shortwave and longwave radiation and the outgoing radiation components at 1 km spatial resolution are used together to compute the final daily SNR dataset in a consistent manner. Validation results indicate an improvement of the root mean squared error by ca. 8 % with a substantial increase in spatial detail compared to the original MSG product. The resulting pan-European LST and SNR dataset can be used for hydrological modelling and as input to models dedicated to estimating evaporation and surface turbulent heat fluxes and will be regularly updated in the future.
更多查看译文
AI 理解论文
溯源树
样例
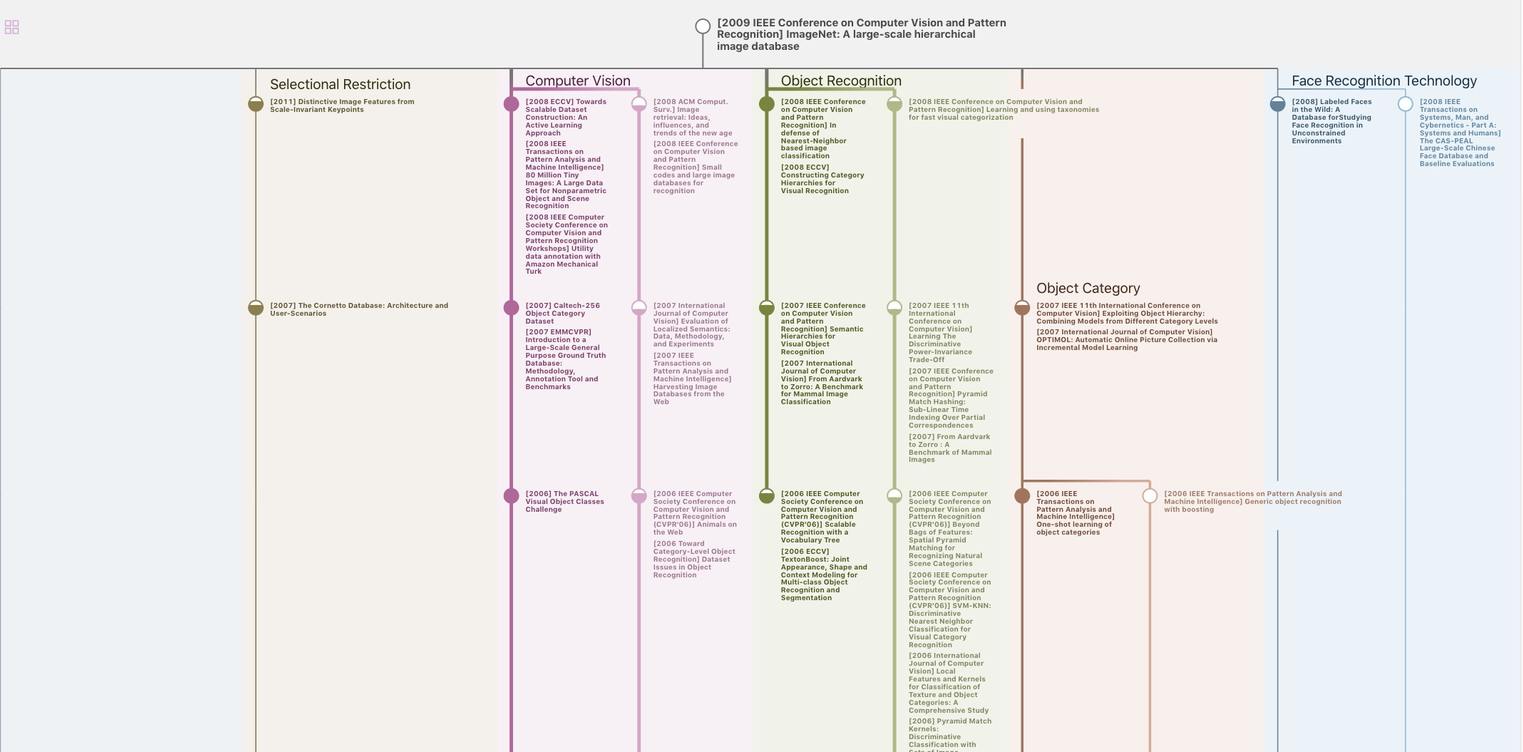
生成溯源树,研究论文发展脉络
Chat Paper
正在生成论文摘要