Unraveling the Secrets of Plant Roots: Simplified Method for Large Scale Root Exudate Sampling and Analysis in Arabidopsis Thaliana
Open Research Europe(2023)
Department of Ecoscience | Department of Chemistry | Department of Molecular Biology and Genetics - Plant Molecular Biology
Abstract
Background:Plants exude a plethora of compounds to communicate with their environment. Although much is known about above-ground plant communication, we are only beginning to fathom the complexities of below-ground chemical communication channels. Studying root-exuded compounds and their role in plant communication has been difficult due to the lack of standardized methodologies. Here, we develop an interdisciplinary workflow to explore the natural variation in root exudate chemical composition of the model plant Arabidopsis thaliana. We highlight key challenges associated with sampling strategies and develop a framework for analyzing both narrow- and broad-scale patterns of root exudate composition in a large set of natural A. thaliana accessions.Methods:Our method involves cultivating individual seedlings in vitro inside a plastic mesh, followed by a short hydroponic sampling period in small quantities of ultrapure water. The mesh makes it easy to handle plants of different sizes and allows for large-scale characterization of individual plant root exudates under axenic conditions. This setup can also be easily extended for prolonged temporal exudate collection experiments. Furthermore, the short sampling time minimizes the duration of the experiment while still providing sufficient signal even with small volume of the sampling solution. We used ultra-high performance liquid chromatography coupled with quadrupole time-of-flight mass spectrometry (UHPLC-QTOF-MS) for untargeted metabolic profiling, followed by tentative compound identification using MZmine3 and SIRIUS 5 software, to capture a broad overview of root exudate composition in A. thaliana accessions.Results:Based on 28 replicates of the Columbia genotype (Col-0) compared with 10 random controls, MZmine3 annotated 354 metabolites to be present only in Col-0 by negative ionization. Of these, 254 compounds could be annotated by SIRIUS 5 software.Conclusions:The methodology developed in this study can be used to broadly investigate the role of root exudates as chemical signals in plant belowground interactions.
MoreTranslated text
Key words
root exudates,natural variation,Arabidopsis thaliana,interdisciplinary,chemical analysis,eng
求助PDF
上传PDF
View via Publisher
AI Read Science
AI Summary
AI Summary is the key point extracted automatically understanding the full text of the paper, including the background, methods, results, conclusions, icons and other key content, so that you can get the outline of the paper at a glance.
Example
Background
Key content
Introduction
Methods
Results
Related work
Fund
Key content
- Pretraining has recently greatly promoted the development of natural language processing (NLP)
- We show that M6 outperforms the baselines in multimodal downstream tasks, and the large M6 with 10 parameters can reach a better performance
- We propose a method called M6 that is able to process information of multiple modalities and perform both single-modal and cross-modal understanding and generation
- The model is scaled to large model with 10 billion parameters with sophisticated deployment, and the 10 -parameter M6-large is the largest pretrained model in Chinese
- Experimental results show that our proposed M6 outperforms the baseline in a number of downstream tasks concerning both single modality and multiple modalities We will continue the pretraining of extremely large models by increasing data to explore the limit of its performance
Upload PDF to Generate Summary
Must-Reading Tree
Example
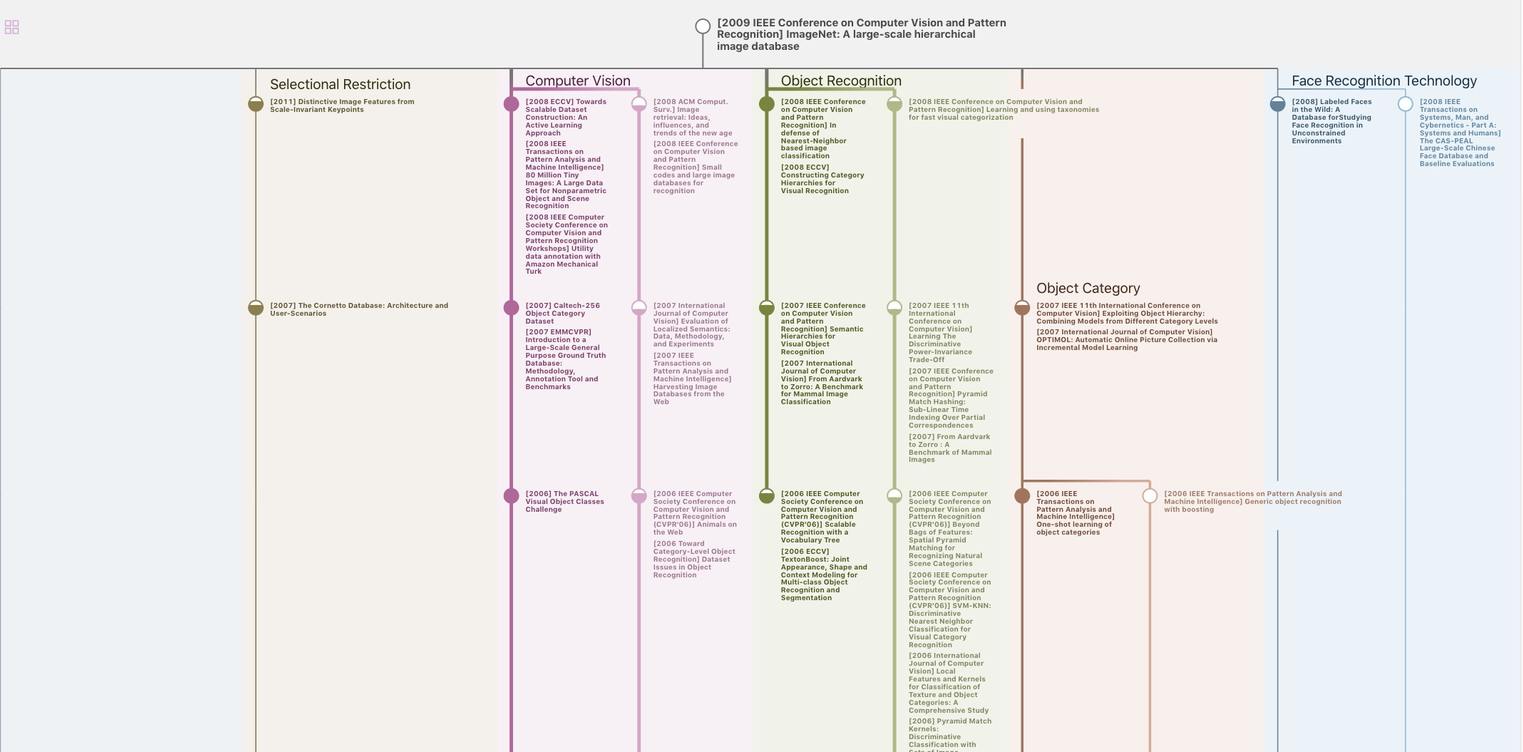
Generate MRT to find the research sequence of this paper
Related Papers
2006
被引用67 | 浏览
2013
被引用172 | 浏览
2014
被引用215 | 浏览
2013
被引用137 | 浏览
2017
被引用177 | 浏览
2019
被引用580 | 浏览
2016
被引用230 | 浏览
2021
被引用26 | 浏览
2021
被引用109 | 浏览
Data Disclaimer
The page data are from open Internet sources, cooperative publishers and automatic analysis results through AI technology. We do not make any commitments and guarantees for the validity, accuracy, correctness, reliability, completeness and timeliness of the page data. If you have any questions, please contact us by email: report@aminer.cn
Chat Paper