A Prior Knowledge-Informed Traceable Neutral Network Modeling Only Using Regular Laboratory Results to Assist Early Diagnosis for Tuberculosis
openalex(2022)
摘要
AbstractBackground To construct a knowledge-informed traceable artificial intelligence (AI)-based model to assist early diagnosis for tuberculosis (TB). Methods 60729 cases were extracted from January 1, 2014, to December 31, 2021, in Beijing Hepingli Hospital. Only using routine laboratory results, five AI-based algorithms were evaluated by accuracy (ACC), area under the receiver operating characteristic curve (AUC), specificity (SPE) and sensitivity(SEN). A Neutral Network (NN) algorithm combined with clinical prior knowledge was designed. SHAP algorithm together with means of metrology was used to improve model explanation. Results For disease screening, our NN model overall performed better (AUC = 0.9913) than the other algorithm models. When differentiating TB with healthy control (HC), the AUC, ACC, SPE and SEN were 0.9759, 0.9348, 0.9389 and 0.9124 respectively. The AUC was 0.8035 for distinguishing pulmonary tuberculosis (PTB) with other pulmonary diseases (OPD), The AUC was 0.7761 in the identification of TB in different parts. The average iteration epochs of the NN model prior-knowledge introduced was only 87.7, while the NN model without prior-knowledge was 190.7. SHAP algorithm together with the evaluation of measurement uncertainty in metrology not only illustrated the relationship of the mortality risk and each test item, but quantitatively the bias and variance of model and data source separately. Conclusions A knowledge-informed AI-based model only based on regular laboratory results offers a more convenient, effective, and highly accurate early diagnosis tool for TB. The ACC of our model was firstly quantitative evaluated through national reference data set traceable to National Institute of Metrology, China.
更多查看译文
关键词
Pneumonia Detection,Transfer Learning,Automated Diagnosis
AI 理解论文
溯源树
样例
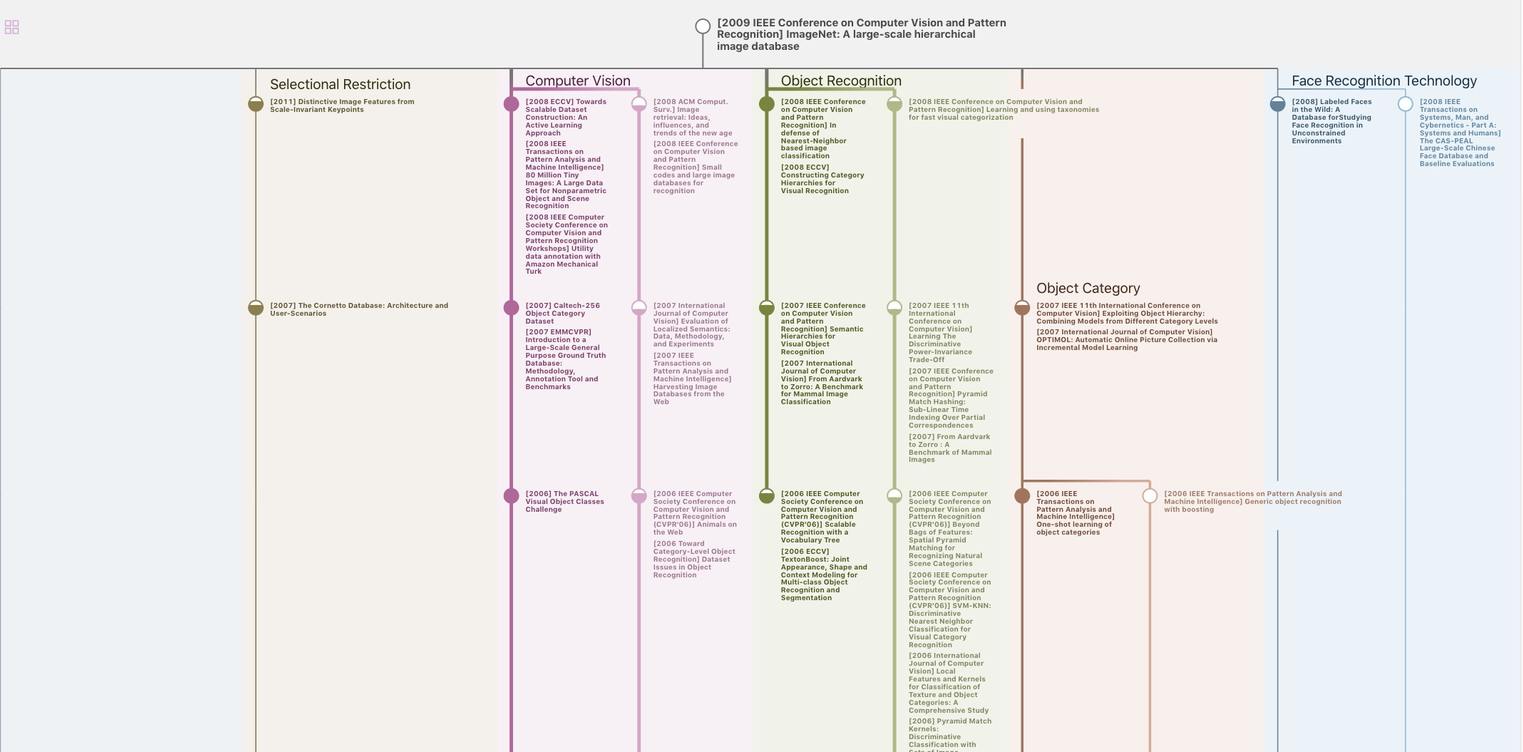
生成溯源树,研究论文发展脉络
Chat Paper
正在生成论文摘要