Development of an Automated Drug Detection System on Social Media (Preprint)
crossref(2022)
摘要
BACKGROUND One of the hallmarks of unregulated drug markets is their unpredictability and constant evolution with newly introduced substances. People who use drugs and the public health workforce are often unaware of the appearance of new drugs on the unregulated market and their type, safe dosage, and potential adverse effects. This increases risks to people who use drugs, including the risk of unknown consumption and unintentional drug poisoning. Early Warning Systems can help monitor the landscape of emerging drugs in a given community by collecting and tracking up-to-date information and determining trends. However, there are currently few ways to systematically monitor the appearance and harms of new drugs on the unregulated market in Canada. OBJECTIVE The goal of this work is to examine how artificial intelligence can assist in identifying patterns of drug-related risks and harms, specifically by monitoring the social media activity of public health and law enforcement groups. This information is beneficial in the form of an Early Warning System as it can be used to identify new and emerging drug trends in various communities. METHODS To build a dataset for this study, 145 relevant Twitter accounts throughout Quebec (33), Ontario (78), and British Columbia (34) were manually identified. Tweets posted between August 23 and December 21, 2021 were collected via the Twitter API for a total of 40,393 tweets. Next, subject matter experts 1) developed a keyword filter that reduced the dataset to 3,746 tweets and 2) manually identified which tweets were relevant to monitoring and early warning efforts for a total of 464 tweets. Using this information, a zero-shot classifier was applied to tweets from step 1 with a set of keep (drug arrest, drug discovery, drug report) and not keep (drug addiction support, public safety report, other) labels to see how accurately it could extract the tweets identified in step 2. RESULTS When looking at the accuracy in identifying relevant posts, the system extracted a total of 523 tweets and had an overlap of 397/477 (specificity of ~83.2%) with the subject matter experts. Conversely, the system identified a total of 3,184 irrelevant tweets and had an overlap of 3,104/3,230 (sensitivity of ~96.1%) with the subject matter experts. CONCLUSIONS This study demonstrates the benefits of using artificial intelligence to assist in finding relevant tweets for an Early Warning System. The results showed that it can be quite accurate in filtering out irrelevant information which greatly reduces the amount of manual work required. Although the accuracy in retaining relevant information was observed to be lower, an analysis showed that the label definitions can impact the results significantly and would therefore be suitable for future work to refine. Nonetheless, the performance is promising and demonstrates the usefulness of artificial intelligence in this domain.
更多查看译文
AI 理解论文
溯源树
样例
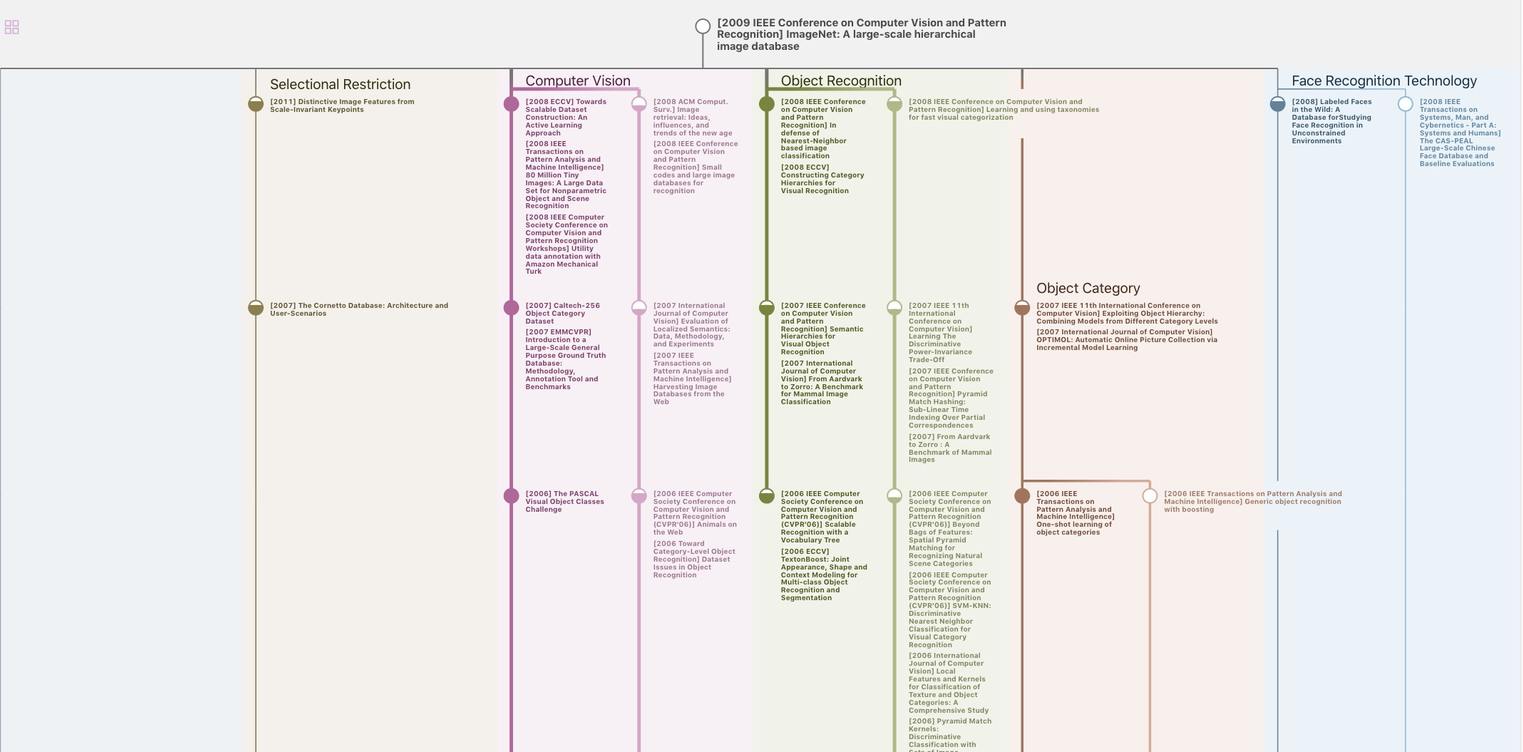
生成溯源树,研究论文发展脉络
Chat Paper
正在生成论文摘要