Dual Attention Recommendation Algorithm Based on Item Attributes
crossref(2022)
摘要
Abstract Massive information often make users lose their focuses so that desired targets are missed. Recommendation system can efficiently provide interesting information for users in the case of "information overload". Most existing recommendation algorithms either only consider the user's overall evaluation on items, or only consider the overall attributes of items. However, the impact of individual attributes of users are often ignored. Hence, this paper proposes a Dual most Relevant Attention recommendation model based on item Attributes (DRAA), comprehensively considering user-item evaluations, individual item attributes and their mutual influence. Firstly, each user's overall preferences for items are built from user-item history interactions. Then, the local self-attention network is used to mine more fine-grained user preferences for individual attributes of items. Finally, a global attention network is applied to distinguish the degrees of importance between the two types of preferences. Extensive experiments on two public data sets demonstrate that our model outperforms the state-of-the-art deep models in terms of prediction accuracy and addressing the problems of cold start and data sparsity.
更多查看译文
AI 理解论文
溯源树
样例
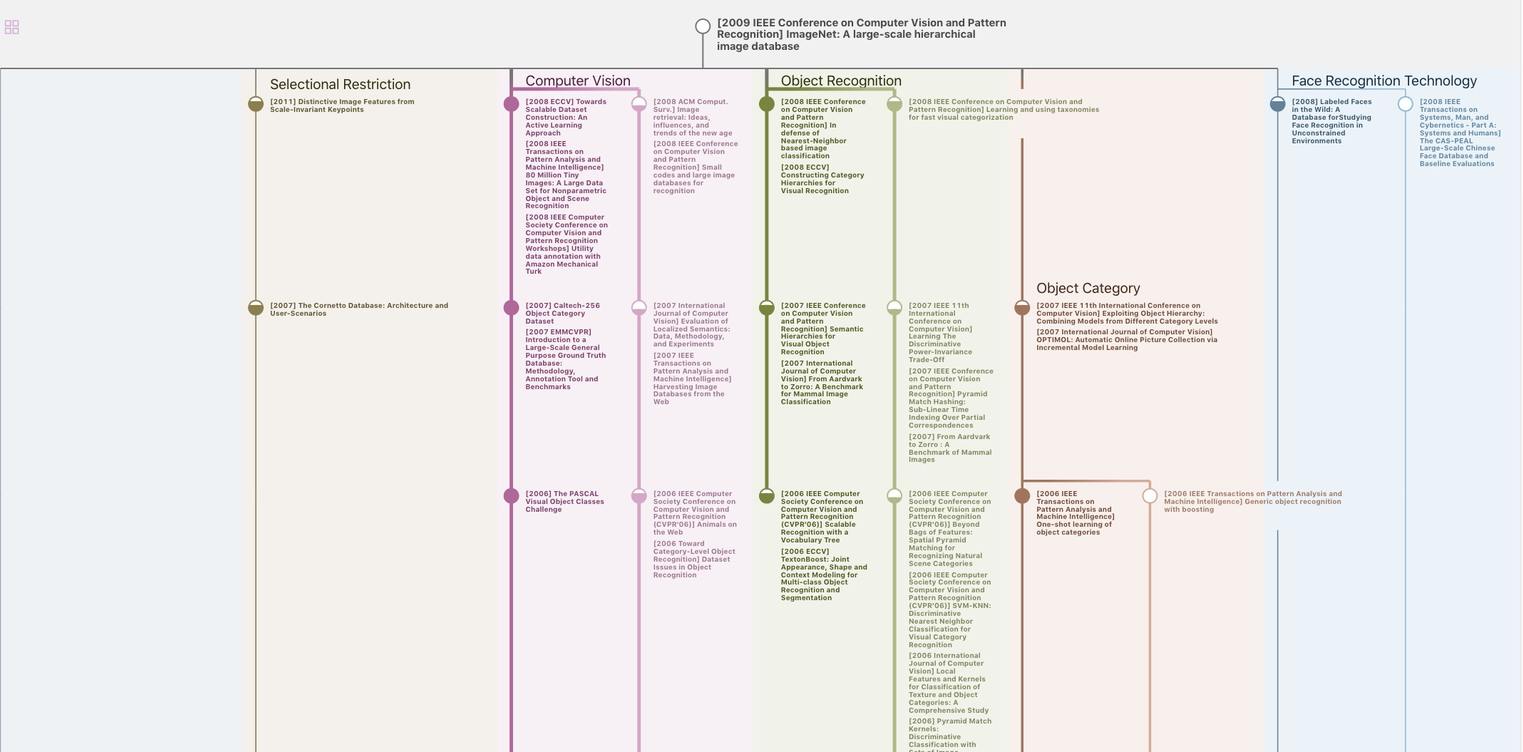
生成溯源树,研究论文发展脉络
Chat Paper
正在生成论文摘要