Accuracy of Predicting IgHV Mutation Status in Chronic Lymphocytic Leukemia Using RNA Expression Profiling and Machine Learning
Journal of Medical Artificial Intelligence(2022)
AI 理解论文
溯源树
样例
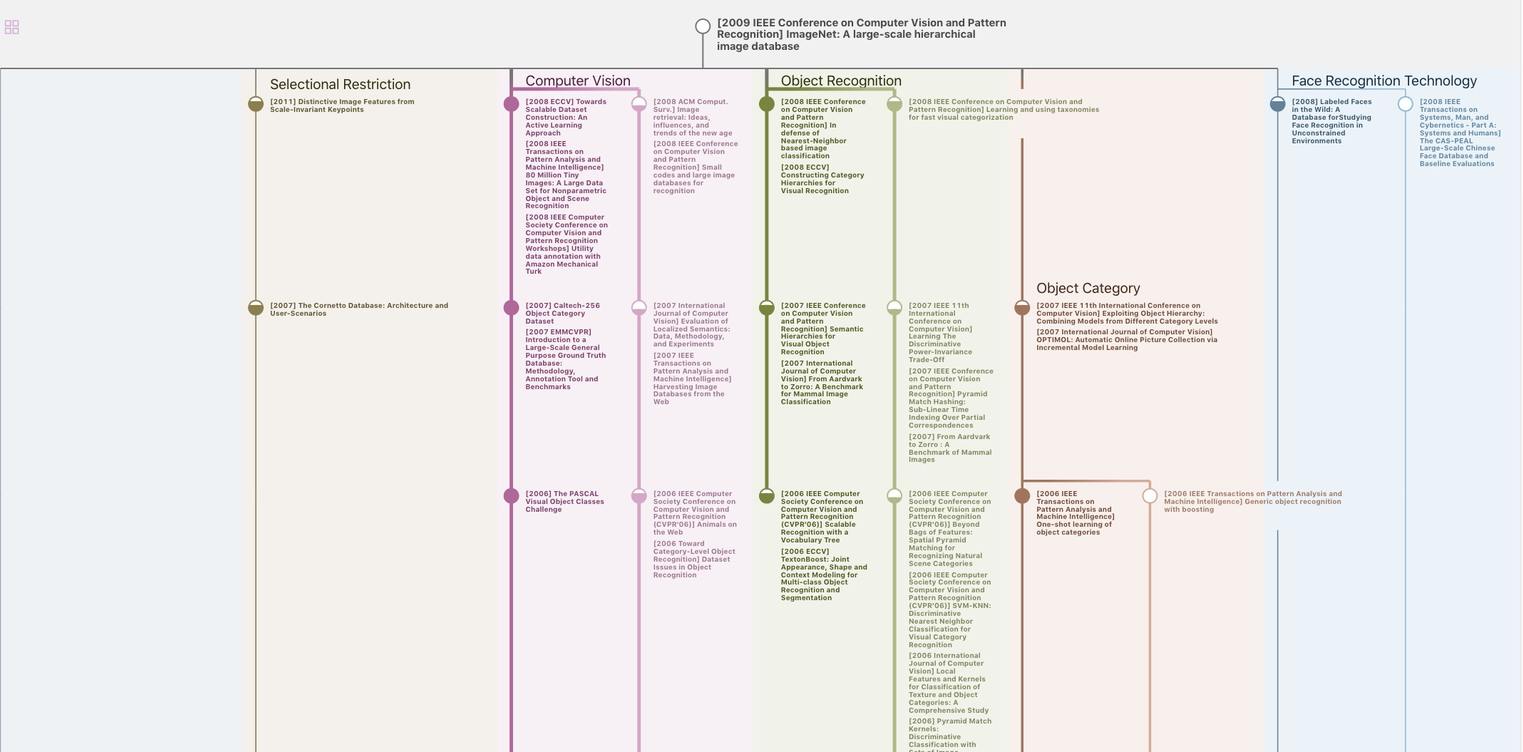
生成溯源树,研究论文发展脉络
Chat Paper
正在生成论文摘要
Journal of Medical Artificial Intelligence(2022)