Empirical Mode Decomposition with Multivariable Time Series Feature Learning for QoS Prediction
Research Square (Research Square)(2022)
摘要
Abstract Predicting the quality of service (QoS) for Web services has become an essential topic in services computing. Existing QoS prediction approaches typically treat each QoS record as a basic unit, ignoring that a QoS time series is a hybrid sequence with multiple frequency features. As a result, we propose AE-mLSTM, a hybrid QoS forecasting method that combines the empirical mode decomposition (EMD) model and the multivariable LSTM model, to capture the intrinsic properties of time-series QoS records. In addition, AE-mLSTM employs an attention mechanism for multi-task learning, which can learn the shared representation of different tasks and jointly predict the tasks of QoS and timing direction. Experiments conducted on two real-world datasets demonstrate that our approach outperforms several state-of-the-art QoS prediction methods. It can improve the average RMSE, MAPE, and MAE by 5.05%, 4.68%, and 5.74% for the Web service dataset and by 10.99%, 12.33%, and 10.93% for the IoT service dataset.
更多查看译文
AI 理解论文
溯源树
样例
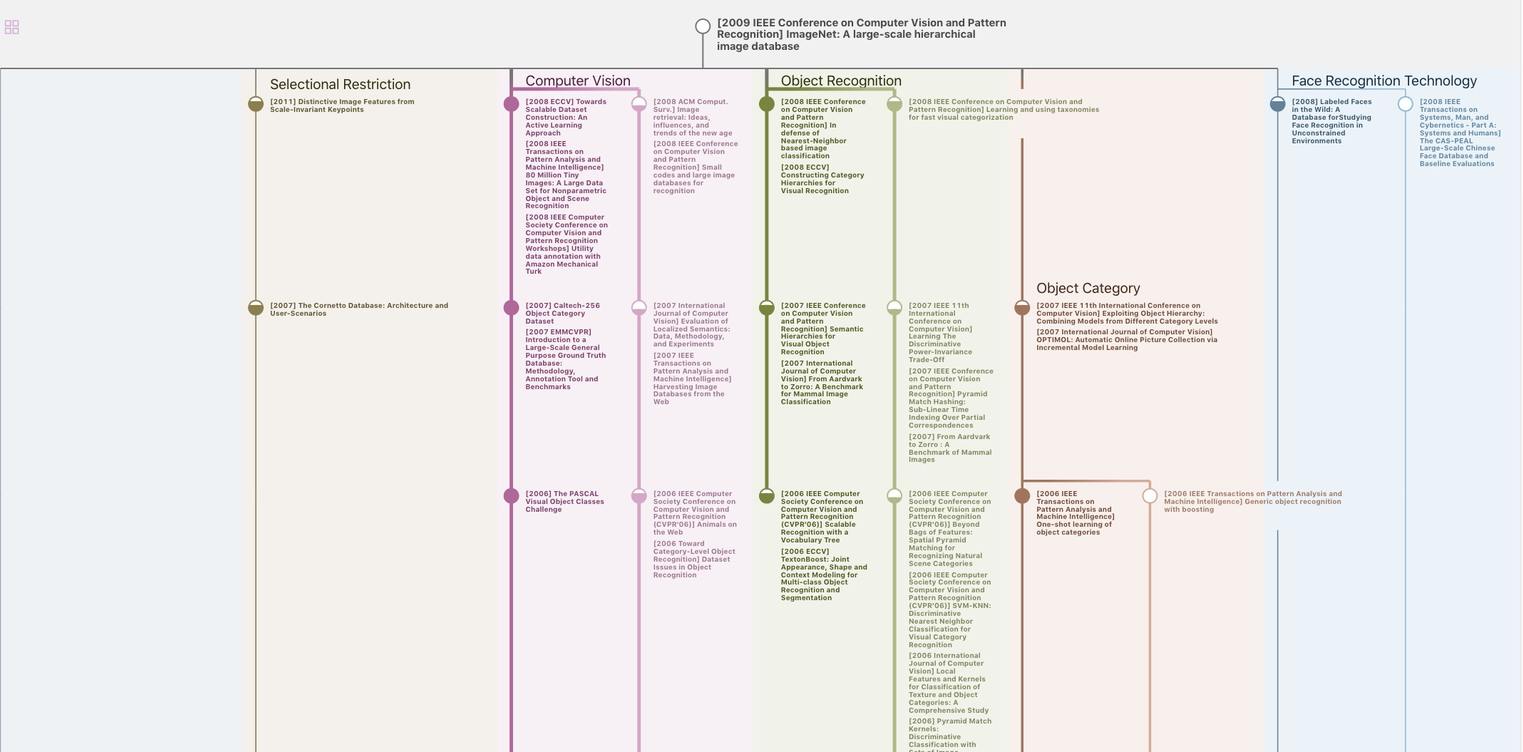
生成溯源树,研究论文发展脉络
Chat Paper
正在生成论文摘要