Power Electronics in HEP Experimental Caverns
Proceedings of 41st International Conference on High Energy physics — PoS(ICHEP2022)(2022)
摘要
Next generation high energy physics experiments will be more granular than those currently in operation, this means more demanding electronics to power the detectors and to process all collected data.Space constraints, cabling, cooling and, last but not least, efficiency are all parameters that need to be optimized during experiment design to have the best performance for data taking.The CAEN R&D to develop a new generation of power supplies for hostile environment is presented and some results from test campaigns are discussed.This R&D named EASY6000, after its predecessors EASY3000 and EASY4000, was launched in 2020 designing and testing electronics capable to survive magnetic fields and mixed radiation fields (composed by gamma, neutral and charge hadrons) of the High Luminosity LHC (HL-LHC) experimental caverns at CERN.To cover all needs, CAEN started an irradiation campaign in various steps and at various irradiation facilities, with the aim to investigate COTS (Commercial Off-The-Shelf) electronics behavior using one radiation at a time: neutron, gamma, protons, and then validate the final board design with a mixed field.Plus, we performed efficiency tests in magnetic fields up to 1 T using various orientations to exploit different symmetries in the boards design.During this talk we will focus on test campaigns performed in the last year.Some undertaken within the RADNEXT EU project and in collaboration with INFN and CERN, that include tests with proton, neutron, and gamma sources, of various components: ADCs, DACs, RAMs, FPGAs, Controllers, Power Transistors and FETs, temperature and humidity sensors, etc.All the necessary pieces to design and build circuits and boards capable to survive in the experiments; these components alone cannot ensure the reliability to run an experiment in such conditions, thus also circuits and control loops must be tested.The results of the test campaigns will be discussed together with some mitigation techniques used to achieve the wanted reliability.First developments of power supply circuits and devices based on these blocks will be also presented, as well as the performances achieved so far in terms of reliability, power density, energy efficiency, noise figure, etc.
更多查看译文
AI 理解论文
溯源树
样例
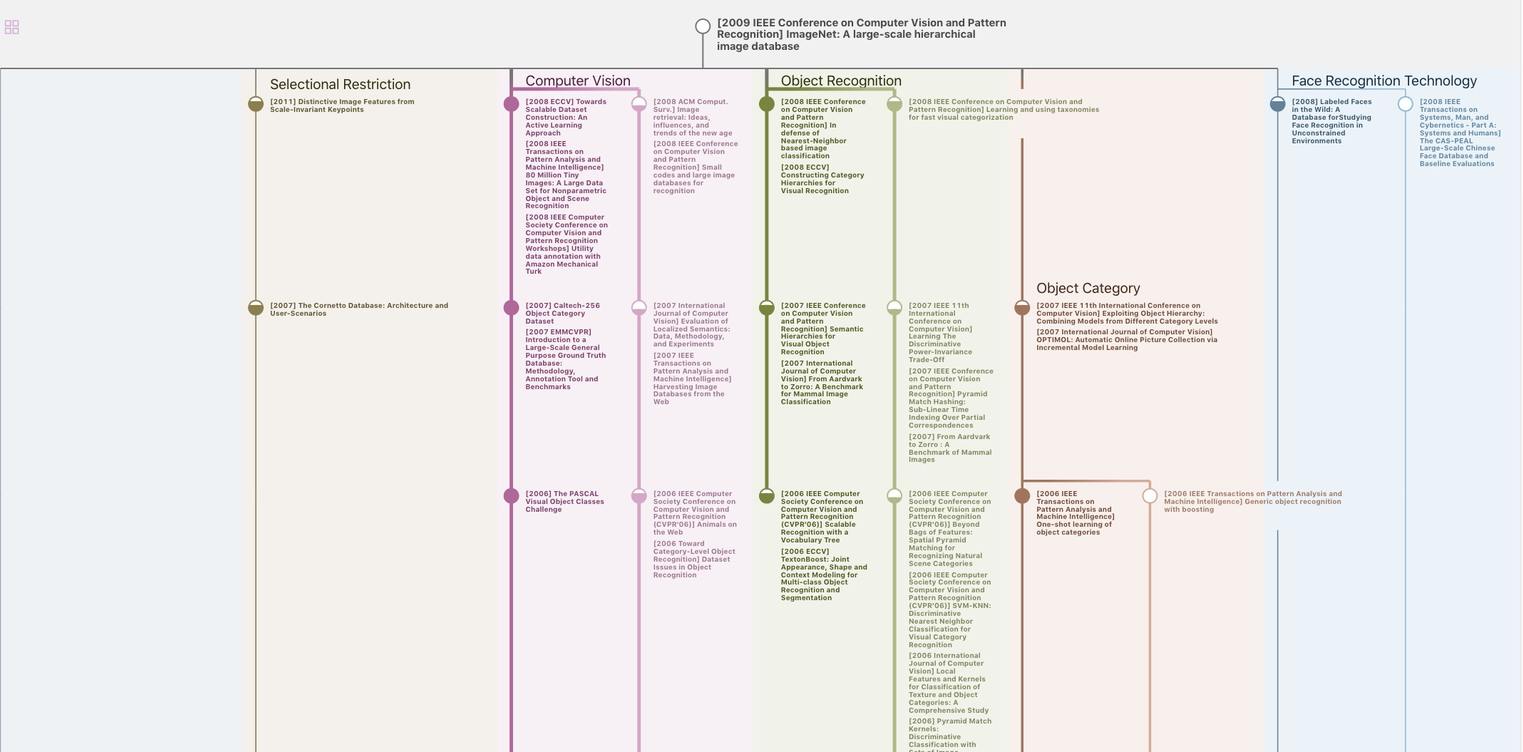
生成溯源树,研究论文发展脉络
Chat Paper
正在生成论文摘要