Grouped Network Poisson Autoregressive Model
STATISTICA SINICA(2024)
摘要
Although multivariate Poisson autoregressive models are popular for fitting count time series data, statistical inferences are quite challenging. The network Poisson autoregressive (NPAR) model reduces the inference complexity by incorporating network information into the dependence structure, where the response of each individual can be explained by its lagged values and the average effect of its neighbors. However, the NPAR model makes the strong assumption that all individuals are homogeneous and share a common autoregressive coefficient. Here, we propose a grouped network Poisson autoregressive (GNPAR) model, in which individuals are classified into groups, using group-specific parameters to describe heterogeneous nodal behaviors. We present the stationarity and ergodicity of the GNPAR model and study the asymptotic properties of the maximum likelihood estimation. We develop an expectation-maximization algorithm to estimate the unknown group labels, and investigate the finite-sample performance of our estimation procedure using simulations. We analyze Chicago Police Investigatory Stop Report data, and find distinct dependence patterns in different neighborhoods of Chicago, which may help with future crime prevention.
更多查看译文
关键词
EM algorithm,individual heterogeneity,maximum likelihood estimation,multivariate Poisson autoregression,network data
AI 理解论文
溯源树
样例
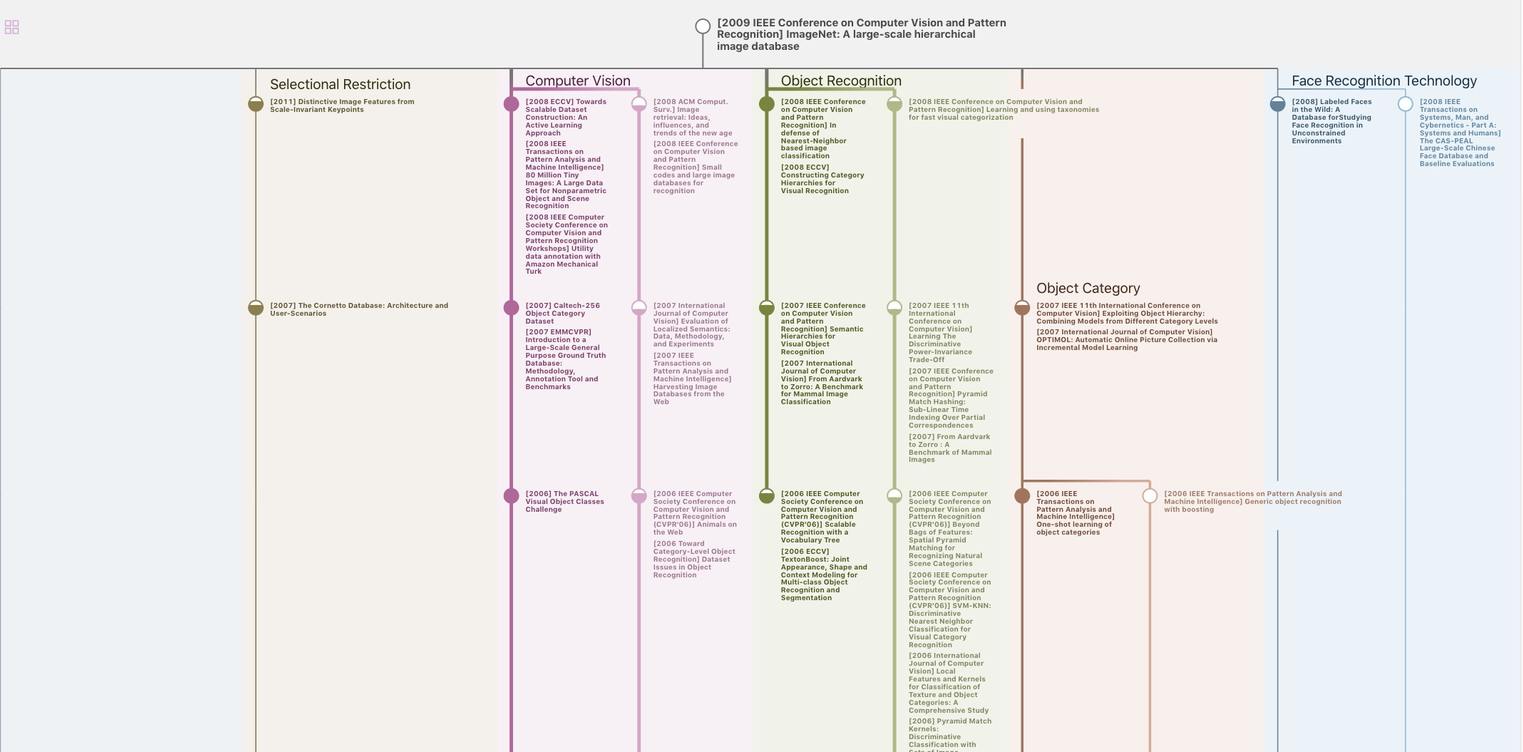
生成溯源树,研究论文发展脉络
Chat Paper
正在生成论文摘要