Analysis of Missing Value Imputation Application with K-Nearest Neighbor (K-NN) Algorithm in Dataset
The IJICS (International Journal of Informatics and Computer Science)(2021)
摘要
Missing value is a problem that is still often found in many studies. Missing value is where data or data features are not available completely and intact. This still happens a lot in datasets that will be used in research. The missing value is caused by many factors such as human error, unavailable data or even from a virus in the database. Data is important for research, incomplete data will affect the results obtained. Data mining is a process that is very influential on data, including the classification process. Classification in data mining can be done if the data is complete. These problems can be overcome by the Imputation process by combining it with the K-Nearest Neighbor process or the process can be called K-Nearest Neighbor Imputation (K-NNI). In the research that has been done the K-Nearest Neighbor Imputation algorithm can overcome the problem of missing values in the dataset. This can be seen from the level of accuracy obtained where the accuracy of the classification process before handling the missing value is 77.01% while after the imputation process the accuracy is 78.31%
更多查看译文
关键词
K-Means Clustering
AI 理解论文
溯源树
样例
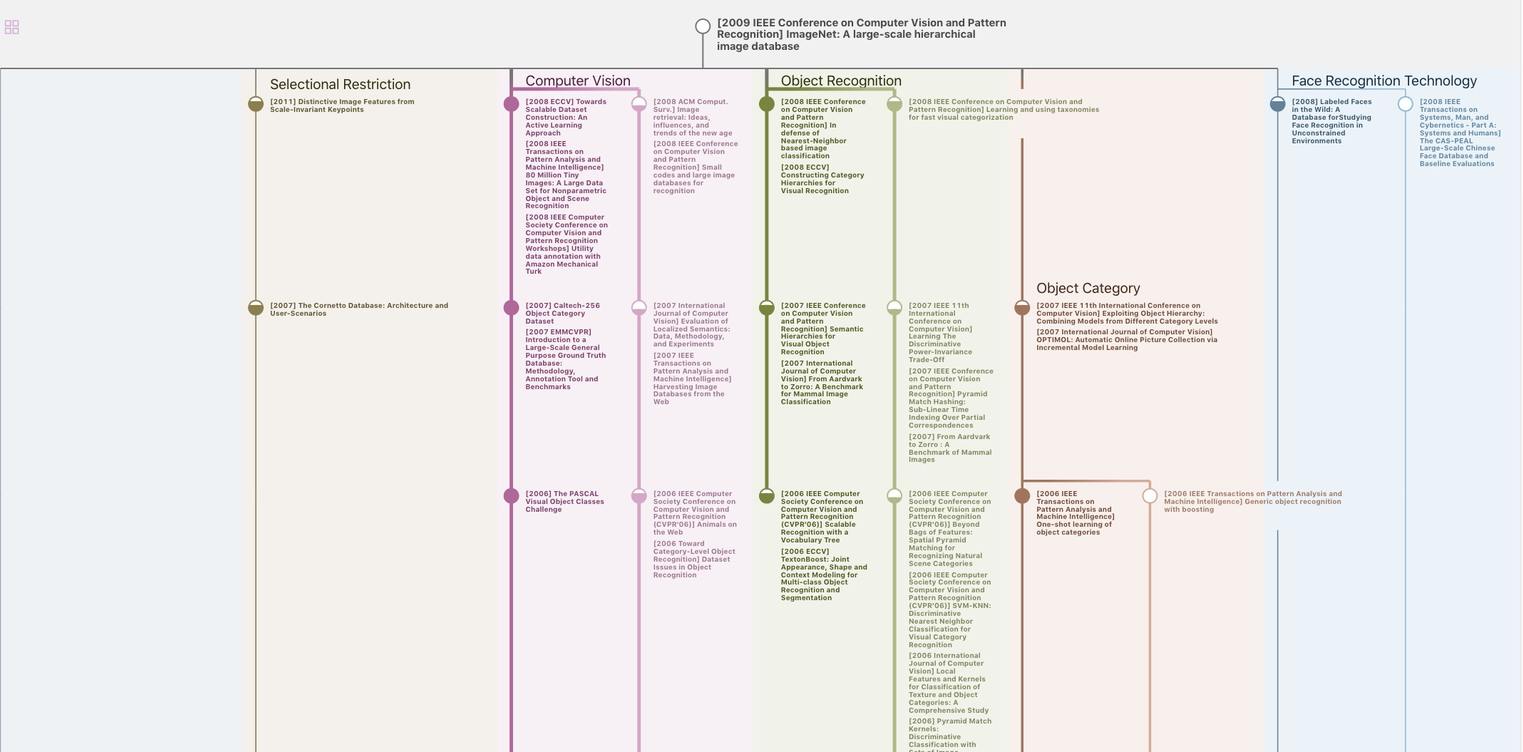
生成溯源树,研究论文发展脉络
Chat Paper
正在生成论文摘要