Neural Wavelet-domain Diffusion for 3D Shape Generation, Inversion, and Manipulation
ACM Transactions on Graphics(2024)
摘要
This paper presents a new approach for 3D shape generation, inversion, and manipulation, through a direct generative modeling on a continuous implicit representation in wavelet domain. Specifically, we propose a compact wavelet representation with a pair of coarse and detail coefficient volumes to implicitly represent 3D shapes via truncated signed distance functions and multi-scale biorthogonal wavelets. Then, we design a pair of neural networks: a diffusion-based generator to produce diverse shapes in the form of the coarse coefficient volumes and a detail predictor to produce compatible detail coefficient volumes for introducing fine structures and details. Further, we may jointly train an encoder network to learn a latent space for inverting shapes, allowing us to enable a rich variety of whole-shape and region-aware shape manipulations. Both quantitative and qualitative experimental results manifest the compelling shape generation, inversion, and manipulation capabilities of our approach over the state-of-the-art methods.
更多查看译文
关键词
Shape generation,shape manipulation,diffusion model,wavelet representation
AI 理解论文
溯源树
样例
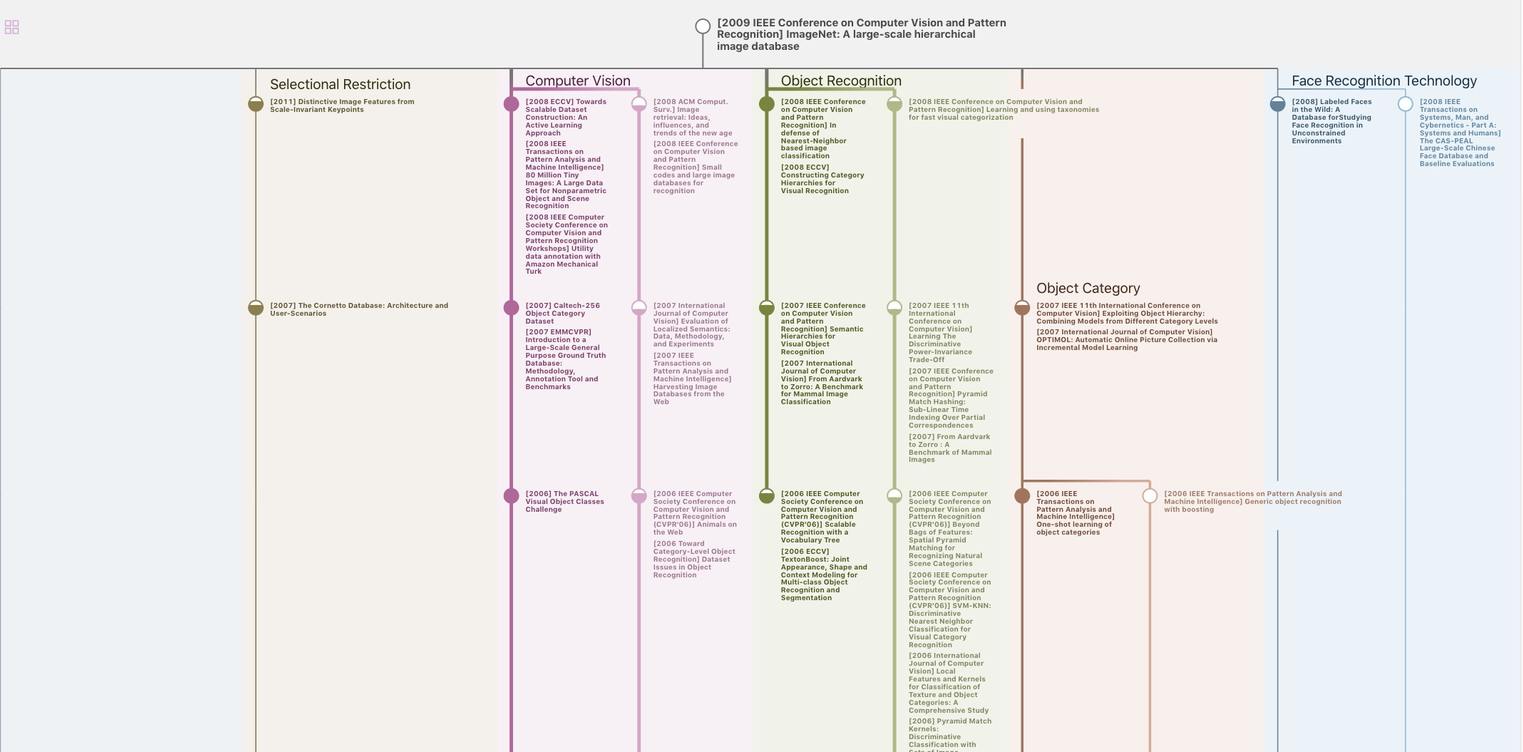
生成溯源树,研究论文发展脉络
Chat Paper
正在生成论文摘要