Evolutionary Optimization of Fuzzy Reinforcement Learning and Its Application to Time-Varying Tracking Control of Industrial Parallel Robotic Manipulators
IEEE transactions on industrial informatics(2023)
摘要
In this article, we contribute to the development of evolutionary optimization of fuzzy reinforcement learning and its application to time-varying tracking control for industrial parallel robotic manipulators. An improved evolutionary social spider optimization (SSO) paradigm with Cauchy mutation is presented to address the optimal fuzzy reinforcement learning problem. The SSO swarm intelligence incorporated with the fuzzy Q-learning strategy, called SSOFQ, is applied to the time-varying tracking control of parallel robotic Stewart manipulators with six degrees of freedom (DOFs). Having the derived kinematics and dynamics of six-DOF Stewart platforms, the SSOFQ is employed to synthesize an intelligent real-time tracking control scheme. More importantly, a custom experimental Stewart platform is constructed using hardware/software codesign and the system-on-a-programmable chip technique. Finally, the simulations, experimental results, and comparative works are provided to validate the efficacy and superiority of the presented SSOFQ control method.
更多查看译文
关键词
Optimization,Manipulators,Robots,Real-time systems,Q-learning,Service robots,Kinematics,Evolutionary optimization,fuzzy reinforcement learning,parallel manipulator,social spider optimization (SSO)
AI 理解论文
溯源树
样例
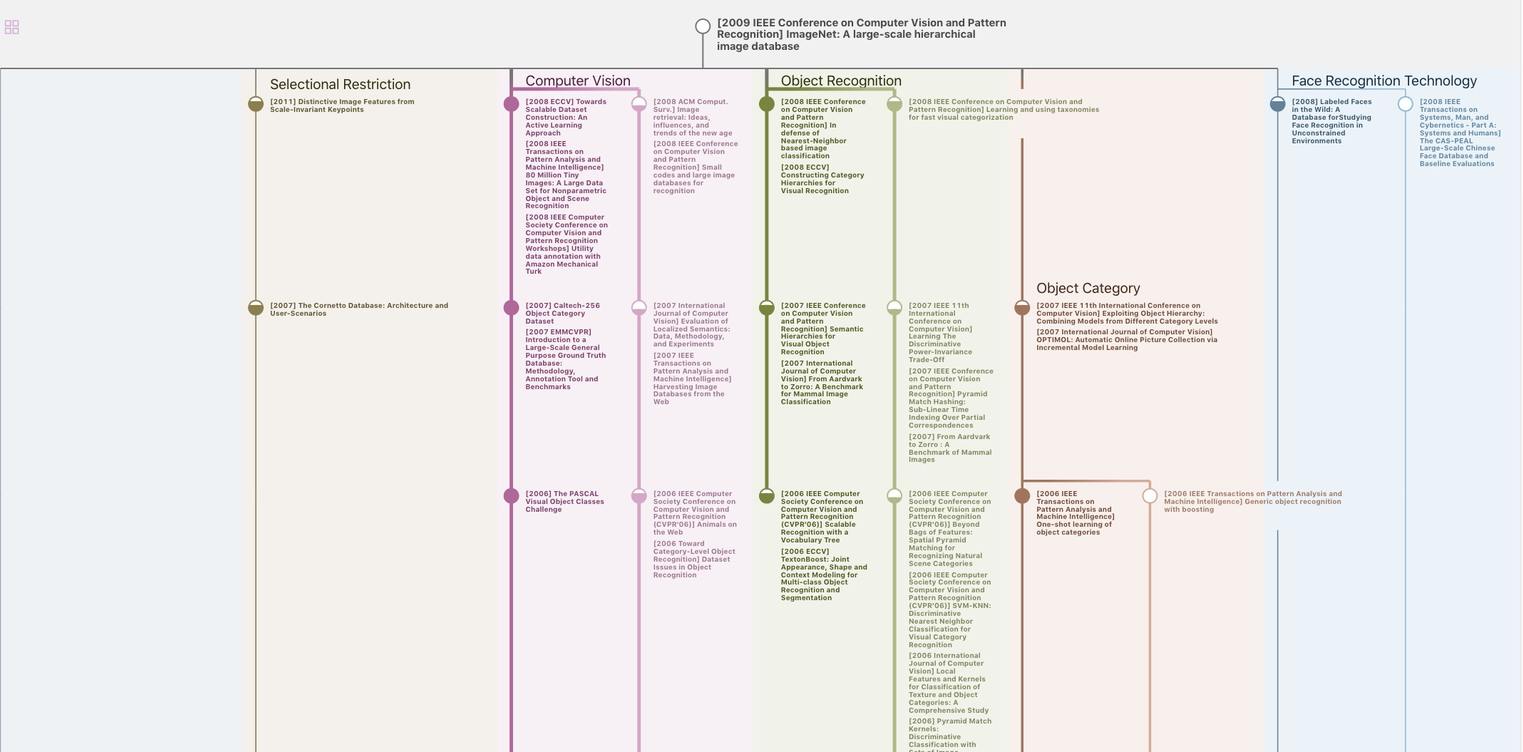
生成溯源树,研究论文发展脉络
Chat Paper
正在生成论文摘要