Can Machine Learning Unravel Unsuspected, Clinically Important Factors Predictive of Long-Term Mortality in Complex Coronary Artery Disease? A Call for ‘big Data’
European heart journal Digital health(2023)
关键词
Machine learning,Decision-making,PCI,CABG,SYNTAX,Long-term clinical outcomes
AI 理解论文
溯源树
样例
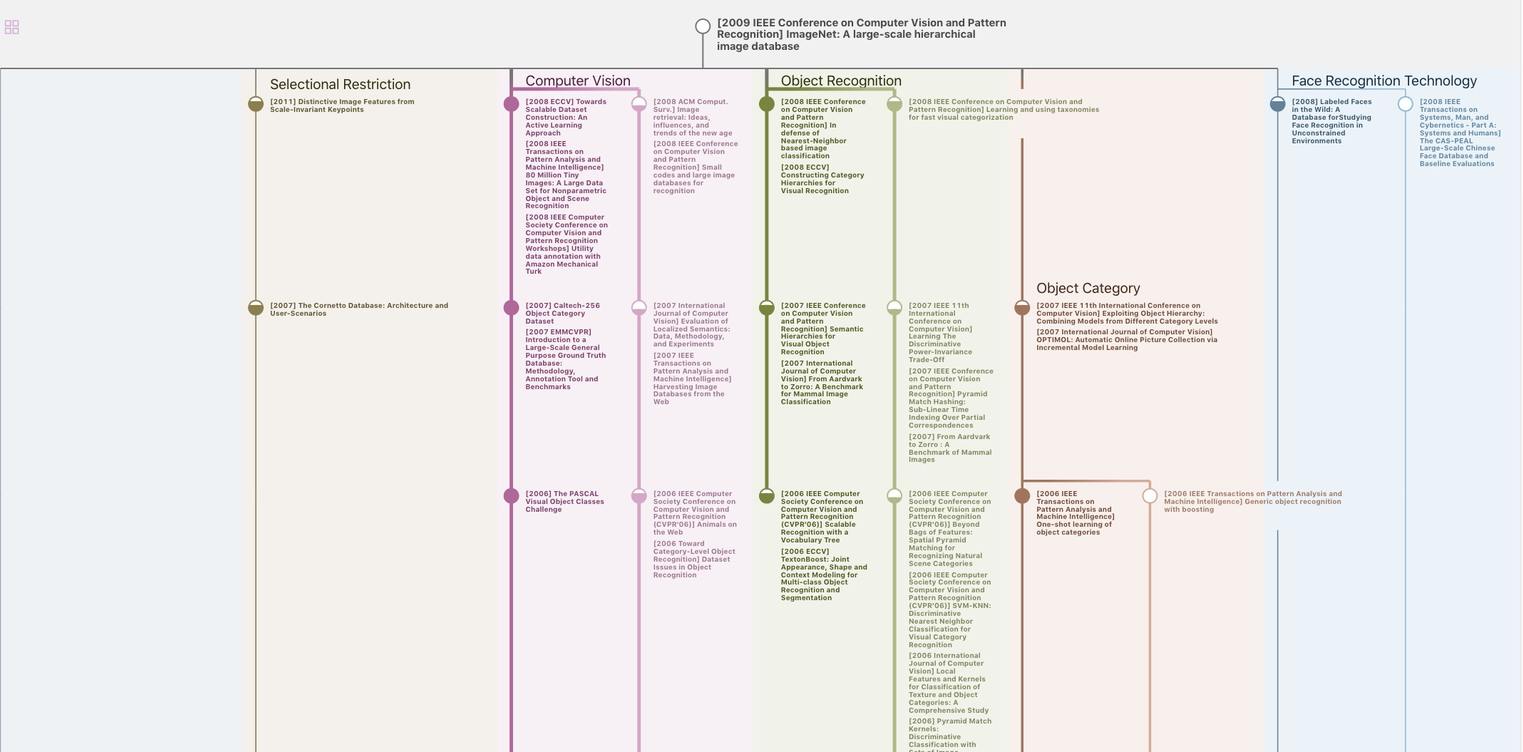
生成溯源树,研究论文发展脉络
Chat Paper
正在生成论文摘要