Control-Oriented Data-Driven and Physics-Based Modeling of Maximum Pressure Rise Rate in Reactivity Controlled Compression Ignition Engines
SAE INTERNATIONAL JOURNAL OF ENGINES(2023)
摘要
Reactivity controlled compression ignition (RCCI) is a viable low-temperature combustion (LTC) regime that can provide high indicated thermal efficiency and very low nitrogen oxides (NOx) and particulate matter (PM) emissions compared to the traditional diesel compression ignition (CI) mode [1]. The burn duration in RCCI engines is generally shorter compared to the burn duration for CI and spark-ignition (SI) combustion modes [2, 3]. This leads to a high pressure rise rate (PRR) and limits their operational range. It is important to predict the maximum pressure rise rate (MPRR) in RCCI engines and avoid excessive MPRRs to enable safe RCCI operation over a wide range of engine conditions. In this article, two control-oriented models are presented to predict the MPRR in an RCCI engine. The first approach includes a combined physical and empirical model that uses the first principle of thermodynamics to estimate the PRR inside the cylinder, and the second approach estimates MPRR through a machine learning method based on kernelized canonical correlation analysis (KCCA) and linear parameter-varying (LPV) methods. The KCCA-LPV approach proved to have higher prediction accuracy compared to physics-based modeling while requiring less amount of calibration. The KCCA-LPV approach could estimate MPRR with an average error of 47 kPa/CAD while the physics-based approach's average estimation error was 87 kPa/CAD. [GRAPHICS] .
更多查看译文
关键词
RCCI engine,Maximum pressure rise rate,Control-oriented model,Data-driven modeling,Physics-based modeling
AI 理解论文
溯源树
样例
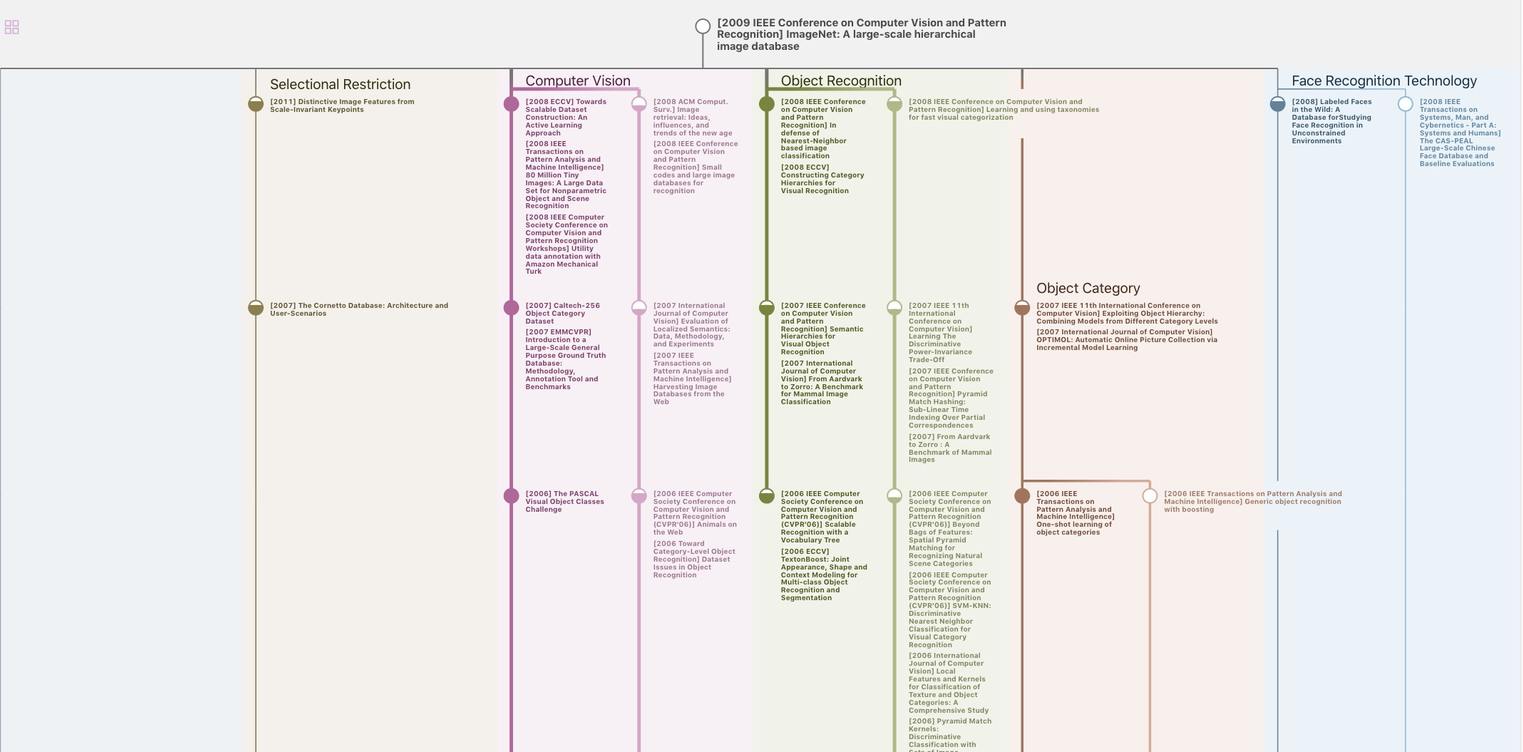
生成溯源树,研究论文发展脉络
Chat Paper
正在生成论文摘要