Revealing Feature Spaces Underlying Similarity Judgments of Natural Scenes in Individual Participants
Journal of Vision(2022)
Abstract
Understanding and responding to the dynamic environment around us requires continuous mapping of sensory information to representational space in our minds. This space can be characterised by a collection of features (e.g. location, animacy, and function) that drive perceived similarities between natural scenes. Resolving the structure of this internal multidimensional space is critical for understanding how we perceive, distinguish, and categorize natural scenes. Here, we collected similarity judgment data using a multiple arrangements task in eight participants for a set of 100 diverse natural scenes. To estimate underlying feature dimensions forming the basis of these similarity judgments, we trained small neural networks to learn object features. For each participant, we randomly initialised a 90-dimensional embedding layer, and used soft-max filtered dot products to predict the participant’s behavioral choices by identifying, for a given trio of stimuli, the stimulus most dissimilar from the other two. Loss estimated from these “odd-one-out” triplet predictions was backpropagated to update embedding weights. Cross-validated cosine distances computed from the learned embeddings were then used to construct model-generated representational dissimilarity matrices (RDMs). The resulting RDMs were significantly correlated with the same-subject RDMs computed from the observed similarity judgement data using classic iterative weighted averaging procedures³ (average Spearman’s rho=0.887; p<0.001), suggesting that the learned embeddings capture multidimensional similarity spaces at the individual participant level. The learned embeddings of each participant showed interpretable dimensions representing a variety of high and low order object features. Additionally, inter-subject comparison of model-generated RDMs showed increased similarity (average Spearman’s rho=0.29) between subjects in contrast to inter-subject comparison of RDMs generated by iterative weighted averaging (0.24). This suggests that our model is not only capable of reproducing similarity judgement data and capturing the underlying basis of these judgements at the individual level, but also able to better capture idiosyncratic relationships between individual participants.
MoreTranslated text
Key words
Interpersonal Synchrony,Object Recognition,Emotion Recognition,Face Perception,Cultural Learning
AI Read Science
Must-Reading Tree
Example
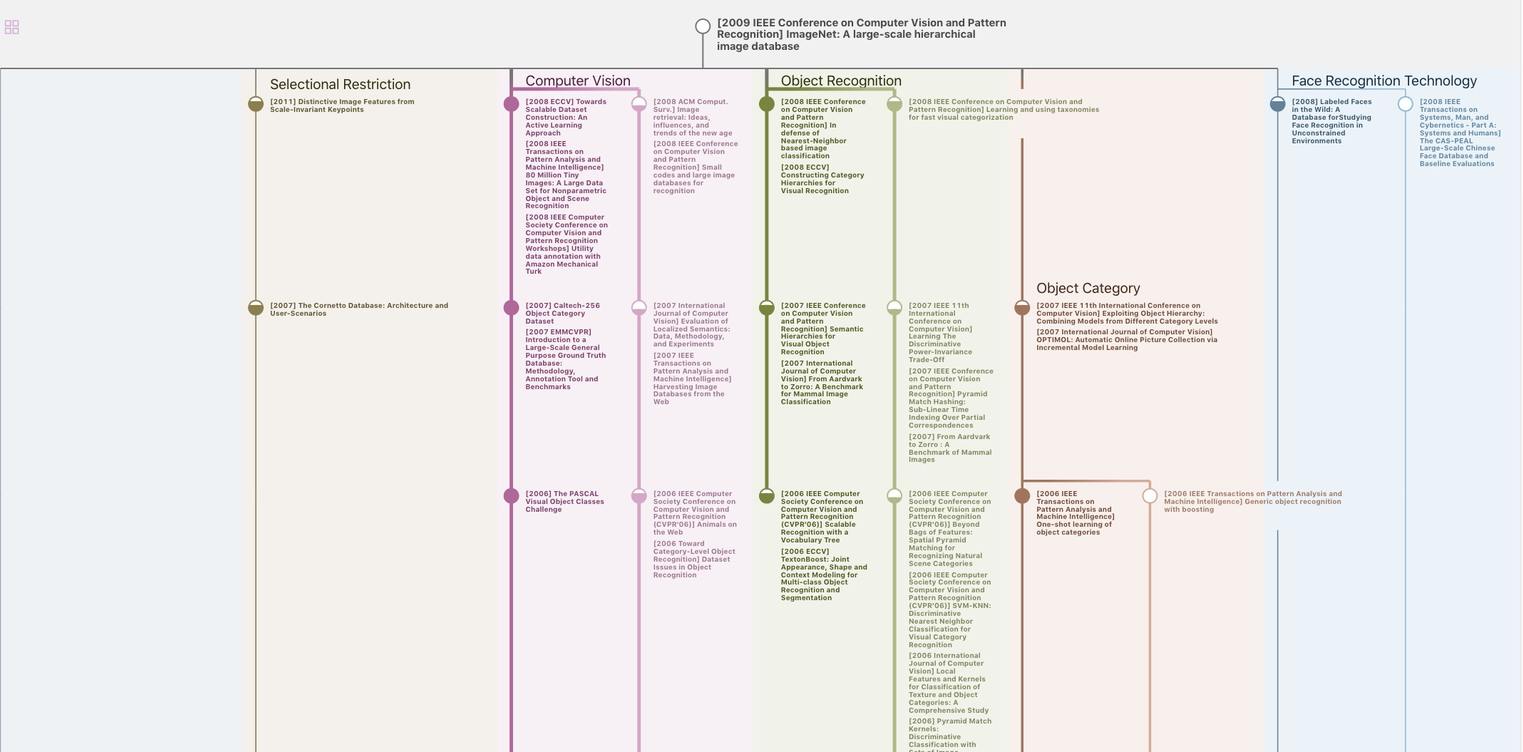
Generate MRT to find the research sequence of this paper
Chat Paper
Summary is being generated by the instructions you defined