Incentivizing High-Quality Content in Online Recommender Systems
CoRR(2023)
摘要
For content recommender systems such as TikTok and YouTube, the platform's decision algorithm shapes the incentives of content producers, including how much effort the content producers invest in the quality of their content. Many platforms employ online learning, which creates intertemporal incentives, since content produced today affects recommendations of future content. In this paper, we study the incentives arising from online learning, analyzing the quality of content produced at a Nash equilibrium. We show that classical online learning algorithms, such as Hedge and EXP3, unfortunately incentivize producers to create low-quality content. In particular, the quality of content is upper bounded in terms of the learning rate and approaches zero for typical learning rate schedules. Motivated by this negative result, we design a different learning algorithm -- based on punishing producers who create low-quality content -- that correctly incentivizes producers to create high-quality content. At a conceptual level, our work illustrates the unintended impact that a platform's learning algorithm can have on content quality and opens the door towards designing platform learning algorithms that incentivize the creation of high-quality content.
更多查看译文
关键词
content,high-quality
AI 理解论文
溯源树
样例
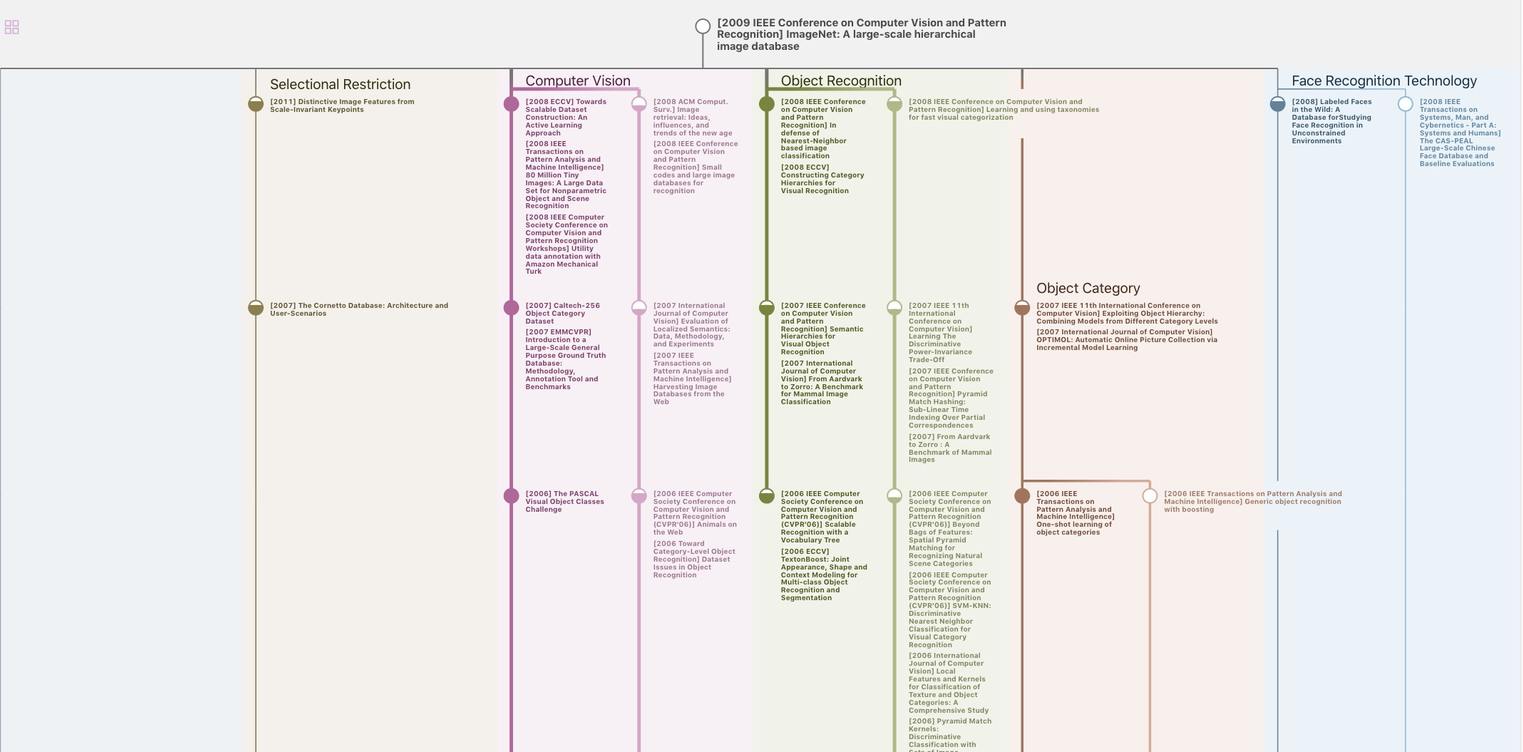
生成溯源树,研究论文发展脉络
Chat Paper
正在生成论文摘要