AFEX-Net: Adaptive Feature EXtraction CNN for Classifying CT Images
crossref(2023)
摘要
Deep convolutional neural networks (CNN) are favored methods widely used in medical image processing due to their assured shown performance. Recently, the emergence of new lung diseases and the possibility of early detection of their symptoms has attracted many researchers to classify diseases by training deep CNNs on lung CT images. The trained networks are expected to distinguish between lung indications in different diseases, especially at the early stages of them. With the hope of achieving this purpose, we proposed an efficient deep CNN called AFEX-Net with adaptive feature extraction layers that successfully extract distinguishing features and classify chest CT images. The efficiency of the proposed network has two aspects: it is a lightweight network with low number of parameters and fast training and it has adaptive pooling layers and adaptive activation functions to increase its level of compatibility to the input data. The proposed network has been evaluated on a dataset with more than 10K chest CT slices, while an efficient pre-processing method is developed to remove any bias from the images. Additionally, we evaluated the performance of the proposed model on the public COVID-CTset dataset to prove the generalisability of our model. The obtained results confirm the competence of the proposed network in confronting medical images, where prompt and accurate learning is required.
更多查看译文
AI 理解论文
溯源树
样例
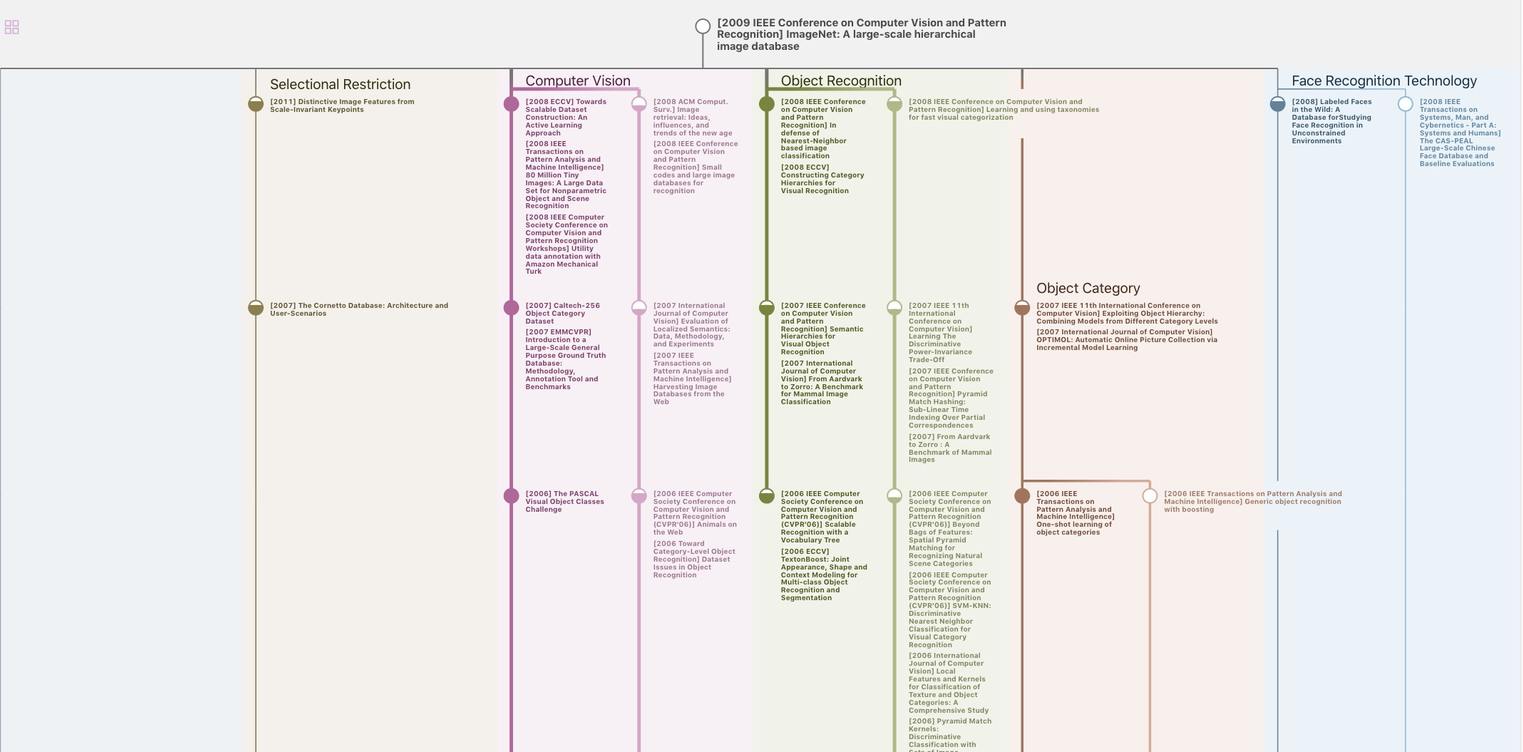
生成溯源树,研究论文发展脉络
Chat Paper
正在生成论文摘要