On the Dynamics of Learning Time-Aware Behavior with Recurrent Neural Networks
CoRR(2023)
摘要
Recurrent Neural Networks (RNNs) have shown great success in modeling time-dependent patterns, but there is limited research on their learned representations of latent temporal features and the emergence of these representations during training. To address this gap, we use timed automata (TA) to introduce a family of supervised learning tasks modeling behavior dependent on hidden temporal variables whose complexity is directly controllable. Building upon past studies from the perspective of dynamical systems, we train RNNs to emulate temporal flipflops, a new collection of TA that emphasizes the need for time-awareness over long-term memory. We find that these RNNs learn in phases: they quickly perfect any time-independent behavior, but they initially struggle to discover the hidden time-dependent features. In the case of periodic "time-of-day" aware automata, we show that the RNNs learn to switch between periodic orbits that encode time modulo the period of the transition rules. We subsequently apply fixed point stability analysis to monitor changes in the RNN dynamics during training, and we observe that the learning phases are separated by a bifurcation from which the periodic behavior emerges. In this way, we demonstrate how dynamical systems theory can provide insights into not only the learned representations of these models, but also the dynamics of the learning process itself. We argue that this style of analysis may provide insights into the training pathologies of recurrent architectures in contexts outside of time-awareness.
更多查看译文
关键词
recurrent neural
AI 理解论文
溯源树
样例
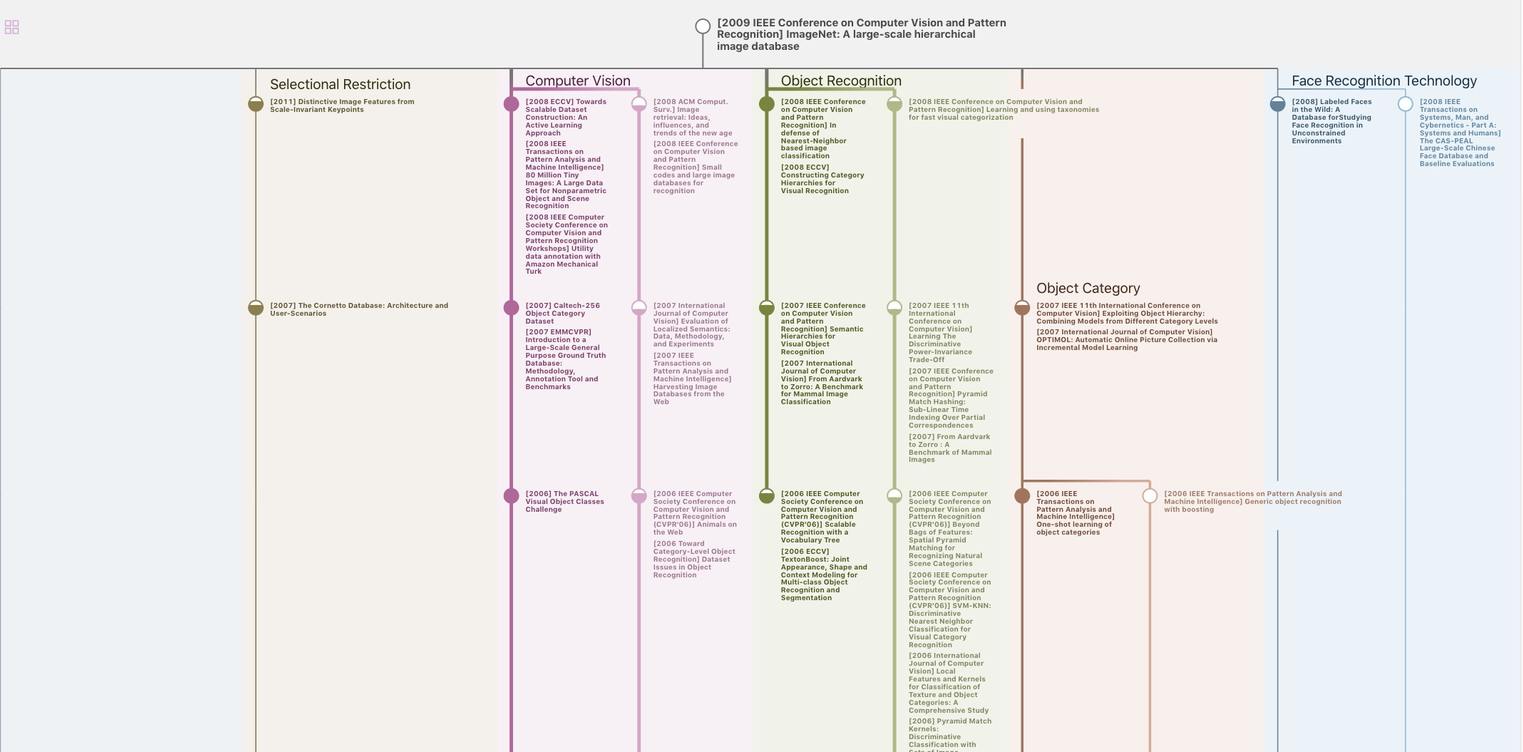
生成溯源树,研究论文发展脉络
Chat Paper
正在生成论文摘要