Public Transit Demand Prediction During Highly Dynamic Conditions: A Meta-Analysis of State-of-the-Art Models and Open-Source Benchmarking Infrastructure
CoRR(2023)
摘要
Real-time demand prediction is a critical input for dynamic bus routing. While many researchers have developed numerous complex methods to predict short-term transit demand, the applications have been limited to short, stable time frames and a few stations. How these methods perform in highly dynamic environments has not been studied, nor has their performance been systematically compared. We built an open-source infrastructure with five common methodologies, including econometric and deep learning approaches, and assessed their performance under stable and highly dynamic conditions. We used a time series from smartcard data to predict demand for the following day for the BRT system in Bogota, Colombia. The dynamic conditions in the time series include a month-long protest and the COVID-19 pandemic. Both conditions triggered drastic shifts in demand. The results reveal that most tested models perform similarly in stable conditions, with MAAPE varying from 0.08 to 0.12. The benchmark demonstrated that all models performed significantly worse in both dynamic conditions compared to the stable conditions. In the month-long protest, the increased MAAPE ranged from 0.14 to 0.24. Similarly, during the COVID-19 pandemic, the increased MAAPE ranged from 0.12 to 0.82. Notably, in the COVID-19 pandemic condition, an LSTM model with adaptive training and a multi-output design outperformed other models, adapting faster to disruptions. The prediction error stabilized within approximately 1.5 months, whereas other models continued to exhibit higher error rates even a year after the start of the pandemic. The aim of this open-source codebase infrastructure is to lower the barrier for other researchers to replicate and reproduce models, facilitate a collective effort within the research community to improve the benchmarking process and accelerate the advancement of short-term ridership prediction models.
更多查看译文
关键词
demand,prediction,benchmarking
AI 理解论文
溯源树
样例
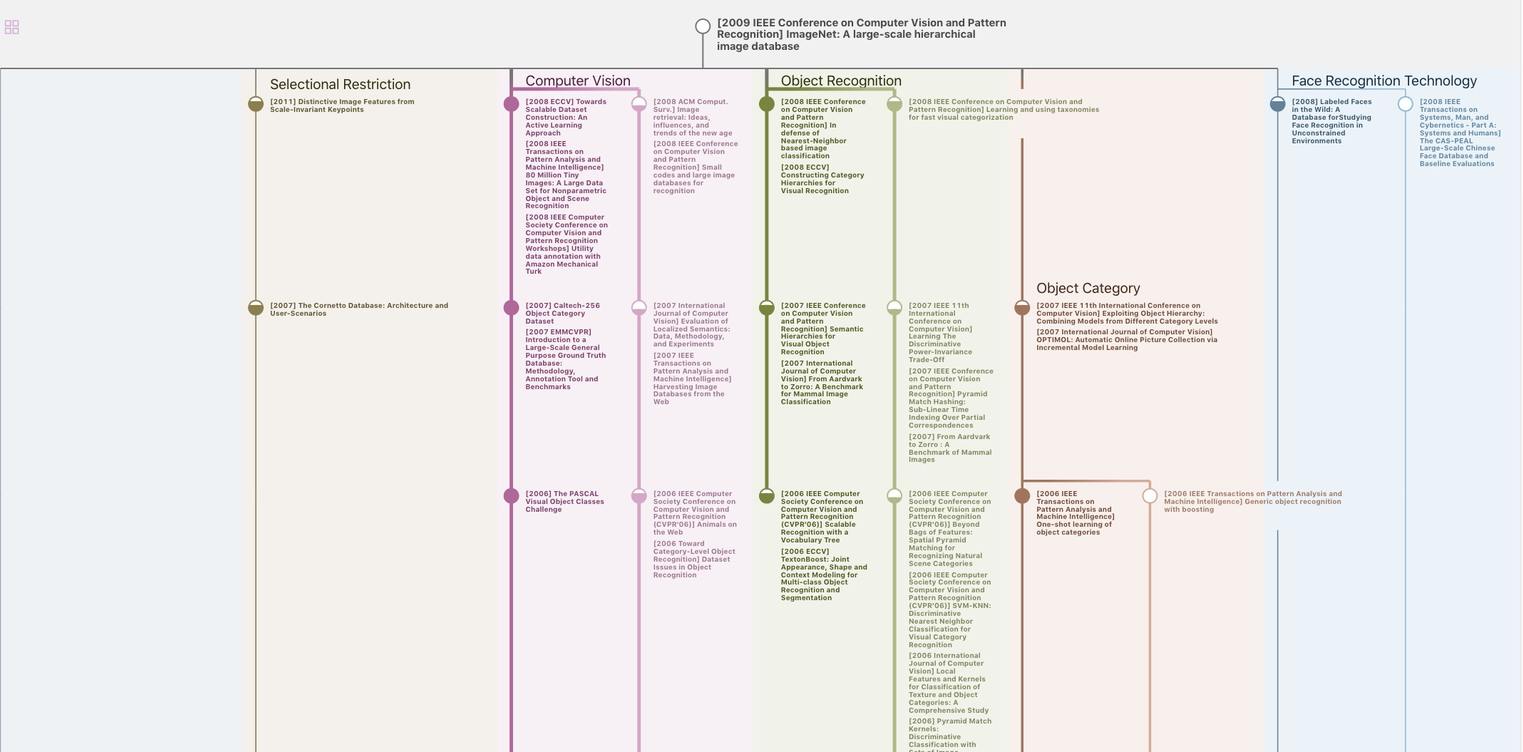
生成溯源树,研究论文发展脉络
Chat Paper
正在生成论文摘要