Advancing Italian Biomedical Information Extraction with Transformers-based Models: Methodological Insights and Multicenter Practical Application
JOURNAL OF BIOMEDICAL INFORMATICS(2023)
IRCCS Ist Ctr San Giovanni Dio Fatebenefratelli | Univ Pavia | IRCCS Ist Auxol Italiano | IRCCS Ist Clin Sci Maugeri
Abstract
The introduction of computerized medical records in hospitals has reduced burdensome activities like manual writing and information fetching. However, the data contained in medical records are still far underutilized, primarily because extracting data from unstructured textual medical records takes time and effort. Information Extraction, a subfield of Natural Language Processing, can help clinical practitioners overcome this limitation by using automated text-mining pipelines. In this work, we created the first Italian neuropsychiatric Named Entity Recognition dataset, PsyNIT, and used it to develop a Transformers-based model. Moreover, we collected and leveraged three external independent datasets to implement an effective multicenter model, with overall F1-score 84.77%, Precision 83.16%, Recall 86.44%. The lessons learned are: (i) the crucial role of a consistent annotation process and (ii) a fine-tuning strategy that combines classical methods with a "low-resource" approach. This allowed us to establish methodological guidelines that pave the way for Natural Language Processing studies in less-resourced languages.
MoreTranslated text
Key words
Natural language processing,Deep learning,Biomedical text mining,Language model,Transformer
PDF
View via Publisher
AI Read Science
Must-Reading Tree
Example
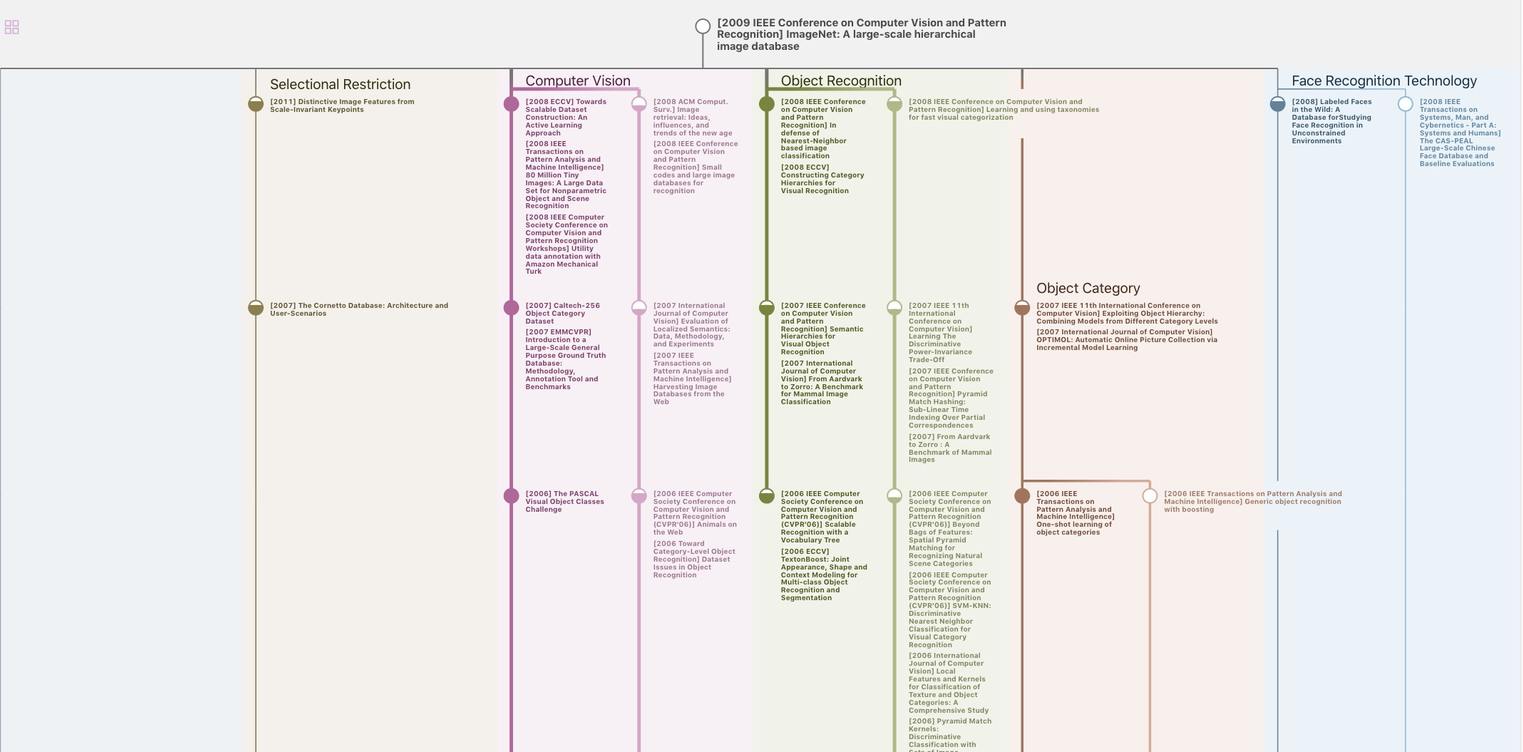
Generate MRT to find the research sequence of this paper
Related Papers
Data Disclaimer
The page data are from open Internet sources, cooperative publishers and automatic analysis results through AI technology. We do not make any commitments and guarantees for the validity, accuracy, correctness, reliability, completeness and timeliness of the page data. If you have any questions, please contact us by email: report@aminer.cn
Chat Paper
去 AI 文献库 对话