Bearing Surface Defect Detection Based on Improved Convolutional Neural Network.
Mathematical Biosciences and Engineering(2023)
摘要
This paper addresses the issue of artificial visual inspection being overly reliant on subjective experience and the difficulty for the human eye to accurately identify dense and non-significant defects. To solve this problem, we have implemented an automatic object detection algorithm based on an improved version of YOLOv5.First, we use the K-means++ clustering algorithm to automatically calculate the Anchor of the model to reduce the effect of the close location of the initial clustering centers on the clustering of the sample data.Second, we add the Coordinate Attention (CA) attention mechanism to the model to allow the model to better capture and understand important features in the images. Then, we add a new detection layer with a downsampling multiplier of 4 to the Neck network to improve the precision of the model. Finally, we use the lightweight network MobileNetV3 instead of YOLOv5's backbone network to reduce the model detection time overhead.Our model achieves 85.87% mAP, which is 6.44% better than the YOLOv5 network, and the detection time for a single image is only 54ms, which is 50% faster than the YOLOv5 network. After testing, we have proven that our proposed algorithm can quickly and accurately detect the condition of bearing appearance defects, improving detection efficiency and reducing costs.
更多查看译文
关键词
target detection,defect detection,attention mechanism,lightweight network
AI 理解论文
溯源树
样例
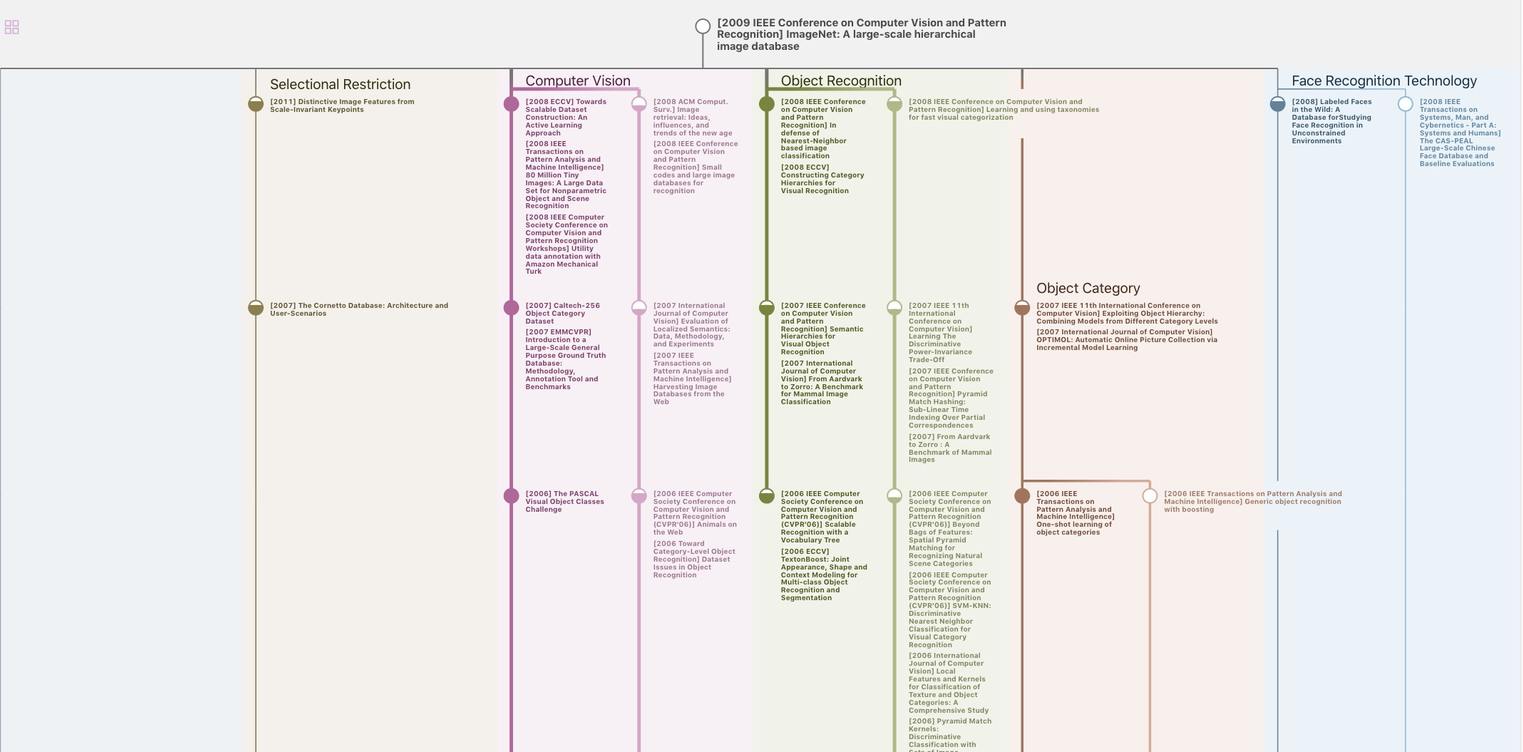
生成溯源树,研究论文发展脉络
Chat Paper
正在生成论文摘要