CIForm as a Transformer-based model for cell-type annotation of large-scale single-cell RNA-seq data
BRIEFINGS IN BIOINFORMATICS(2023)
摘要
Single-cell omics technologies have made it possible to analyze the individual cells within a biological sample, providing a more detailed understanding of biological systems. Accurately determining the cell type of each cell is a crucial goal in single-cell RNA-seq (scRNA-seq) analysis. Apart from overcoming the batch effects arising from various factors, single-cell annotation methods also face the challenge of effectively processing large-scale datasets. With the availability of an increase in the scRNA-seq datasets, integrating multiple datasets and addressing batch effects originating from diverse sources are also challenges in cell-type annotation. In this work, to overcome the challenges, we developed a supervised method called CIForm based on the Transformer for cell-type annotation of large-scale scRNA-seq data. To assess the effectiveness and robustness of CIForm, we have compared it with some leading tools on benchmark datasets. Through the systematic comparisons under various cell-type annotation scenarios, we exhibit that the effectiveness of CIForm is particularly pronounced in cell-type annotation. The source code and data are available at .
更多查看译文
关键词
cell-type annotation,deep learning,Transformer,scRNA-seq,large-scale dataset
AI 理解论文
溯源树
样例
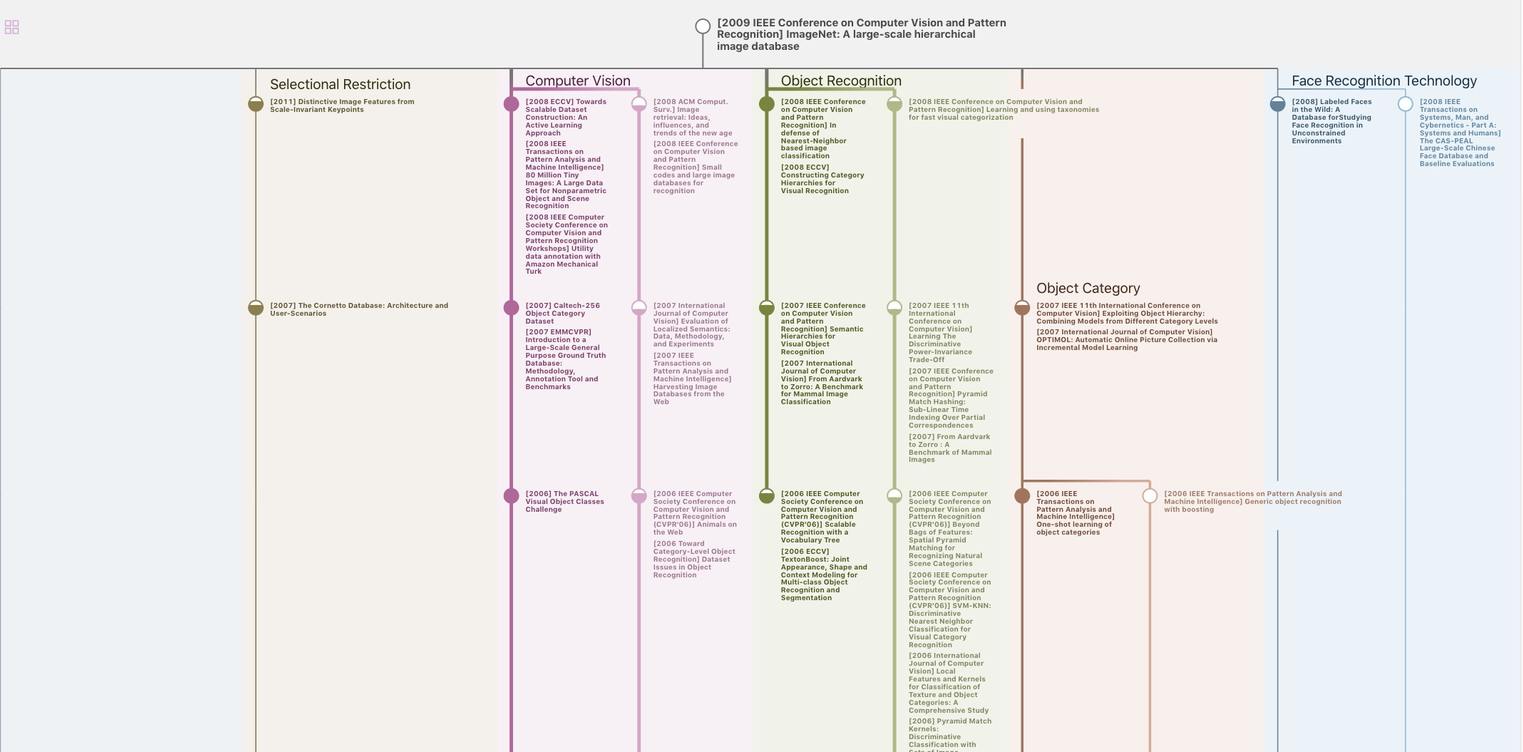
生成溯源树,研究论文发展脉络
Chat Paper
正在生成论文摘要