Multi-activation Stochastic Configuration Network
2023 4th International Conference on Computer Engineering and Application (ICCEA)(2023)
Abstract
Stochastic configuration network (SCN) utilize a unique supervisory mechanism to construct hidden layer nodes to ensure the universal approximation capability, constituting a neural network with advantages such as fast convergence and guaranteed generalization error. However, the disadvantage of the original SCN is that the single activation function used could cause premature overfitting of the model as the number of hidden layer nodes continues to increase, in order to ensure the model perform better. To address the issue, a kind of improvement strategy aiming to the constructure of the hidden layer of original SCN, a multi-activation stochastic configuration network (MA-SCN) architecture is proposed in this paper. That means, the selection of the activation functions deeply dependent on data, namely, the increase progressively progress of hidden node by selecting the activation function with the best performance, so the better generalization capability of the model could obtain with compacter structure of the hidden layer. Simulation results demonstrate that the strategy of multiple activation function selection by merit can improve the accuracy of the SCN model in solving regression problems while reducing computational effort. The proposed model has been used in real industrial data cases and has fully demonstrated its feasibility and effectiveness.
MoreTranslated text
Key words
Stochastic configuration network,multi-activation strategy,big data modelling,incremental learning
AI Read Science
Must-Reading Tree
Example
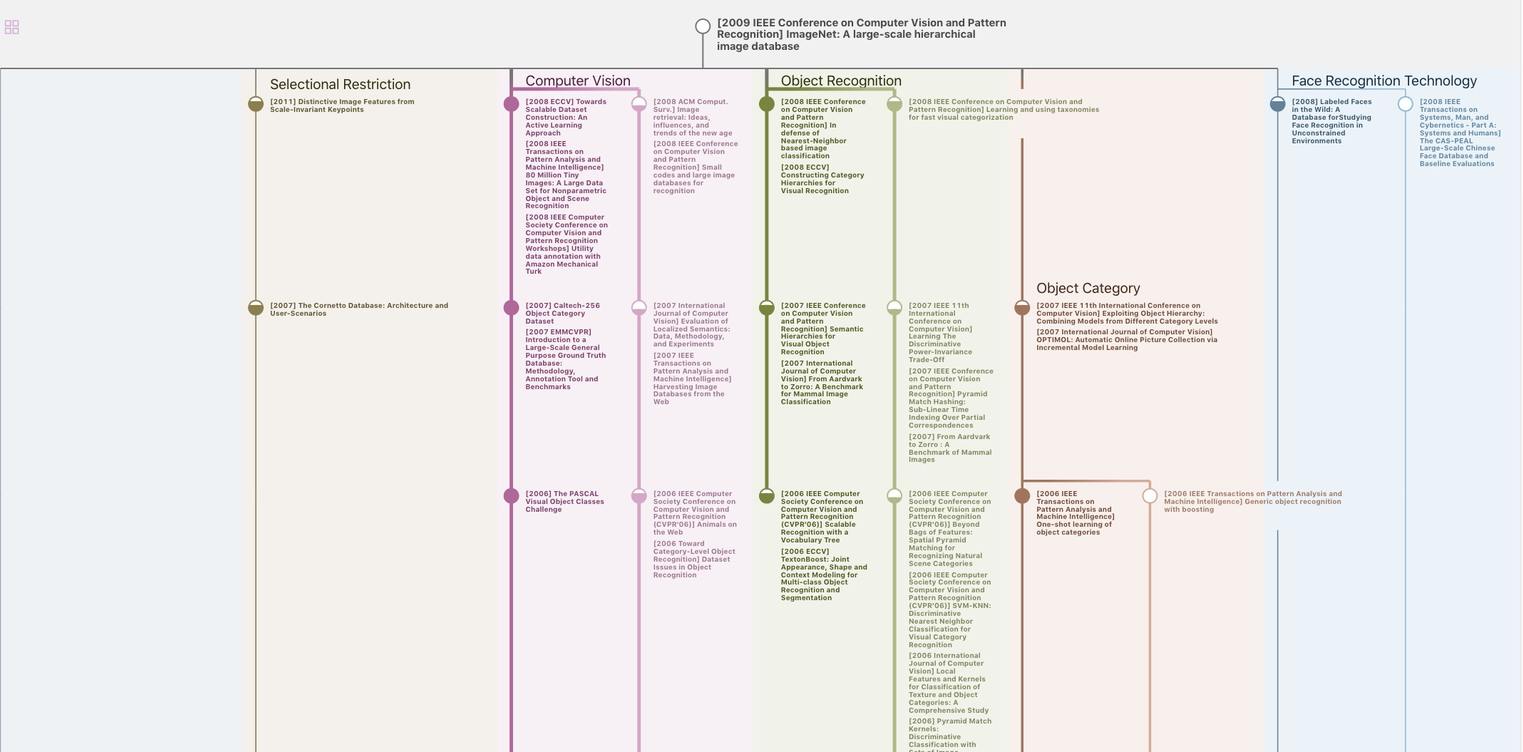
Generate MRT to find the research sequence of this paper
Chat Paper
Summary is being generated by the instructions you defined