Fingerprint-Enhanced Graph Attention Network (FinGAT) Model for Antibiotic Discovery.
J. Chem. Inf. Model.(2023)
摘要
Artificial Intelligence (AI) techniques are of great potential to fundamentally change antibiotic discovery industries. Efficient and effective molecular featurization is key to all highly accurate learning models for antibiotic discovery. In this paper, we propose a fingerprint-enhanced graph attention network (FinGAT) model by the combination of sequence-based 2D fingerprints and structure-based graph representation. In our feature learning process, sequence information is transformed into a fingerprint vector, and structural information is encoded through a GAT module into another vector. These two vectors are concatenated and input into a multilayer perceptron (MLP) for antibiotic activity classification. Our model is extensively tested and compared with existing models. It has been found that our FinGAT can outperform various state-of-the-art GNN models in antibiotic discovery.
更多查看译文
关键词
antibiotic discovery,graph attention network,fingerprint-enhanced
AI 理解论文
溯源树
样例
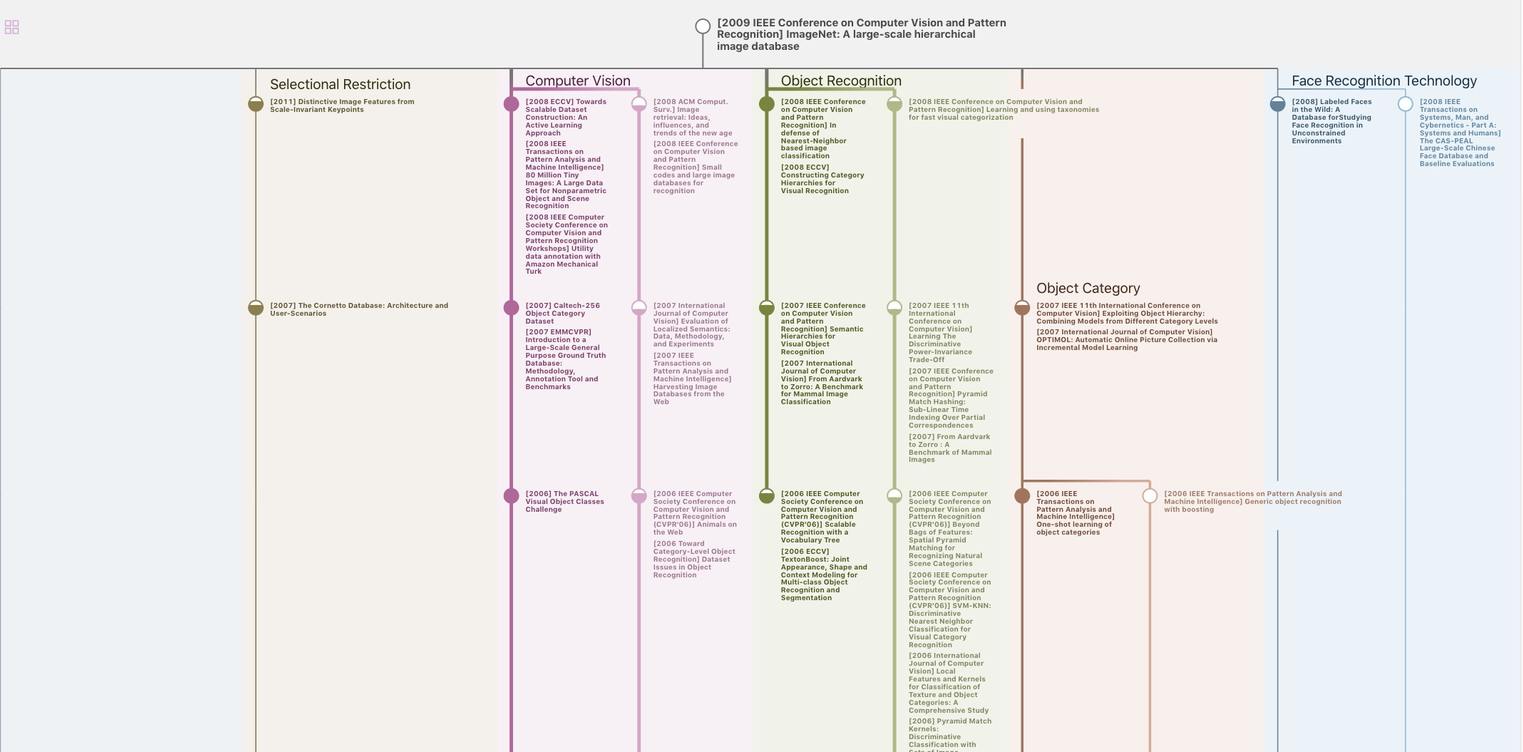
生成溯源树,研究论文发展脉络
Chat Paper
正在生成论文摘要