Microstructure quality control of steels using deep learning
FRONTIERS IN MATERIALS(2023)
摘要
In quality control, microstructures are investigated rigorously to ensure structural integrity, exclude the presence of critical volume defects, and validate the formation of the target microstructure. For quenched, hierarchically-structured steels, the morphology of the bainitic and martensitic microstructures are of major concern to guarantee the reliability of the material under service conditions. Therefore, industries conduct small sample-size inspections of materials cross-sections through metallographers to validate the needle morphology of such microstructures. We demonstrate round-robin test results revealing that this visual grading is afflicted by pronounced subjectivity despite the thorough training of personnel. Instead, we propose a deep learning image classification approach that distinguishes steels based on their microstructure type and classifies their needle length alluding to the ISO 643 grain size assessment standard. This classification approach facilitates the reliable, objective, and automated classification of hierarchically structured steels. Specifically, an accuracy of 96% and roughly 91% is attained for the distinction of martensite/bainite subtypes and needle length, respectively. This is achieved on an image dataset that contains significant variance and labeling noise as it is acquired over more than 10 years from multiple plants, alloys, etchant applications, and light optical microscopes by many metallographers (raters). Interpretability analysis gives insights into the decision-making of these models and allows for estimating their generalization capability.
更多查看译文
关键词
quality control,microstructure,grain size,steel,martensite,bainite,deep learning
AI 理解论文
溯源树
样例
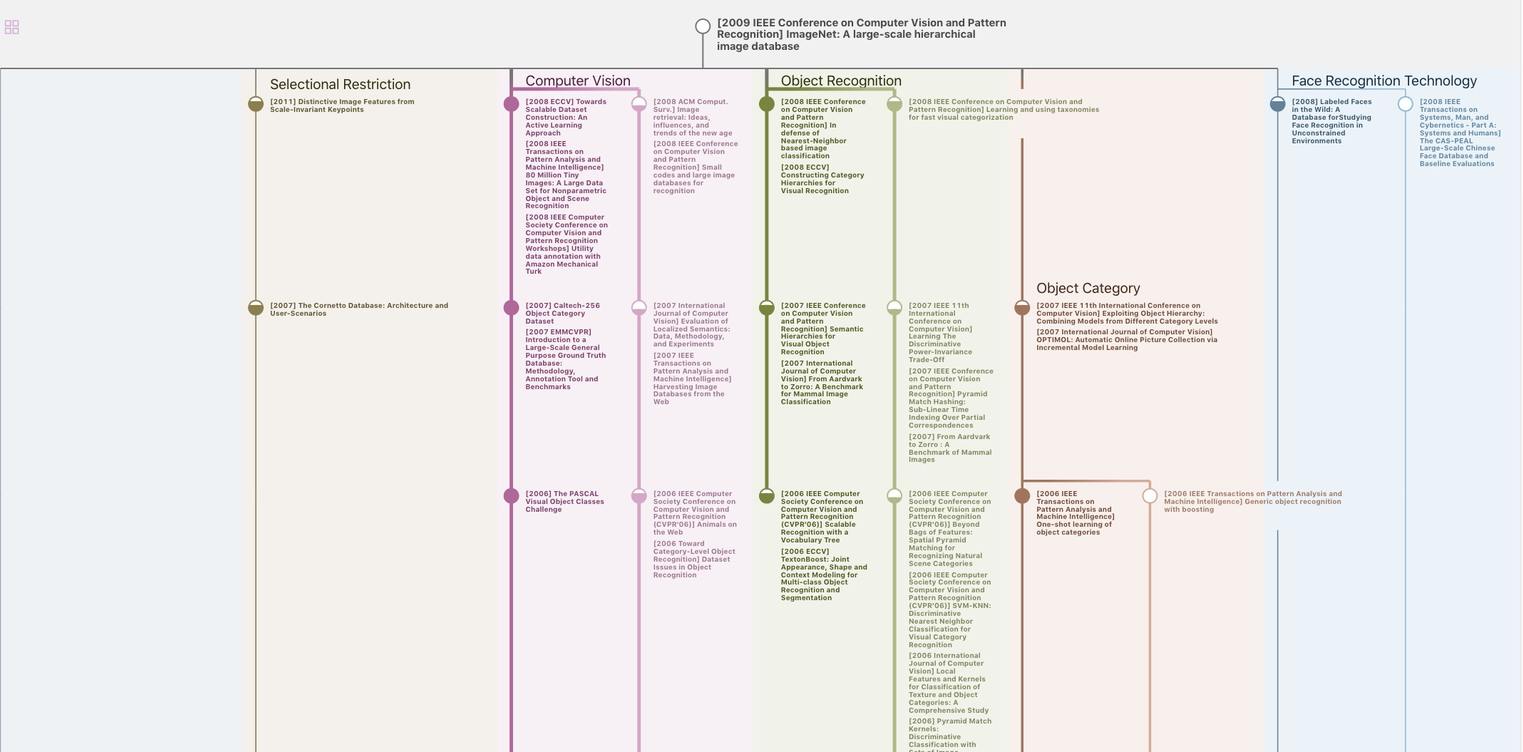
生成溯源树,研究论文发展脉络
Chat Paper
正在生成论文摘要