Effective Structured Prompting by Meta-Learning and Representative Verbalizer
CoRR(2023)
摘要
Prompt tuning for pre-trained masked language models (MLM) has shownpromising performance in natural language processing tasks with few labeledexamples. It tunes a prompt for the downstream task, and a verbalizer is usedto bridge the predicted token and label prediction. Due to the limited trainingdata, prompt initialization is crucial for prompt tuning. Recently,MetaPrompting (Hou et al., 2022) uses meta-learning to learn a sharedinitialization for all task-specific prompts. However, a single initializationis insufficient to obtain good prompts for all tasks and samples when the tasksare complex. Moreover, MetaPrompting requires tuning the whole MLM, causing aheavy burden on computation and memory as the MLM is usually large. To addressthese issues, we use a prompt pool to extract more task knowledge and constructinstance-dependent prompts via attention. We further propose a novel softverbalizer (RepVerb) which constructs label embedding from feature embeddingsdirectly. Combining meta-learning the prompt pool and RepVerb, we proposeMetaPrompter for effective structured prompting. MetaPrompter isparameter-efficient as only the pool is required to be tuned. Experimentalresults demonstrate that MetaPrompter performs better than the recentstate-of-the-arts and RepVerb outperforms existing soft verbalizers.
更多查看译文
AI 理解论文
溯源树
样例
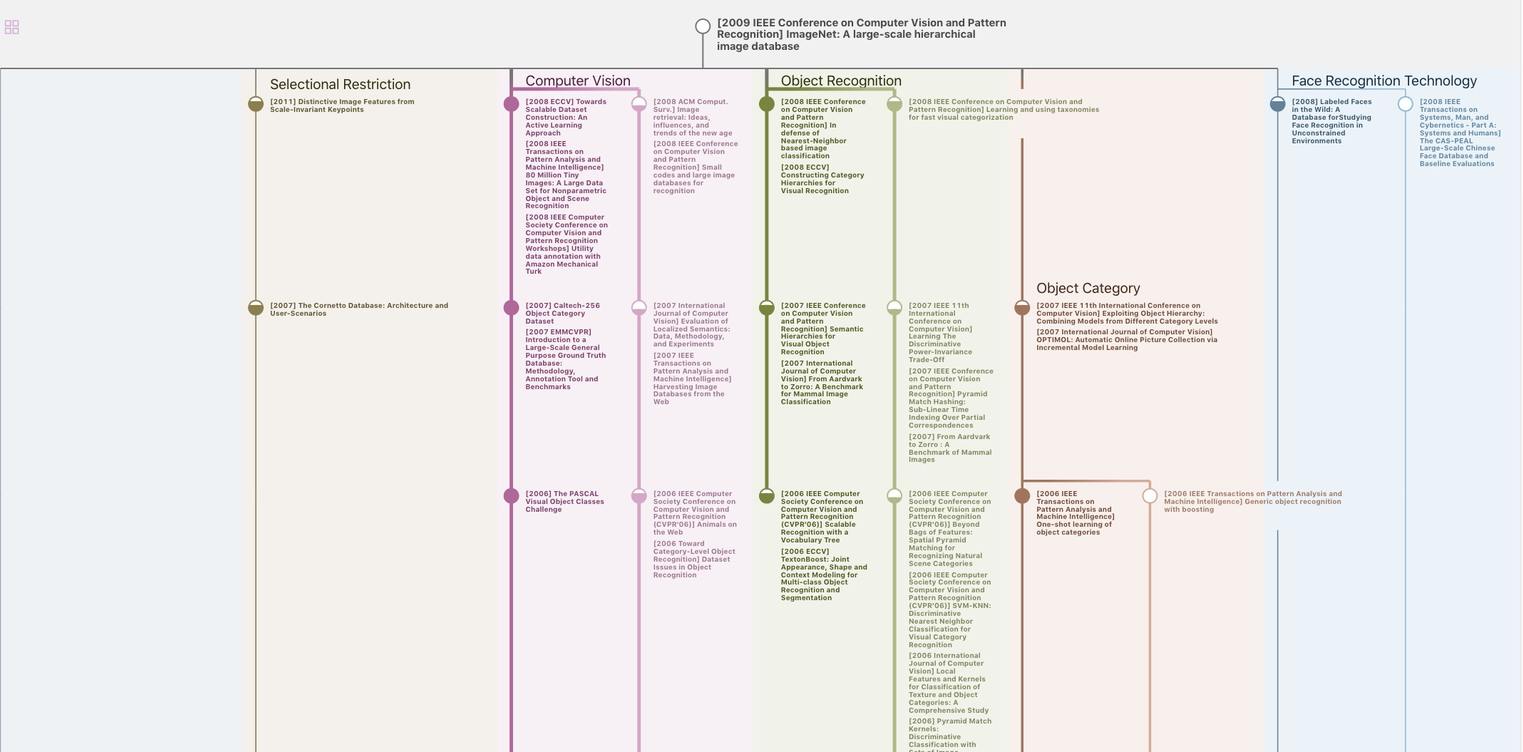
生成溯源树,研究论文发展脉络
Chat Paper
正在生成论文摘要