Automated body organ segmentation and volumetry for 3D motion-corrected T2-weighted fetal body MRI: a pilot pipeline
medRxiv : the preprint server for health sciences(2023)
摘要
Structural fetal body MRI provides true 3D information required for volumetry of fetal organs. However, current clinical and research practice primarily relies on manual slice-wise segmentation of raw T2-weighted stacks, which is time consuming, subject to inter- and intra-observer bias and affected by motion-corruption. Furthermore, there are no existing standard guidelines defining a universal approach to parcellation of fetal organs. This work produces the first parcellation protocol of the fetal body organs for motion-corrected 3D fetal MRI. It includes 10 organ ROIs relevant to fetal quantitative volumetry studies. The protocol was used as a basis for training of a neural network for automated multi-label segmentation based on manual segmentations and semi-supervised training. The deep learning pipeline showed robust performance for different gestational ages. This solution minimises the need for manual editing and significantly reduces time in comparison to conventional manual segmentation. The general feasibility of the proposed pipeline was assessed by analysis of organ growth charts created from automated parcellations of 91 normal control 3T MRI datasets that showed expected increase in volumetry during 22-38 weeks gestational age range. In addition, the results of comparison between 60 normal and 12 fetal growth restriction datasets revealed significant differences in organ volumes.
### Competing Interest Statement
The authors have declared no competing interest.
### Funding Statement
This work was supported by: NIHR Advanced Fellowship awarded to Lisa Story [NIHR30166]; MRC Confidence in concept [MC\_PC\_19041]; the NIH Human Placenta Project grant [1U01HD087202-01]; the Wellcome/ EPSRC Centre for Medical Engineering at Kings' College London [WT 203148/Z/16/Z]; the NIHR Clinical Research Facility (CRF) at Guy's and St Thomas'; and by the National Institute for Health Research Biomedical Research Centre based at Guy's and St Thomas' NHS Foundation Trust and King's College London.
### Author Declarations
I confirm all relevant ethical guidelines have been followed, and any necessary IRB and/or ethics committee approvals have been obtained.
Yes
The details of the IRB/oversight body that provided approval or exemption for the research described are given below:
King's College London gave ethical approval for this work. The Placental Imaging Project - PiP: REC 16/LO/1573; Individualised Risk prediction of adverse neonatal outcome in pregnancies that deliver preterm using advanced MRI techniques and machine learning study: REC 21/SS/0082
I confirm that all necessary patient/participant consent has been obtained and the appropriate institutional forms have been archived, and that any patient/participant/sample identifiers included were not known to anyone (e.g., hospital staff, patients or participants themselves) outside the research group so cannot be used to identify individuals.
Yes
I understand that all clinical trials and any other prospective interventional studies must be registered with an ICMJE-approved registry, such as ClinicalTrials.gov. I confirm that any such study reported in the manuscript has been registered and the trial registration ID is provided (note: if posting a prospective study registered retrospectively, please provide a statement in the trial ID field explaining why the study was not registered in advance).
Yes
I have followed all appropriate research reporting guidelines, such as any relevant EQUATOR Network research reporting checklist(s) and other pertinent material, if applicable.
Yes
The 3D fetal body MRI atlas and the segmentation pipeline the will be publicly available online after publication of the article. The individual fetal MRI datasets used in this work are not publicly available due to privacy or ethical restrictions due to privacy or ethical restrictions.
[https://gin.g-node.org/SVRTK/fetal\_mri\_atlases][1]
[1]: https://gin.g-node.org/SVRTK/fetal_mri_atlases
更多查看译文
关键词
fetal body mri,body organ segmentation,volumetry,3d,motion-corrected
AI 理解论文
溯源树
样例
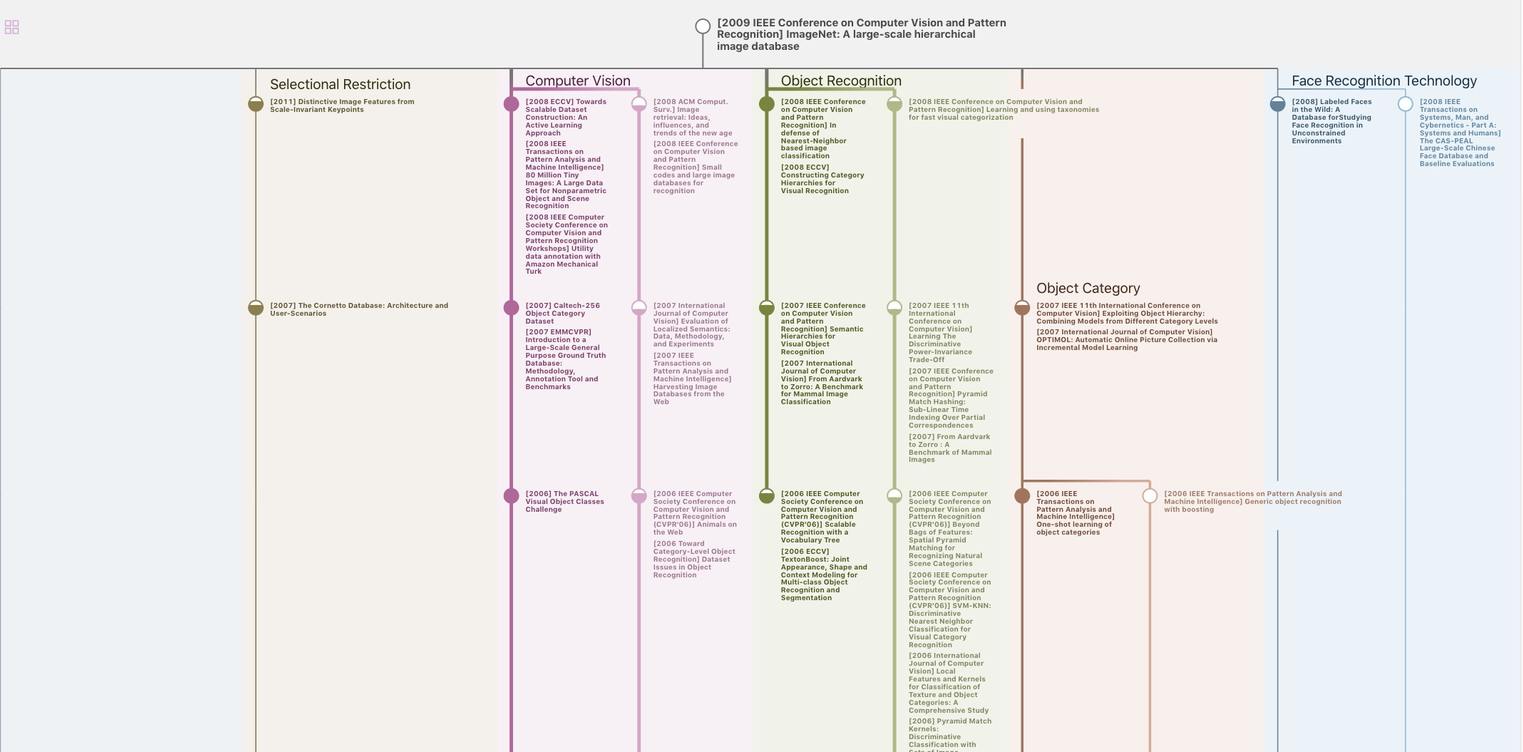
生成溯源树,研究论文发展脉络
Chat Paper
正在生成论文摘要