Hardware-aware Training Techniques for Improving Robustness of Ex-Situ Neural Network Transfer onto Passive TiO2 ReRAM Crossbars
CoRR(2023)
摘要
Passive resistive random access memory (ReRAM) crossbar arrays, a promising emerging technology used for analog matrix-vector multiplications, are far superior to their active (1T1R) counterparts in terms of the integration density. However, current transfers of neural network weights into the conductance state of the memory devices in the crossbar architecture are accompanied by significant losses in precision due to hardware variabilities such as sneak path currents, biasing scheme effects and conductance tuning imprecision. In this work, training approaches that adapt techniques such as dropout, the reparametrization trick and regularization to TiO2 crossbar variabilities are proposed in order to generate models that are better adapted to their hardware transfers. The viability of this approach is demonstrated by comparing the outputs and precision of the proposed hardware-aware network with those of a regular fully connected network over a few thousand weight transfers using the half moons dataset in a simulation based on experimental data. For the neural network trained using the proposed hardware-aware method, 79.5% of the test set's data points can be classified with an accuracy of 95% or higher, while only 18.5% of the test set's data points can be classified with this accuracy by the regularly trained neural network.
更多查看译文
关键词
neural network transfer,passive tio2,hardware-aware,ex-situ
AI 理解论文
溯源树
样例
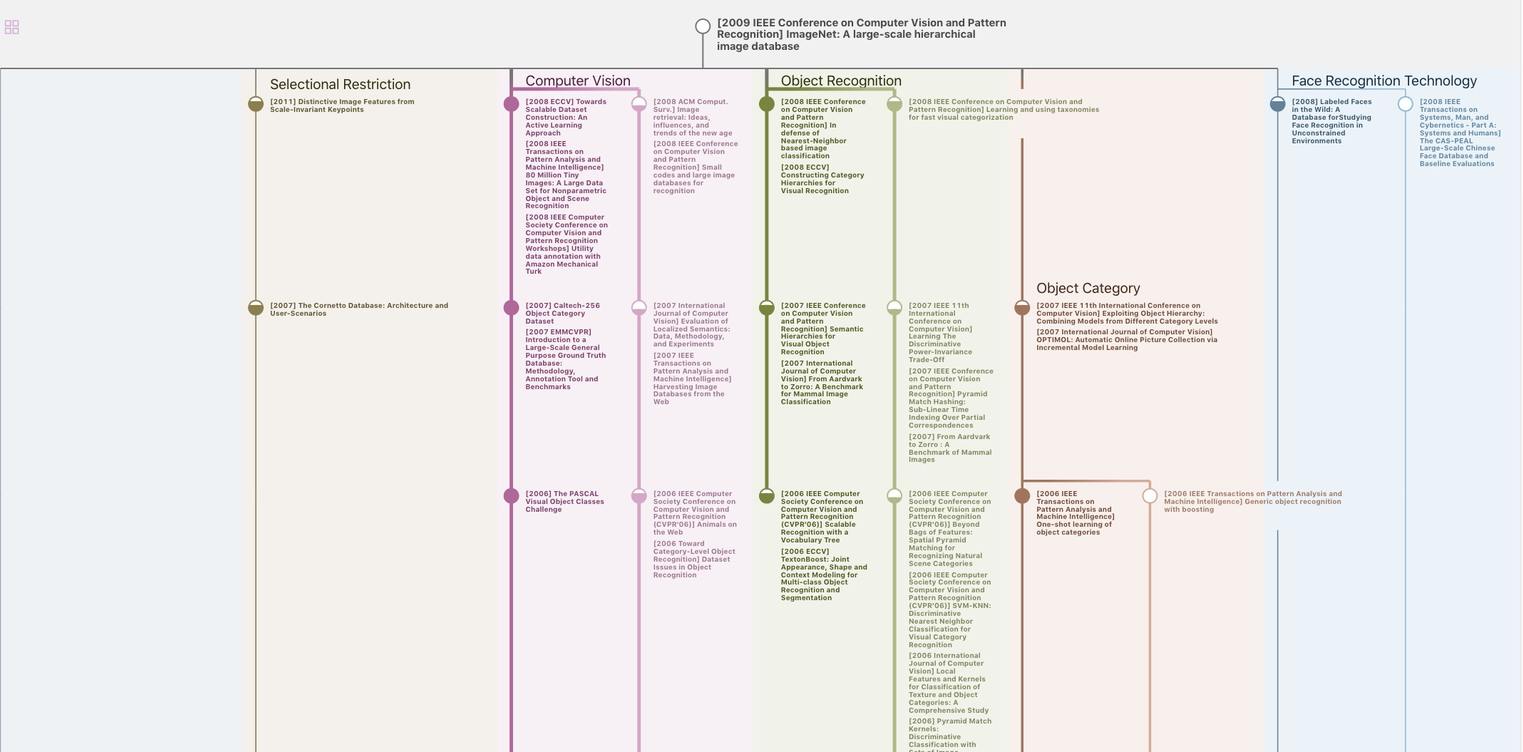
生成溯源树,研究论文发展脉络
Chat Paper
正在生成论文摘要