Intelligent Onboard Routing in Stochastic Dynamic Environments using Transformers
AAMAS '23: Proceedings of the 2023 International Conference on Autonomous Agents and Multiagent Systems(2023)
摘要
Autonomous marine agents find extensive applications in environmental data collection, naval security, and exploration of harsh ocean regions. As intelligent agents, they must perform onboard routing, collect data about their surroundings and update their route to minimize mission travel time, energy, or data collection. While Markov Decision Processes (MDPs) and Reinforcement Learning (RL) are often used for path planning, they are computationally expensive for onboard routing as they need in-mission re-planning. In the present paper, we develop a novel, deep learning method based on the decision transformers for optimal path planning and onboard routing of autonomous marine agents. The transformer architectures convert the RL-based optimal path planning problem into a supervised learning problem via sequence modeling. Before the mission, during the offline planning phase, the environment is first modeled as a stochastic dynamic ocean flow with dynamically orthogonal flow equations. A training dataset for the transformer model is created by solving the stochastic dynamically orthogonal Hamilton-Jacobi level set partial differential equations or a dynamic programming solution for MDPs. These paths are then processed to obtain sequences of states, actions and returns for our transformer models, where the agent's state is typically its spatio-temporal coordinate and other collectible data. We propose and analyze multiple state modeling choices against the agent's state estimation capabilities and scenarios with multiple target locations. We demonstrate that (i) a trained agent learns to infer the surrounding flow and perform optimal onboard routing when the agent's state estimation is accurate,(ii) specifying the target locations (in case of multiple targets) as a part of the state enables a trained agent to route itself to the correct destination, and (iii) a trained agent is robust to limited noise in state transitions and is capable of reaching target locations in completely new flow scenarios. We extensively showcase end-to-end planning and onboard routing in various canonical and idealized ocean flow scenarios. We analyze the predictions of the transformer models and explain the inner mechanics of learning through a novel visualization of self-attention of actions and states on the trajectories.
更多查看译文
AI 理解论文
溯源树
样例
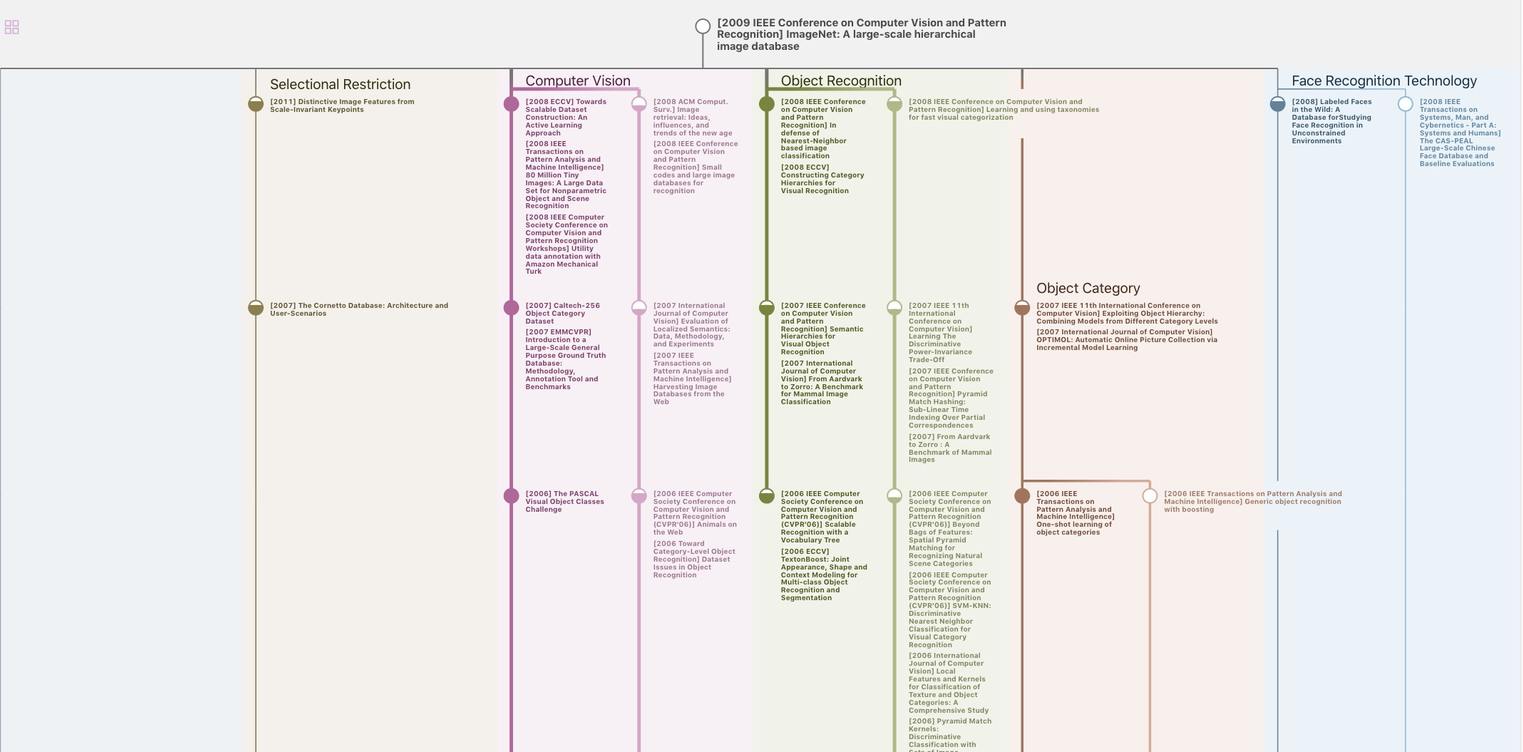
生成溯源树,研究论文发展脉络
Chat Paper
正在生成论文摘要