Adaptive PD Control using Deep Reinforcement Learning for Local-Remote Teleoperation with Stochastic Time Delays
2023 IEEE/RSJ INTERNATIONAL CONFERENCE ON INTELLIGENT ROBOTS AND SYSTEMS (IROS)(2023)
Abstract
Local-remote systems allow robots to execute complex tasks in hazardous environments such as space and nuclear power stations. However, establishing accurate positional mapping between local and remote devices can be difficult due to time delays that can compromise system performance and stability. Enhancing the synchronicity and stability of local-remote systems is vital for enabling robots to interact with environments at greater distances and under highly challenging network conditions, including time delays. We introduce an adaptive control method employing reinforcement learning to tackle the time-delayed control problem. By adjusting controller parameters in real-time, this adaptive controller compensates for stochastic delays and improves synchronicity between local and remote robotic manipulators. To improve the adaptive PD controller's performance, we devise a model-based reinforcement learning approach that effectively incorporates multi-step delays into the learning framework. Utilizing this proposed technique, the local-remote system's performance is stabilized for stochastic communication time-delays of up to 290ms. Our results demonstrate that the suggested model-based reinforcement learning method surpasses the Soft-Actor Critic and augmented state Soft-Actor Critic techniques. Access the code at: https://github.com/CAV-Research-Lab/Predictive-Model-Delay-Correction
MoreTranslated text
Key words
teleoperation,adaptive pd control,deep reinforcement learning,local-remote
AI Read Science
Must-Reading Tree
Example
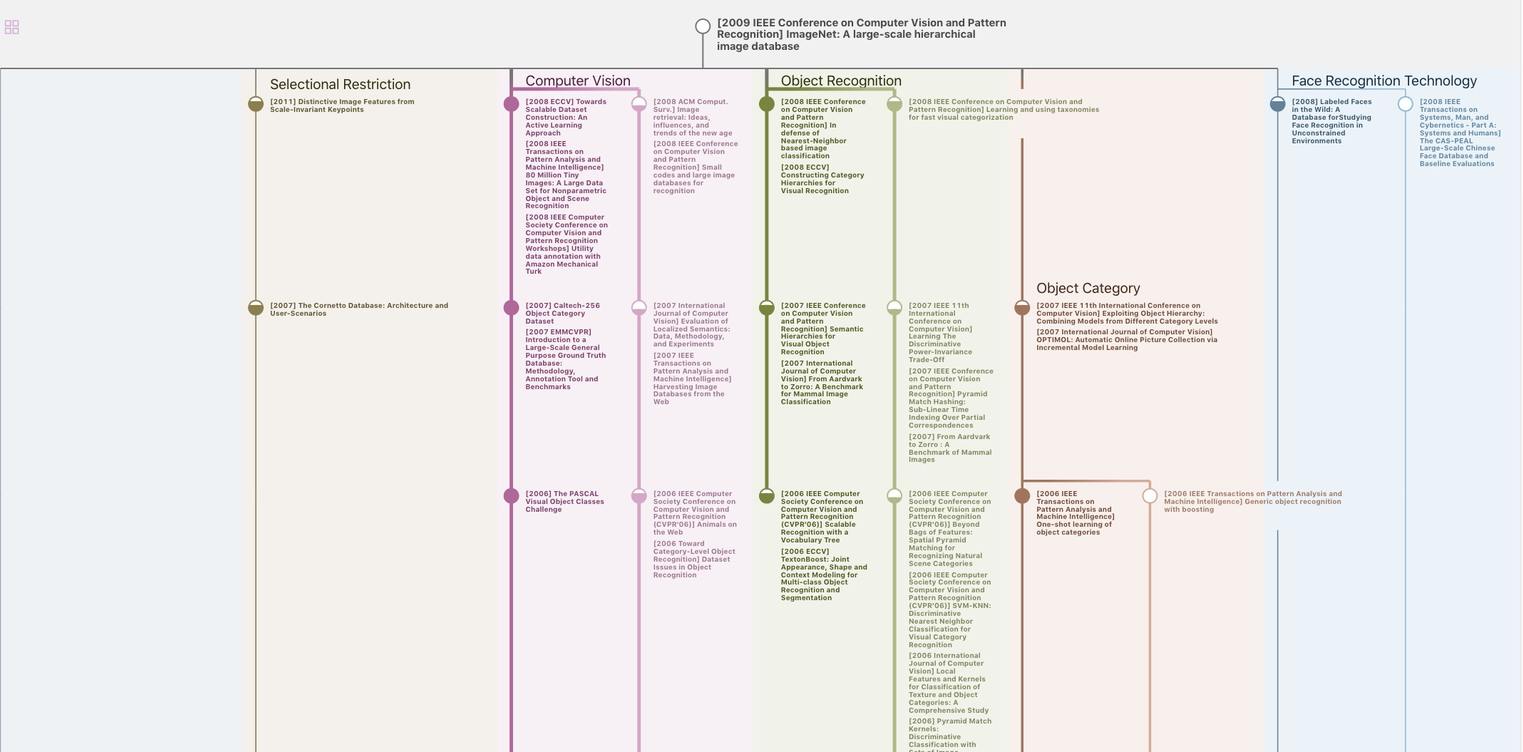
Generate MRT to find the research sequence of this paper
Chat Paper
Summary is being generated by the instructions you defined