Error Bounds for Flow Matching Methods
CoRR(2023)
摘要
Score-based generative models are a popular class of generative modelling techniques relying on stochastic differential equations (SDE). From their inception, it was realized that it was also possible to perform generation using ordinary differential equations (ODE) rather than SDE. This led to the introduction of the probability flow ODE approach and denoising diffusion implicit models. Flow matching methods have recently further extended these ODE-based approaches and approximate a flow between two arbitrary probability distributions. Previous work derived bounds on the approximation error of diffusion models under the stochastic sampling regime, given assumptions on the $L^2$ loss. We present error bounds for the flow matching procedure using fully deterministic sampling, assuming an $L^2$ bound on the approximation error and a certain regularity condition on the data distributions.
更多查看译文
关键词
error bounds,flow,matching,methods
AI 理解论文
溯源树
样例
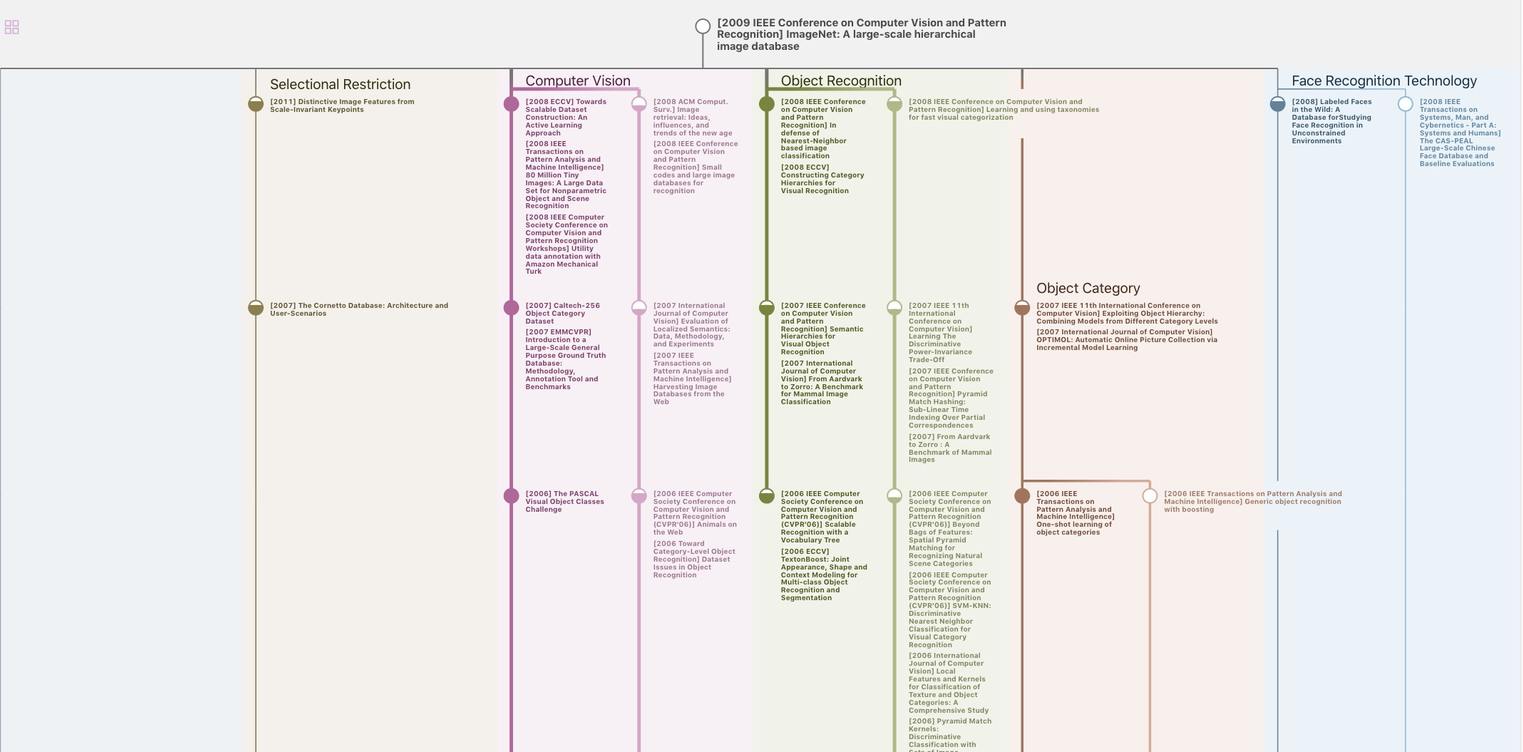
生成溯源树,研究论文发展脉络
Chat Paper
正在生成论文摘要