Federated Composite Saddle Point Optimization
CoRR(2023)
摘要
Federated learning (FL) approaches for saddle point problems (SPP) have recently gained in popularity due to the critical role they play in machine learning (ML). Existing works mostly target smooth unconstrained objectives in Euclidean space, whereas ML problems often involve constraints or non-smooth regularization, which results in a need for composite optimization. Addressing these issues, we propose Federated Dual Extrapolation (FeDualEx), an extra-step primal-dual algorithm, which is the first of its kind that encompasses both saddle point optimization and composite objectives under the FL paradigm. Both the convergence analysis and the empirical evaluation demonstrate the effectiveness of FeDualEx in these challenging settings. In addition, even for the sequential version of FeDualEx, we provide rates for the stochastic composite saddle point setting which, to our knowledge, are not found in prior literature.
更多查看译文
关键词
optimization,composite
AI 理解论文
溯源树
样例
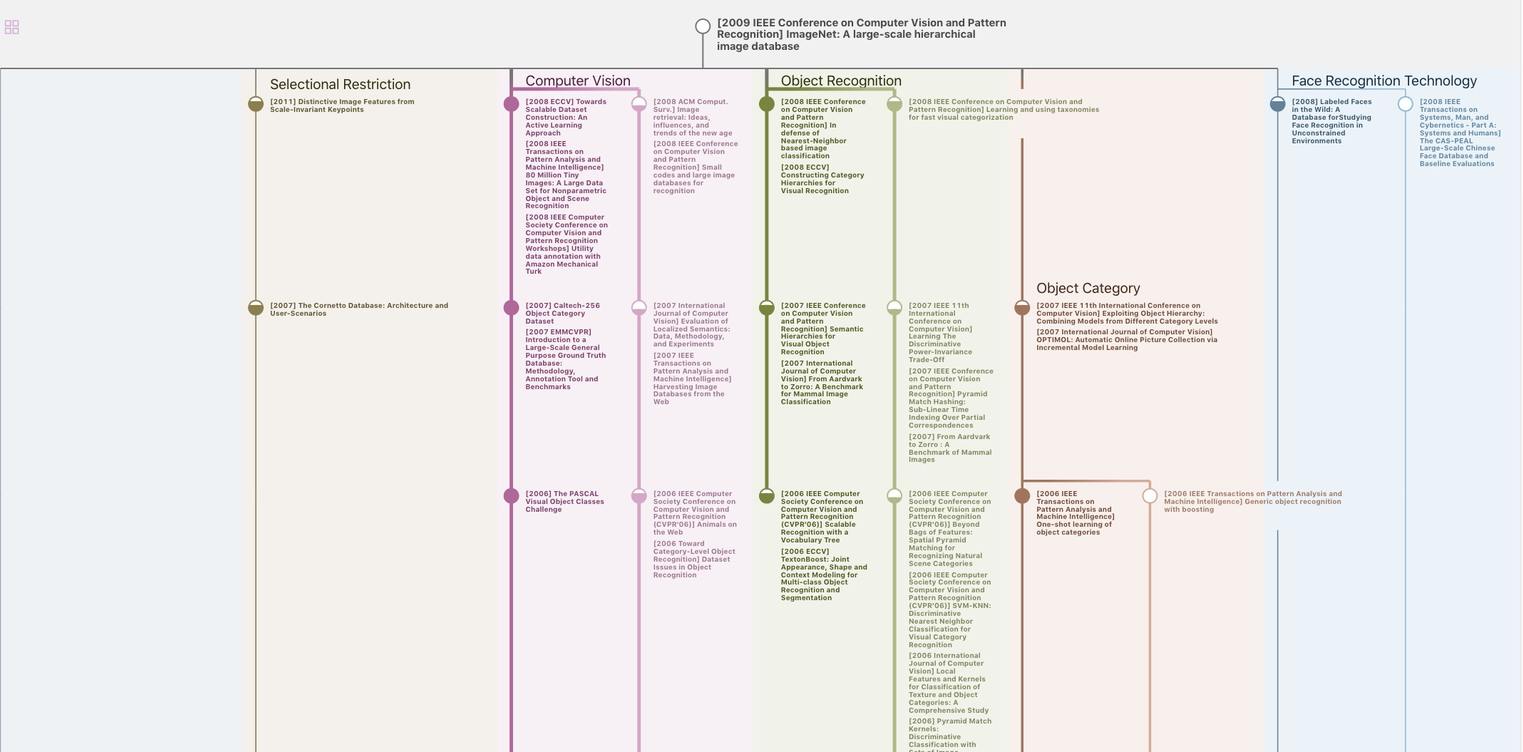
生成溯源树,研究论文发展脉络
Chat Paper
正在生成论文摘要