A Privacy-Preserving Finite-Time Push-Sum based Gradient Method for Distributed Optimization over Digraphs
CoRR(2023)
摘要
This paper addresses the problem of distributed optimization, where a network of agents represented as a directed graph (digraph) aims to collaboratively minimize the sum of their individual cost functions. Existing approaches for distributed optimization over digraphs, such as Push-Pull, require agents to exchange explicit state values with their neighbors in order to reach an optimal solution. However, this can result in the disclosure of sensitive and private information. To overcome this issue, we propose a state-decomposition-based privacy-preserving finite-time push-sum (PrFTPS) algorithm without any global information such as network size or graph diameter. Then, based on PrFTPS, we design a gradient descent algorithm (PrFTPS-GD) to solve the distributed optimization problem. It is proved that under PrFTPS-GD, the privacy of each agent is preserved and the linear convergence rate related to the optimization iteration number is achieved. Finally, numerical simulations are provided to illustrate the effectiveness of the proposed approach.
更多查看译文
关键词
Distributed optimization,privacy-preserving,finite-time consensus,directed graph
AI 理解论文
溯源树
样例
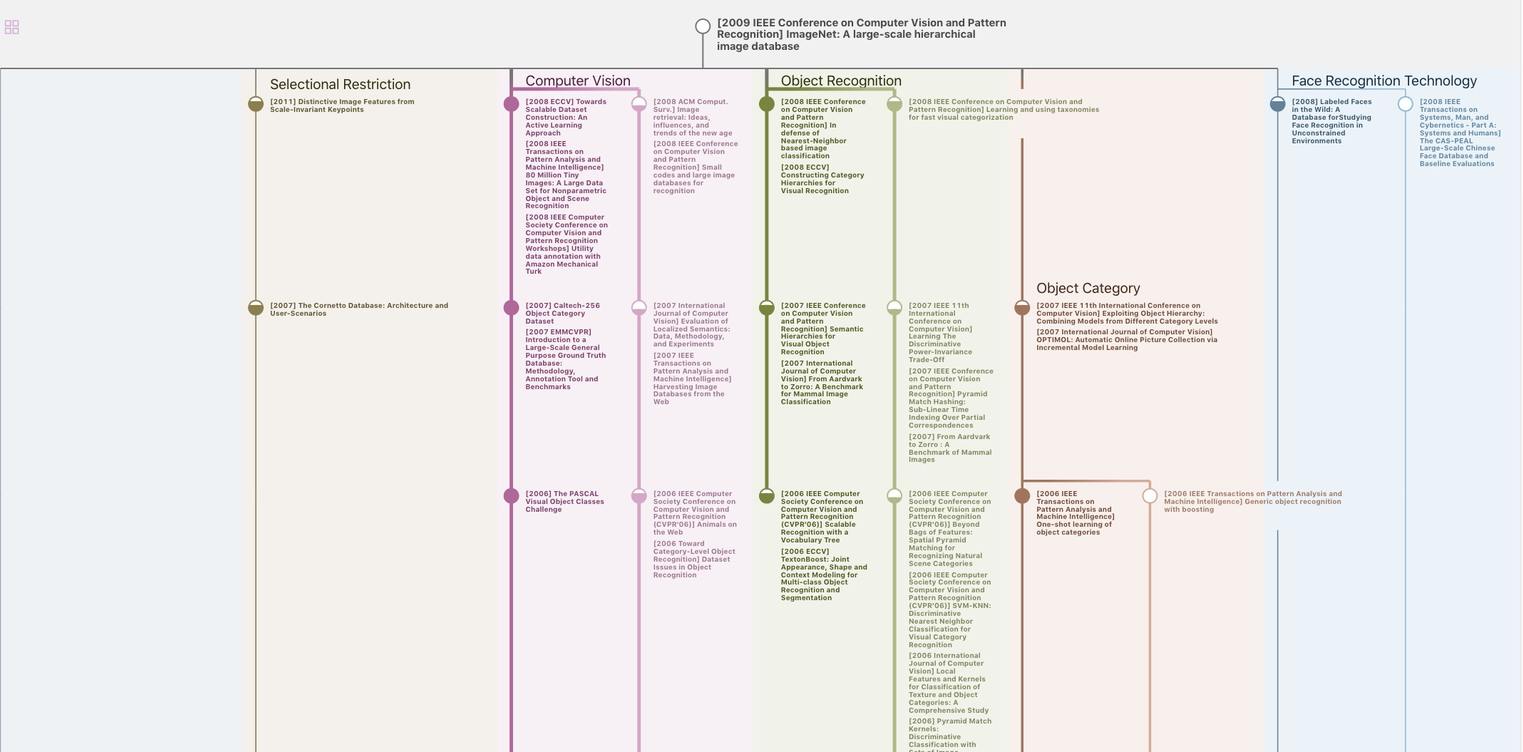
生成溯源树,研究论文发展脉络
Chat Paper
正在生成论文摘要