Faithful and Efficient Explanations for Neural Networks via Neural Tangent Kernel Surrogate Models
arXiv (Cornell University)(2023)
摘要
A recent trend in explainable AI research has focused on surrogate modeling,
where neural networks are approximated as simpler ML algorithms such as kernel
machines. A second trend has been to utilize kernel functions in various
explain-by-example or data attribution tasks. In this work, we combine these
two trends to analyze approximate empirical neural tangent kernels (eNTK) for
data attribution. Approximation is critical for eNTK analysis due to the high
computational cost to compute the eNTK. We define new approximate eNTK and
perform novel analysis on how well the resulting kernel machine surrogate
models correlate with the underlying neural network. We introduce two new
random projection variants of approximate eNTK which allow users to tune the
time and memory complexity of their calculation. We conclude that kernel
machines using approximate neural tangent kernel as the kernel function are
effective surrogate models, with the introduced trace NTK the most consistent
performer. Open source software allowing users to efficiently calculate kernel
functions in the PyTorch framework is available
(https://github.com/pnnl/projection_ntk).
更多查看译文
关键词
Explainability,Surrogate Models,Neural Tangent Kernel,Deep Learning,Attribution
AI 理解论文
溯源树
样例
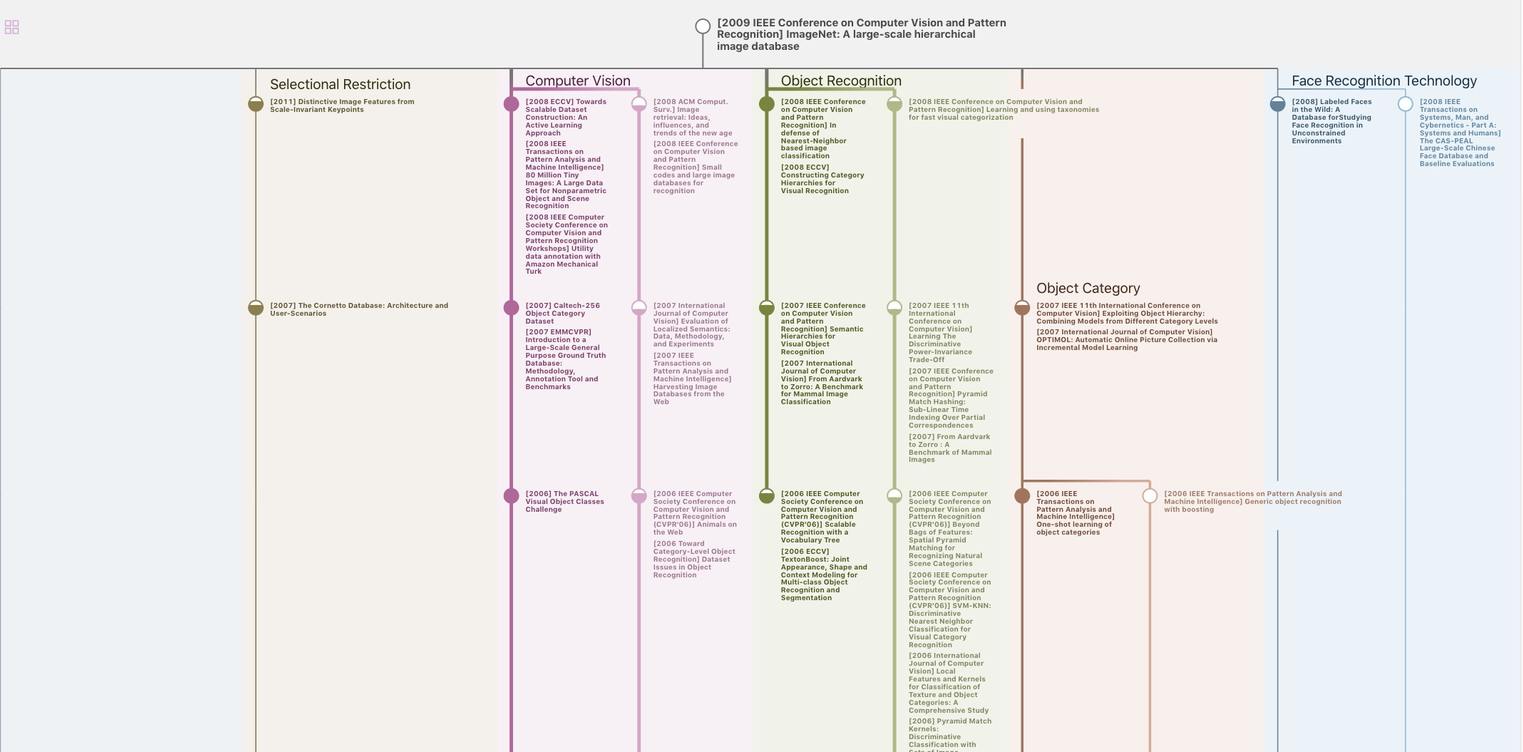
生成溯源树,研究论文发展脉络
Chat Paper
正在生成论文摘要