Constituent Attention for Vision Transformers
COMPUTER VISION AND IMAGE UNDERSTANDING(2023)
Abstract
Multi-head self-attention (MSA) endows vision Transformers (ViTs) with the ability of modeling long-range interactions between tokens. However, recent works have uncovered that current arbitrary attention makes tokens distracted by extraneous dependencies, complicating the understanding of ViTs and hindering the overall optimization of ViTs. In this paper, we propose Constituent Attention (CA), a straightforward yet effective constraint on MSA to resolve aforementioned issues in different variants of ViTs with negligible overhead. Specifically, CA is designed to encourage the mutual attention between spatially connectable tokens via gathering such token pairs to the same group (termed constituent). Furthermore, with the layers going deeper in ViTs, small adjacent constituents are gradually merged into larger ones, exploring hierarchical structures in the visual objectives. Extensive experiments on four popular benchmarks demonstrate that CA assists the optimization of ViTs and contributes to significant performance gains especially at data -scarce scenarios. Moreover, the layer-wise reasoning mechanism aids ViTs in more consistent and progressive attention across different layers, rendering the inner workings easier to interpret. Our code is publicly available at https://github.com/zju-vipa/ConstituentAttention.
MoreTranslated text
Key words
Vision Transformer,Attention mechanism,Classification,Interpretability for deep learning
AI Read Science
Must-Reading Tree
Example
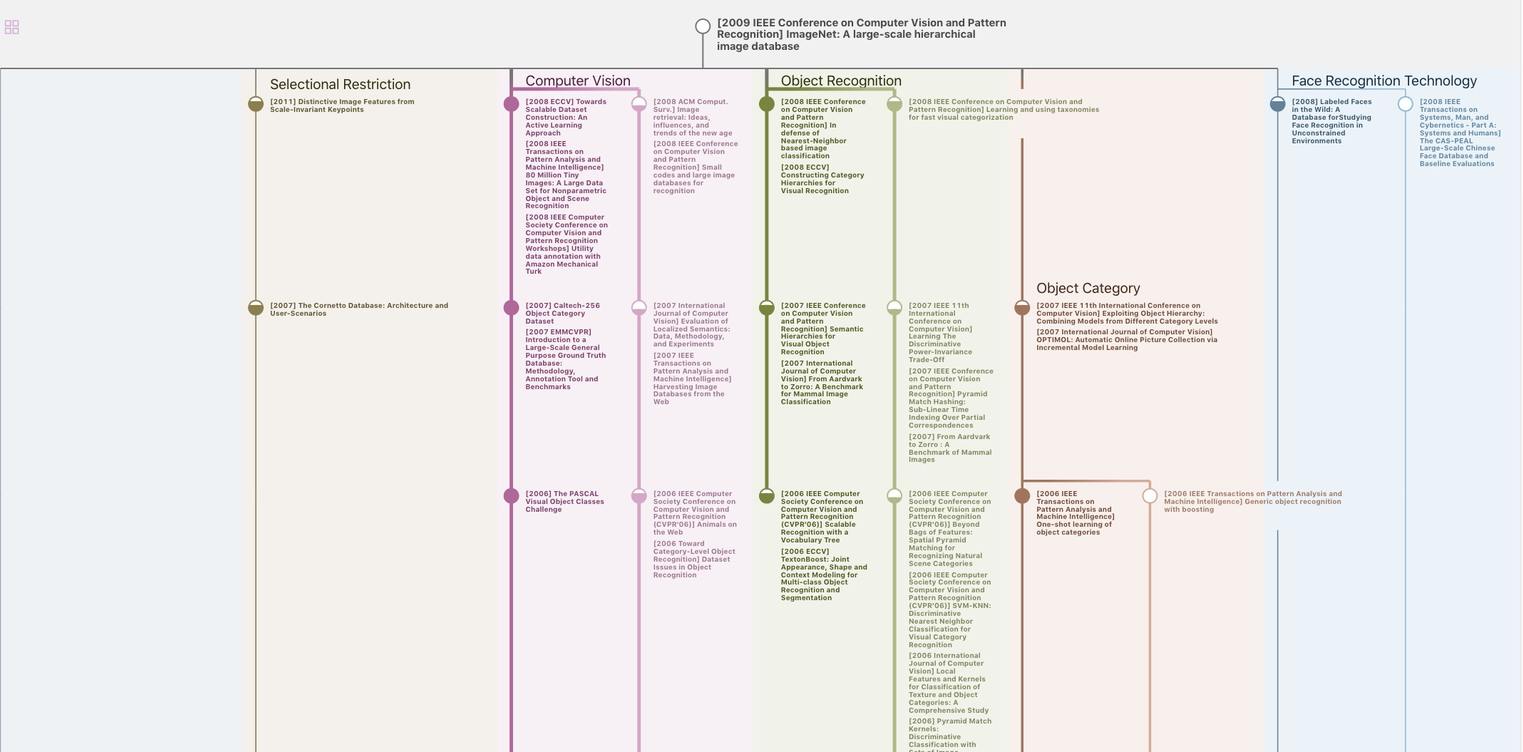
Generate MRT to find the research sequence of this paper
Chat Paper
Summary is being generated by the instructions you defined