Data-driven Track Geometry Fault Localisation Using Unsupervised Machine Learning
Construction & building materials(2023)
摘要
This study presents a pipeline of two unsupervised machine learning models (autoencoder and KMeans clustering) used to localise the on-going development of track defects based on geometry measurements. The data used covers over 15 years of inspections carried out on a high-speed track using a specialised recording vehicle. The models make use of a moving frame of reference to estimate track quality, rather than discrete segments of a fixed length. In doing so, an autoencoder can highlight more precisely where changes in geometry are occurring on the track, and the clustering algorithm is able to further classify those regions of higher settlement. The algorithms were found to be highly successful in identifying such regions of the track. The work presented here can help develop a more targeted maintenance approach, rather than the current preventive and corrective techniques, which may lead to over-tamping of the track. Tamping is known to additionally degrade ballast condition, by causing particle breakage and subsequent fouling. By identifying and aiming to remove specific defects, and thus reducing the amount of track tamped annually, the lifespan of ballast can be extended, postponing renewal works and reducing lifecycle costs.
更多查看译文
关键词
Railway track geometry,Big data,Machine learning,Track fault localisation,Unsupervised learning
AI 理解论文
溯源树
样例
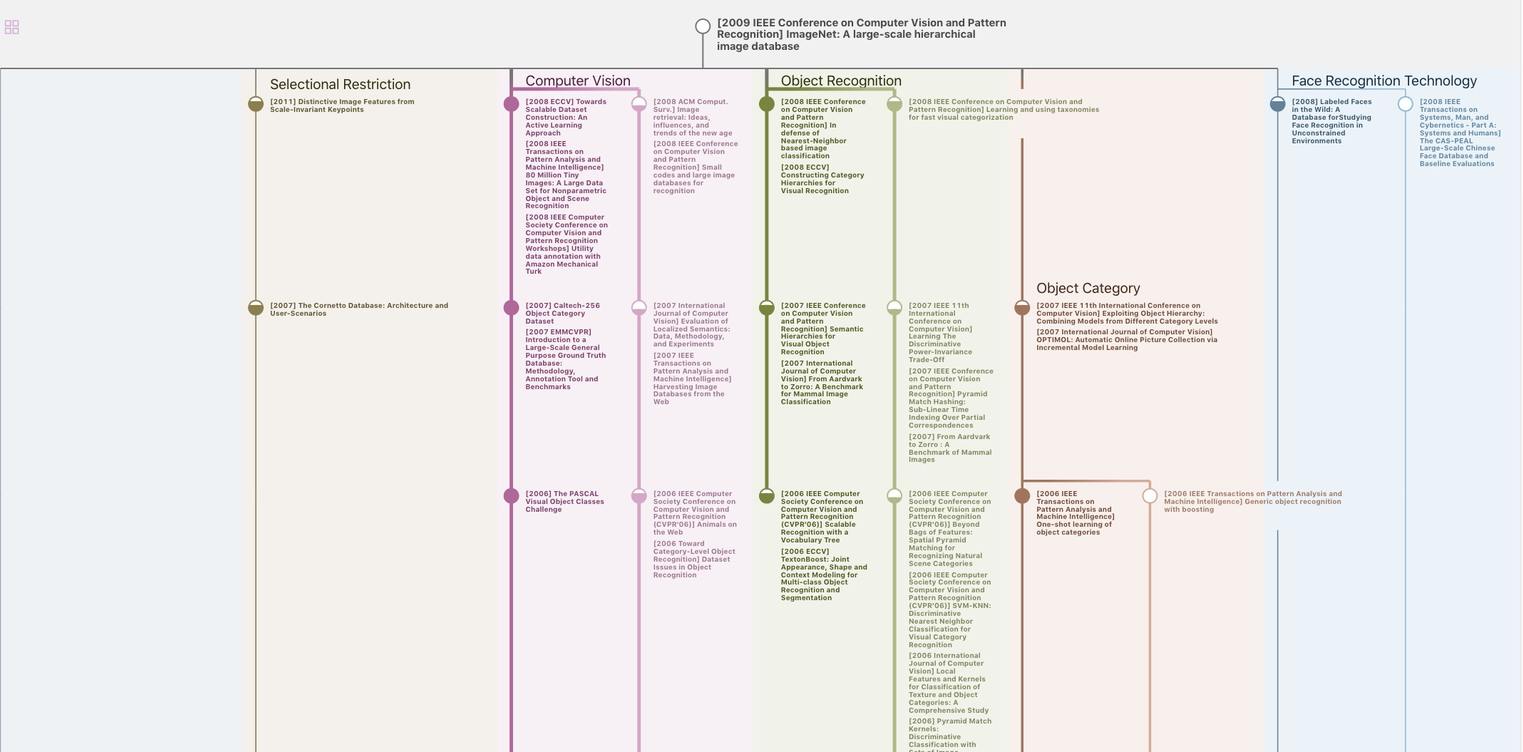
生成溯源树,研究论文发展脉络
Chat Paper
正在生成论文摘要