The Ear Unwrapper: A Maize Ear Image Acquisition and Machine Learning Pipeline for Disease Severity Phenotyping
Research Square (Research Square)(2023)
Abstract
Abstract Background To successfully breed any plant for disease resistance, an accurate method of phenotyping disease severity is crucial. Fusarium ear rot (FER) is a common disease of maize ( Zea mays ) caused by the pathogen Fusarium verticillioides (Sacc.) Nirenberg (synonym F. moniliforme Sheldon, teleomorph Gibberella moniliformis Wineland). Because of the quantitative nature of the disease, scoring disease severity is difficult and nuanced, relying on various ways to quantify damage caused by the pathogen. Towards the goal of designing a system with greater objectivity, reproducibility, and accuracy than subjective scores or estimations of area damaged, a system of semi-automated image acquisition and subsequent image analysis was designed. Results The tool created for image acquisition, “The Ear Unwrapper”, successfully obtains images of the full exterior of maize ears in roughly 10 seconds. To “unwrap” each ear of maize, the approach was to rotate the ear around its axis (the cob) and using a camera, take a continuous set of images of a single row of pixels and merge them to form one image. A set of images produced from The Ear Unwrapper were used to produce a probabilistic pixel classification model for predicting disease severity from unannotated images. The system was deliberately constructed using open-source software and off-the-shelf parts so that the image acquisition and analysis pipeline is adaptable for quantifying other maize ear pathogens, morphologies, and phenotypes. The data obtained from The Ear Unwrapper was correlated with two other phenotyping methods for validation and comparison and showed that the output from the system was reasonably accurate to determine lesion size. Conclusions This study provides an example of how a simplified image acquisition machine can be built and incorporated into a machine learning pipeline to measure phenotypes of interest. Here, The Ear Unwrapper was built to image ears of maize, but other cylindrical objects can also be “unwrapped” to obtain a single image of the object’s exterior. We also present how the use of machine learning in image analysis can be adapted from open-source software to estimate complex phenotypes, here, disease severity of Fusarium Ear Rot.
MoreTranslated text
Key words
maize ear image acquisition,ear unwrapper,disease severity phenotyping,machine learning pipeline
AI Read Science
Must-Reading Tree
Example
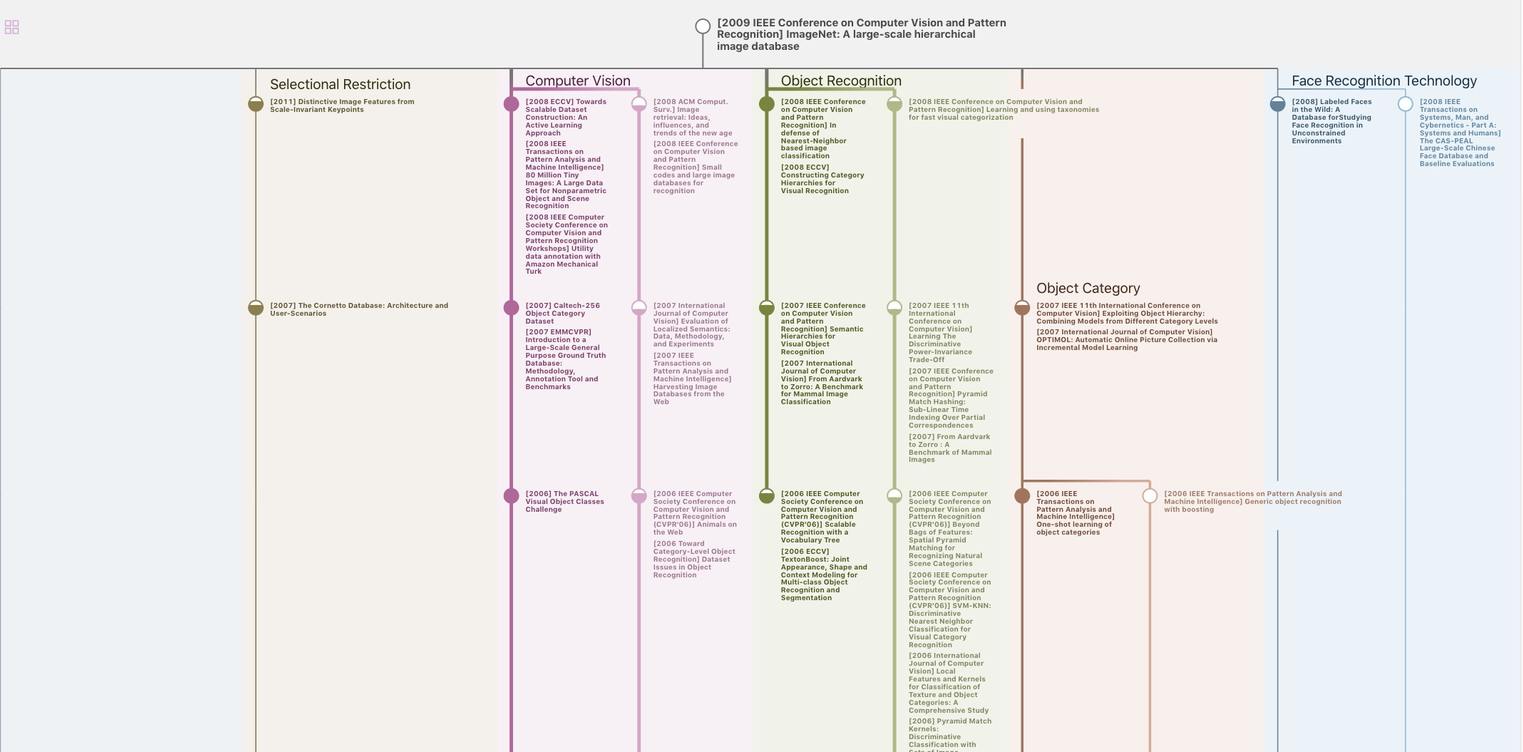
Generate MRT to find the research sequence of this paper
Chat Paper
Summary is being generated by the instructions you defined