SMARTformer: Semi-Autoregressive Transformer with Efficient Integrated Window Attention for Long Time Series Forecasting
IJCAI 2023(2023)
摘要
Transformers have achieved remarkable performance in long time series forecasting (LTSF), thanks to their powerful capture of long-range dependencies. However, the prediction on long time sequences has been significantly affected by the ability of capturing reliable local dependencies in segments of sequences. To address this issue, we introduce the SMARTformer denoting SeMiAutoRegressive Transformer with Efficient Integrated Window Attention. In detail, the semi-autoregressive (SAR) decoder first predicts each segment of the sequence iteratively to comprehensively capture local context in a way as autoregressive (AR) decoding; based on the previous output, it then refines the whole sequence in a non-autoregressive (NAR) way. Therefore, SAR benefits from both the global horizon of NAR and local detail capturing of AR. Moreover, it can be used as a general plug-in to further enhance the predicting performance of various transformer models on time series. Furthermore, to achieve complementary clues in local and enlarged receptive fields, we propose the Integrated Window Attention to separately conduct both local self-attention in multi-scale windows and global attention across windows. Especially, with a linear complexity, this design also brings significant improvement in computational efficiency. Finally, extensive studies on five benchmark datasets show the effectiveness of SMARTformer against SOTA works, with an improvement of 10.2% and 18.4% in multivariate and univariate long-term forecasting, respectively.
更多查看译文
AI 理解论文
溯源树
样例
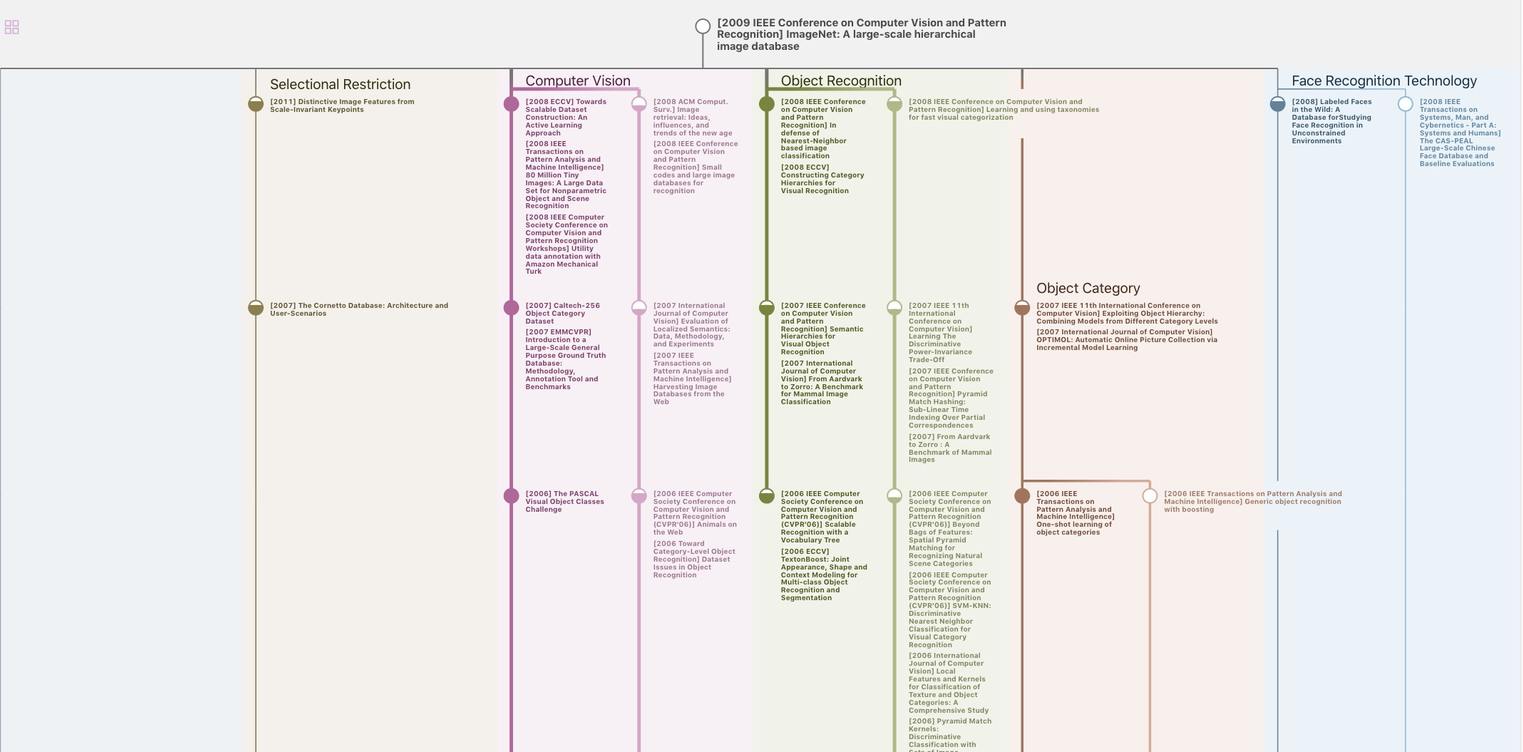
生成溯源树,研究论文发展脉络
Chat Paper
正在生成论文摘要