A GNN Computing-in-Memory Macro and Accelerator with Analog-Digital Hybrid Transformation and CAMenabled Search-reduce
2023 IEEE Custom Integrated Circuits Conference (CICC)(2023)
摘要
Graph Neural Networks (GNN) recently find many exciting applications. Despite previous approaches [1], [2], accelerating spatial GNN remains challenging due to its unbalanced computing flow, poor locality, high sparsity, and high memory bandwidth requirements, especially for edge applications such as real-time motion detectors and point cloud processing. This work presents the first GNN computing-in-memory (CIM) macro and accelerator chip, addressing major issues and achieving up to 78.6 X improvement in system energy efficiency compared with previous implementations.
更多查看译文
AI 理解论文
溯源树
样例
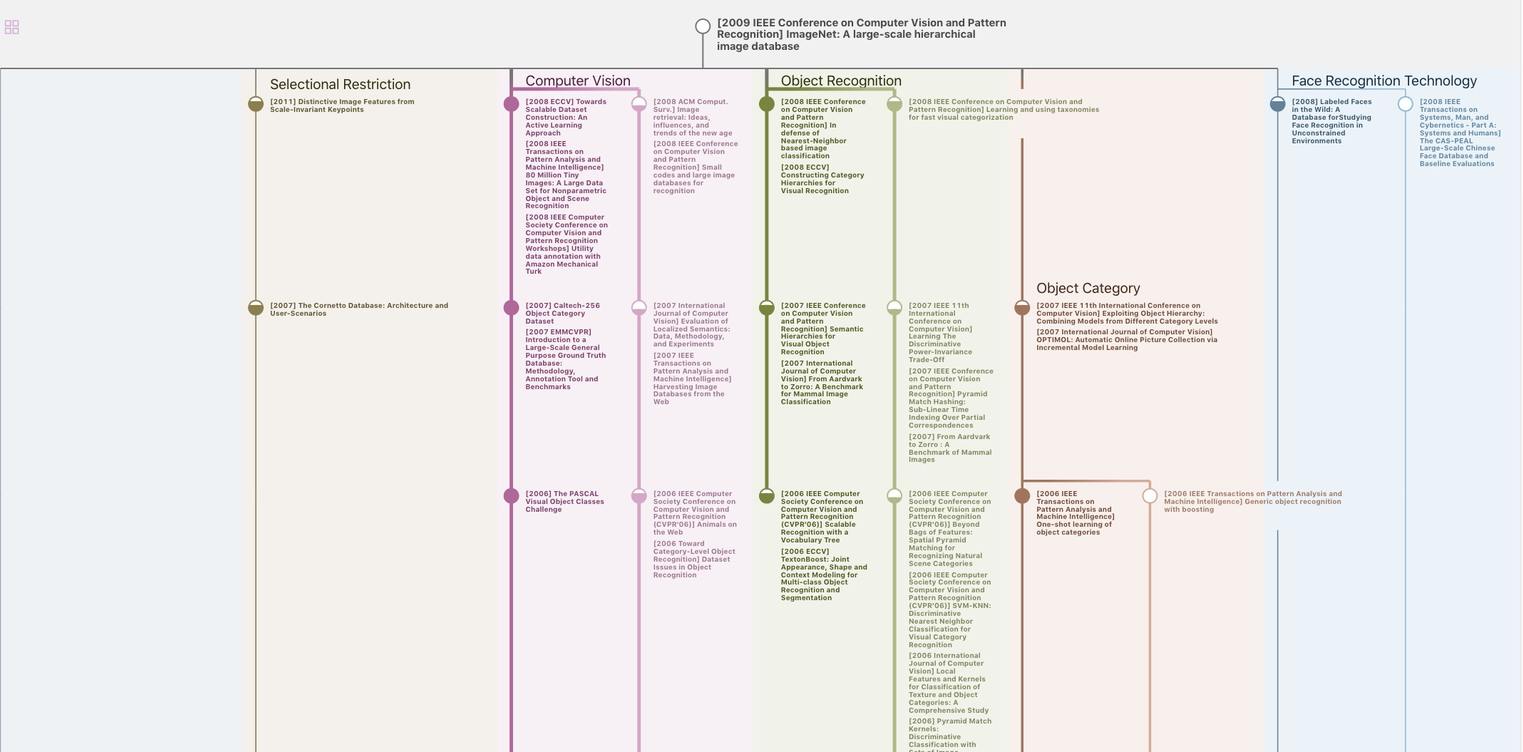
生成溯源树,研究论文发展脉络
Chat Paper
正在生成论文摘要