Value-aware Meta-Transfer Learning and Convolutional Mask Attention Networks for Reservoir Identification with Limited Data
EXPERT SYSTEMS WITH APPLICATIONS(2023)
摘要
Reservoir identification is important for reservoir evaluation and petroleum development. Existing methods cannot automatically identify the categories of the reservoir that exhibit: (a) local features differences of well logging data; (b) limited with non-reservoir interference; and (c) insufficient real labels. Transfer learning-based methods utilize other blocks partially address the problem of small samples. However, they ignore the significant geological differences between blocks. Therefore, this paper proposes a small sample reservoir identification method combining Convolutional Mask Attention Network (CMAN) and Value-aware Meta-Transfer Learning (VMTL) strategy. First, we pre-train the CMAN on the source block to adaptively extract the local information of each depth point. The CMAN also automatically masks the non-reservoir information while capturing the relationship between reservoirs and non-reservoirs to improve feature extraction. Then we design a VMTL strategy to learn valuable transfer knowledge for overcoming the geological difference. Finally, we fine-tune our model using target block data to address the insufficient samples. The average accuracy and F1 score of the proposed method on real-world oilfield data are respectively 92.61% and 88.85%. The results of the two cases demonstrate our method outperforms existing methods in convergence speed, stability, and generalizability.
更多查看译文
关键词
Reservoir identification,Convolutional mask-attention,Value-aware meta-learning,Transfer learning,Deep learning
AI 理解论文
溯源树
样例
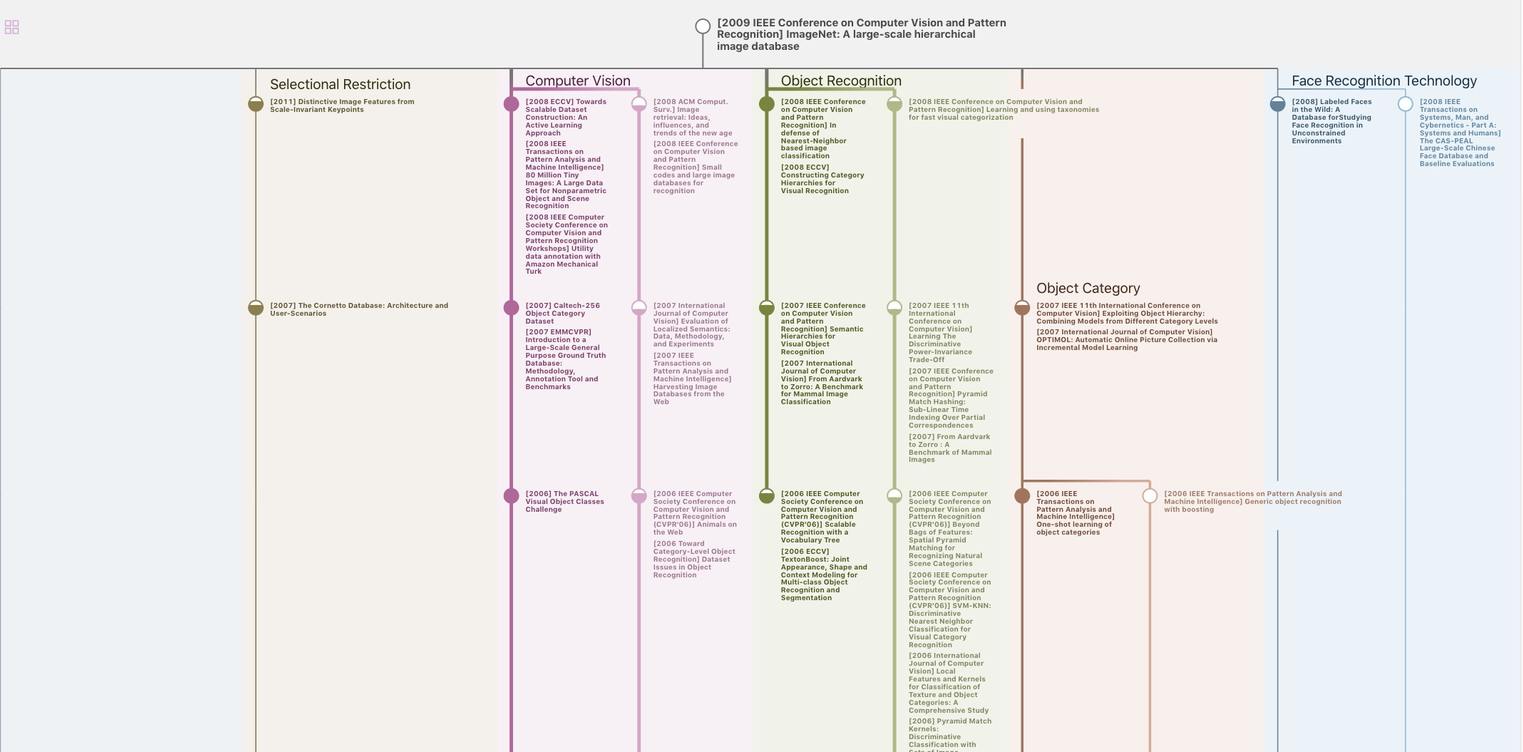
生成溯源树,研究论文发展脉络
Chat Paper
正在生成论文摘要