Penalized Weighted Least-Squares Estimate for Variable Selection on Correlated Multiply Imputed Data
Applied statistics/Journal of the Royal Statistical Society Series C, Applied statistics(2023)
摘要
Considering the inevitable correlation among different datasets within the same subject, we propose a framework of variable selection on multiply imputed data with penalized weighted least squares (PWLS-MI). The methodological development is motivated by an epidemiological study of A/H7N9 patients from Zhejiang province in China, where nearly half of the variables are not fully observed. Multiple imputation is commonly adopted as a missing data processing method. However, it generates correlations among imputed values within the same subject across datasets. Recent work on variable selection for multiply imputed data does not fully address such similarities. We propose PWLS-MI to incorporate the correlation when performing the variable selection. PWLS-MI can be considered as a framework for variable selection on multiply imputed data since it allows various penalties. We use adaptive LASSO as an illustrating example. Extensive simulation studies are conducted to compare PWLS-MI with recently developed methods and the results suggest that the proposed approach outperforms in terms of both selection accuracy and deletion accuracy. PWLS-MI is shown to select variables with clinical relevance when applied to the A/H7N9 database.
更多查看译文
关键词
multiple imputation,variable selection,weighted least squares
AI 理解论文
溯源树
样例
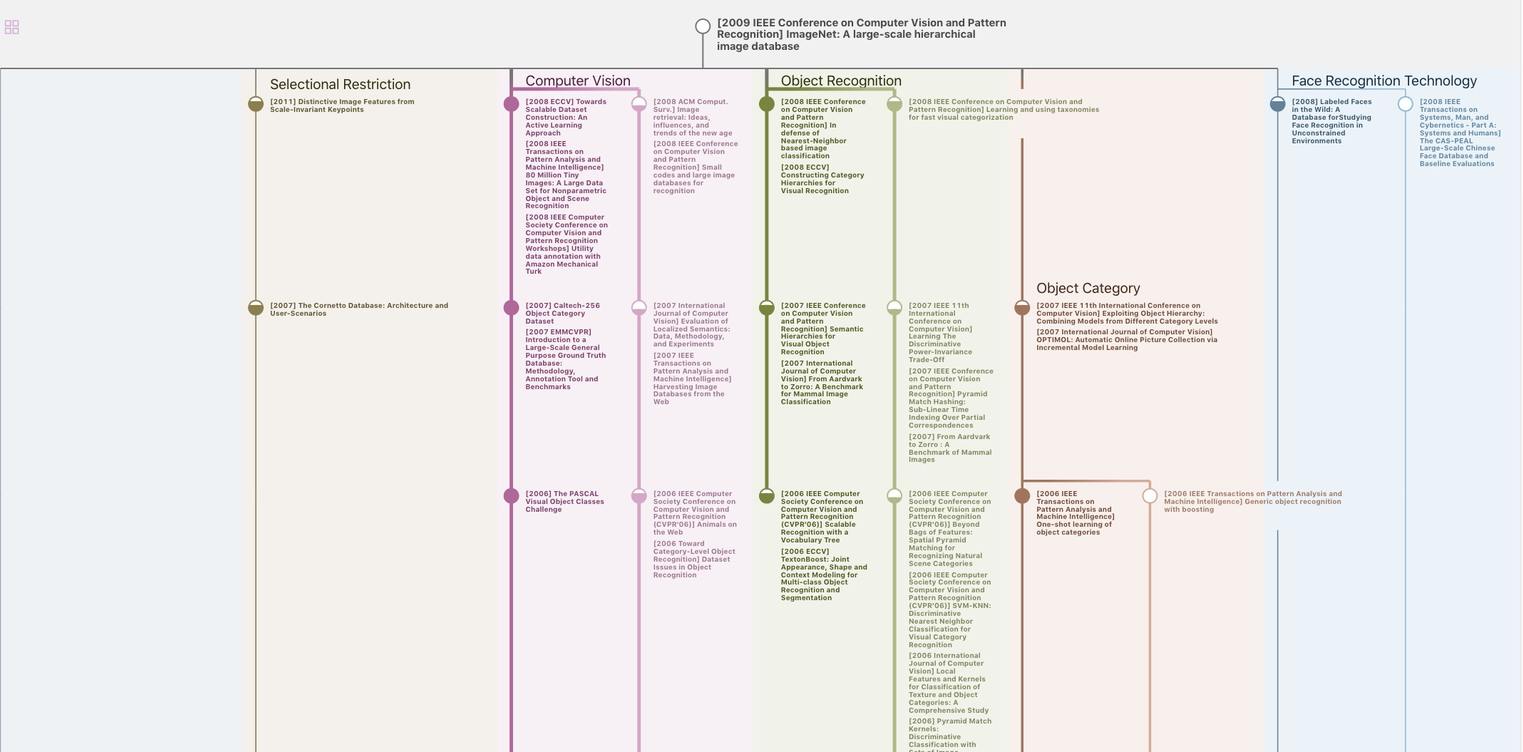
生成溯源树,研究论文发展脉络
Chat Paper
正在生成论文摘要