Reference Evapotranspiration Prediction Using Artifiicial Neural Networks
CIENCIAUAT(2023)
摘要
Reference evapotranspiration (ETo) is a hydro-logical variable of great importance in irrigation management. Its estimation is carried out with the Penman-Montieth (PM) equation that requi-res many meteorological variables and that are sometimes not available. Since ETo is a nonli-near and complex variable, in recent years al-ternative methods have emerged for its estima-tion, such as artificial neural networks (ANN). The objective of this work was to estimate the re-ference evapotranspiration (ETo) using the Pen-man-Montieth equation, in order to develop arti-ficial neural network (ANN) models that allow ETo to be predicted in regions with limited clima-tological information, and in turn to compare the performance of three RNA models: FFNN, ERNN and NARX. Daily informtion was used during the January 1, 2007 to December 31, 2018 period, for the ENP8 and ENP4 meteorological stations in Mexico city. Based on the correlation analysis and the Garson sensitivity analysis, 2 cases were studied for the 3 ANN models: 1) ANN with 6 in-puts: solar radiation (Rad), maximum and mini-mum temperature (Tmax, Tmin), maximum and minimum relative humidity (RHmax, RHmin), and wind speed (u),and 2) RNA with 2 inputs (Rad and Tmax). The output variable was the ETo, calculated with the PM equation. In all ca-ses, the performance of the 3 ANNs was very si-milar. The most notable difference is that the dy-namic networks (ERNN and NARX) require fe-wer iterations to achieve the optimum perfor-mance. ANNs trained only with radiation and maximum temperature as inputs were able to predict a long-term ETo for 440 at another near-by meteorological station (ENP4), with efficien-cies greater than 90 %.
更多查看译文
关键词
neural networks,evapotranspira-tion,FFNN,ERNN,NARX
AI 理解论文
溯源树
样例
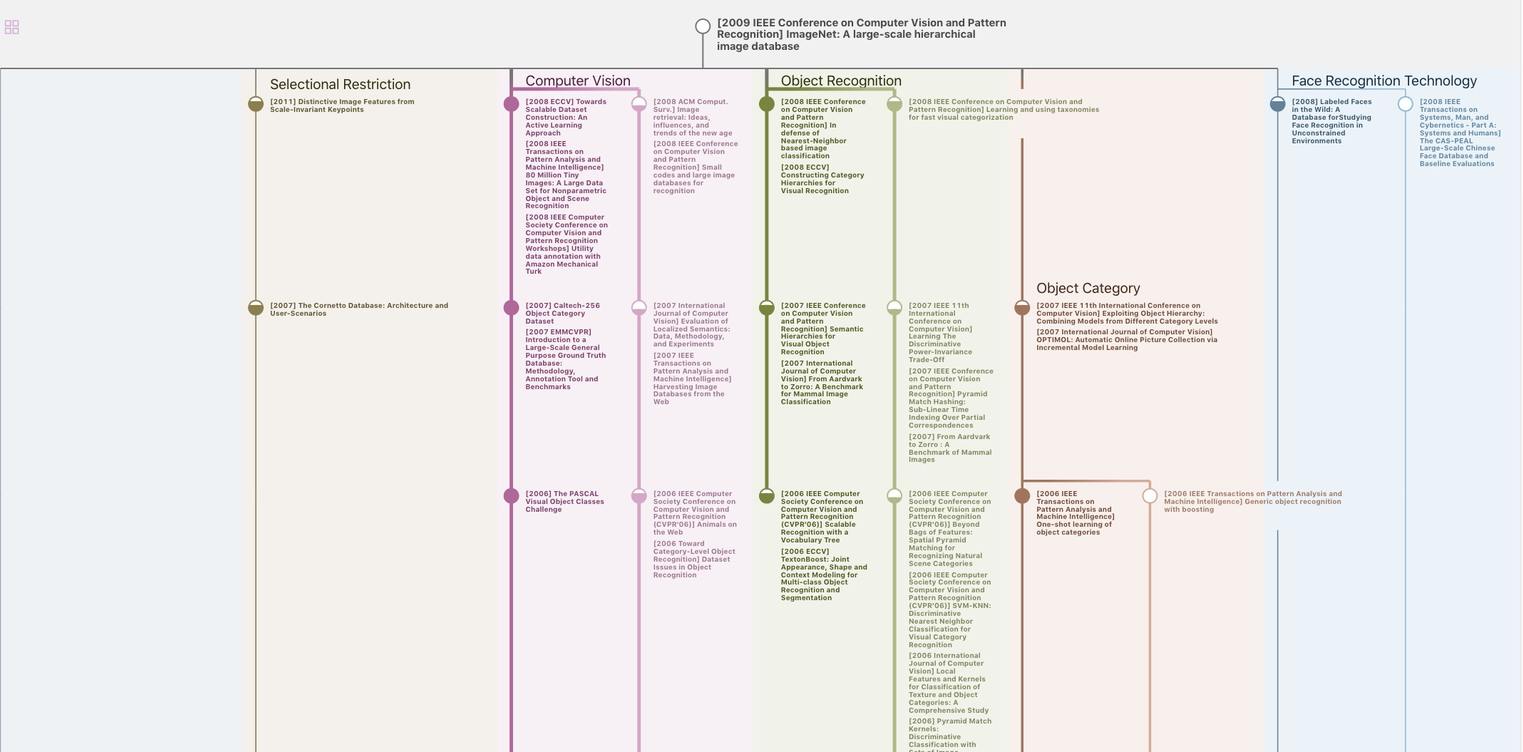
生成溯源树,研究论文发展脉络
Chat Paper
正在生成论文摘要