Automated Protein Function Description for Novel Class Discovery
biorxiv(2022)
摘要
Knowledge of protein function is necessary for understanding biological systems, but the discovery of new sequences from high-throughput sequencing technologies far outpaces their functional characterization. Beyond the problem of assigning newly sequenced proteins to known functions, a more challenging issue is discovering novel protein functions. The space of possible functions becomes unlimited when considering designed proteins. Protein function prediction, as it is framed in the case of Gene Ontology term prediction, is a multilabel classification problem with a hierarchical label space. However, this framing does not provide guiding principles for discovering completely novel functions. Here we propose a neural machine translation model in order to generate descriptions of protein functions in natural language. In this way, instead of making predictions in a limited label space, our model generates descriptions in the language space, and thus is capable of composing novel functions. Given the novelty of our approach, we design metrics to evaluate the performance of our model: correctness, specificity and robustness. We provide results of our model in the zero-shot classification setting, scoring functional descriptions that the model has not seen before for proteins that have limited homology to those in the training set. Finally, we show generated function descriptions compared to ground truth descriptions for qualitative evaluation.
### Competing Interest Statement
The authors have declared no competing interest.
更多查看译文
关键词
protein,discovery
AI 理解论文
溯源树
样例
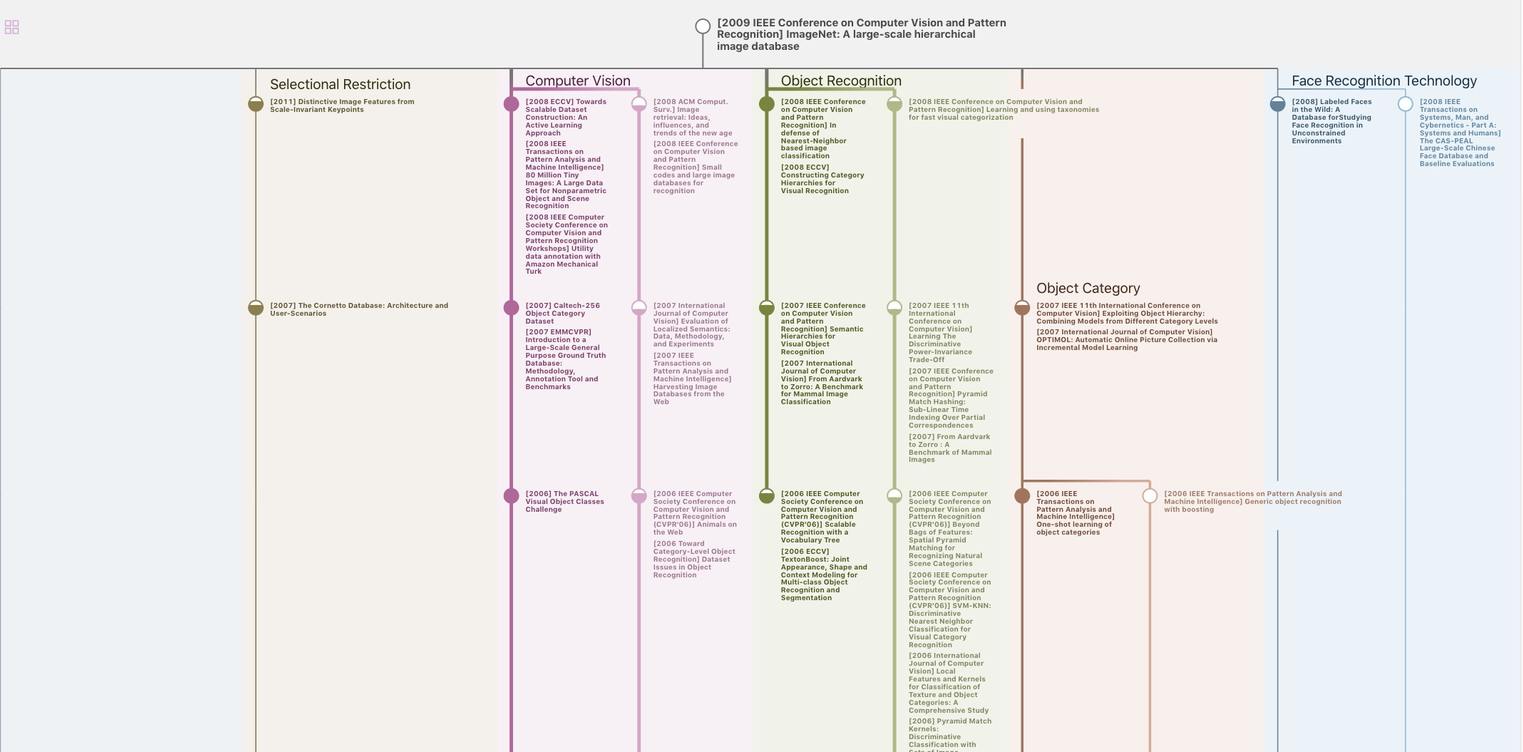
生成溯源树,研究论文发展脉络
Chat Paper
正在生成论文摘要